کد مقاله | کد نشریه | سال انتشار | مقاله انگلیسی | نسخه تمام متن |
---|---|---|---|---|
1162897 | 1490911 | 2016 | 12 صفحه PDF | دانلود رایگان |
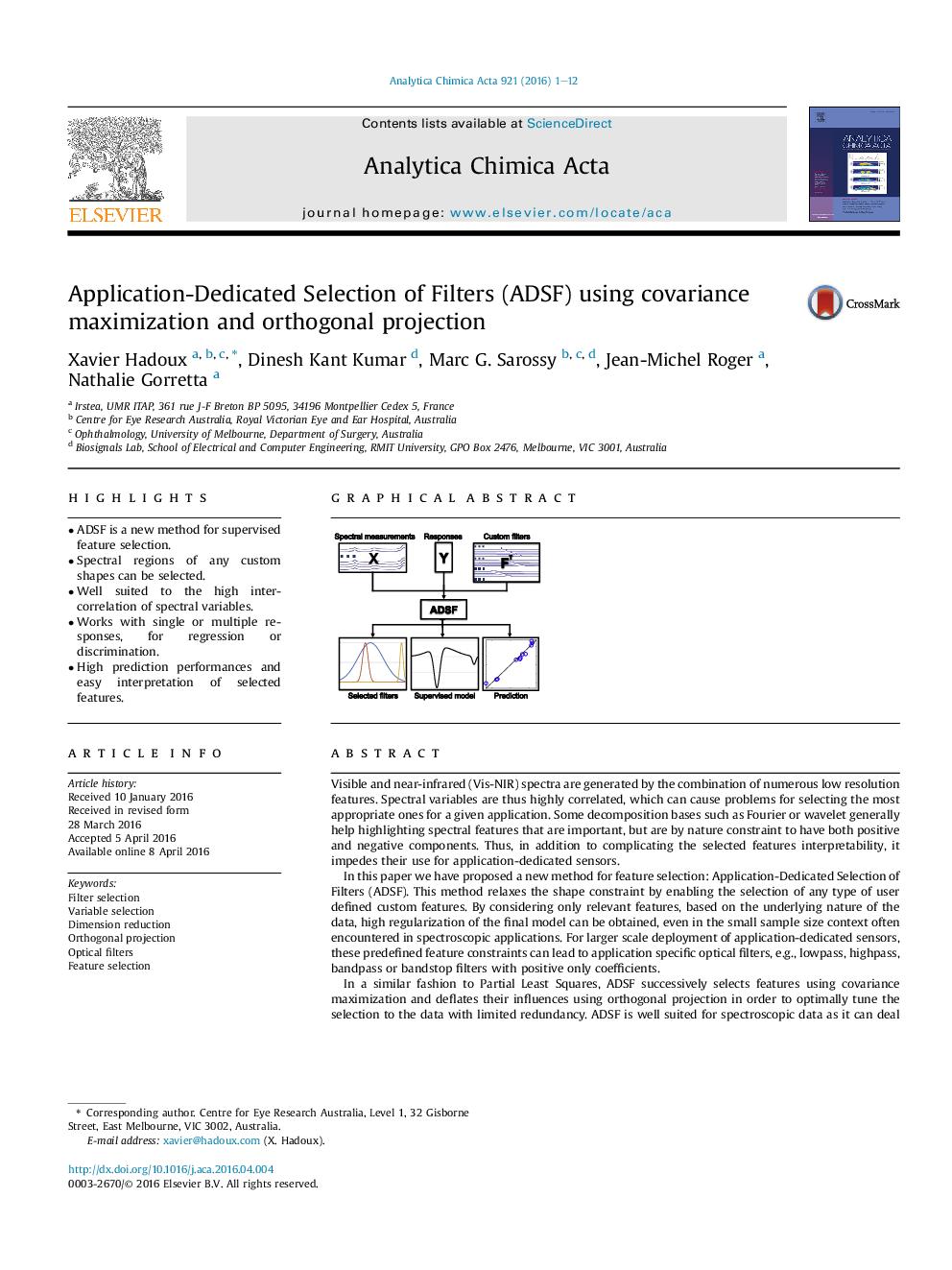
• ADSF is a new method for supervised feature selection.
• Spectral regions of any custom shapes can be selected.
• Well suited to the high inter-correlation of spectral variables.
• Works with single or multiple responses, for regression or discrimination.
• High prediction performances and easy interpretation of selected features.
Visible and near-infrared (Vis-NIR) spectra are generated by the combination of numerous low resolution features. Spectral variables are thus highly correlated, which can cause problems for selecting the most appropriate ones for a given application. Some decomposition bases such as Fourier or wavelet generally help highlighting spectral features that are important, but are by nature constraint to have both positive and negative components. Thus, in addition to complicating the selected features interpretability, it impedes their use for application-dedicated sensors.In this paper we have proposed a new method for feature selection: Application-Dedicated Selection of Filters (ADSF). This method relaxes the shape constraint by enabling the selection of any type of user defined custom features. By considering only relevant features, based on the underlying nature of the data, high regularization of the final model can be obtained, even in the small sample size context often encountered in spectroscopic applications. For larger scale deployment of application-dedicated sensors, these predefined feature constraints can lead to application specific optical filters, e.g., lowpass, highpass, bandpass or bandstop filters with positive only coefficients.In a similar fashion to Partial Least Squares, ADSF successively selects features using covariance maximization and deflates their influences using orthogonal projection in order to optimally tune the selection to the data with limited redundancy. ADSF is well suited for spectroscopic data as it can deal with large numbers of highly correlated variables in supervised learning, even with many correlated responses.
Figure optionsDownload as PowerPoint slide
Journal: Analytica Chimica Acta - Volume 921, 19 May 2016, Pages 1–12