کد مقاله | کد نشریه | سال انتشار | مقاله انگلیسی | نسخه تمام متن |
---|---|---|---|---|
1168819 | 1491161 | 2009 | 6 صفحه PDF | دانلود رایگان |
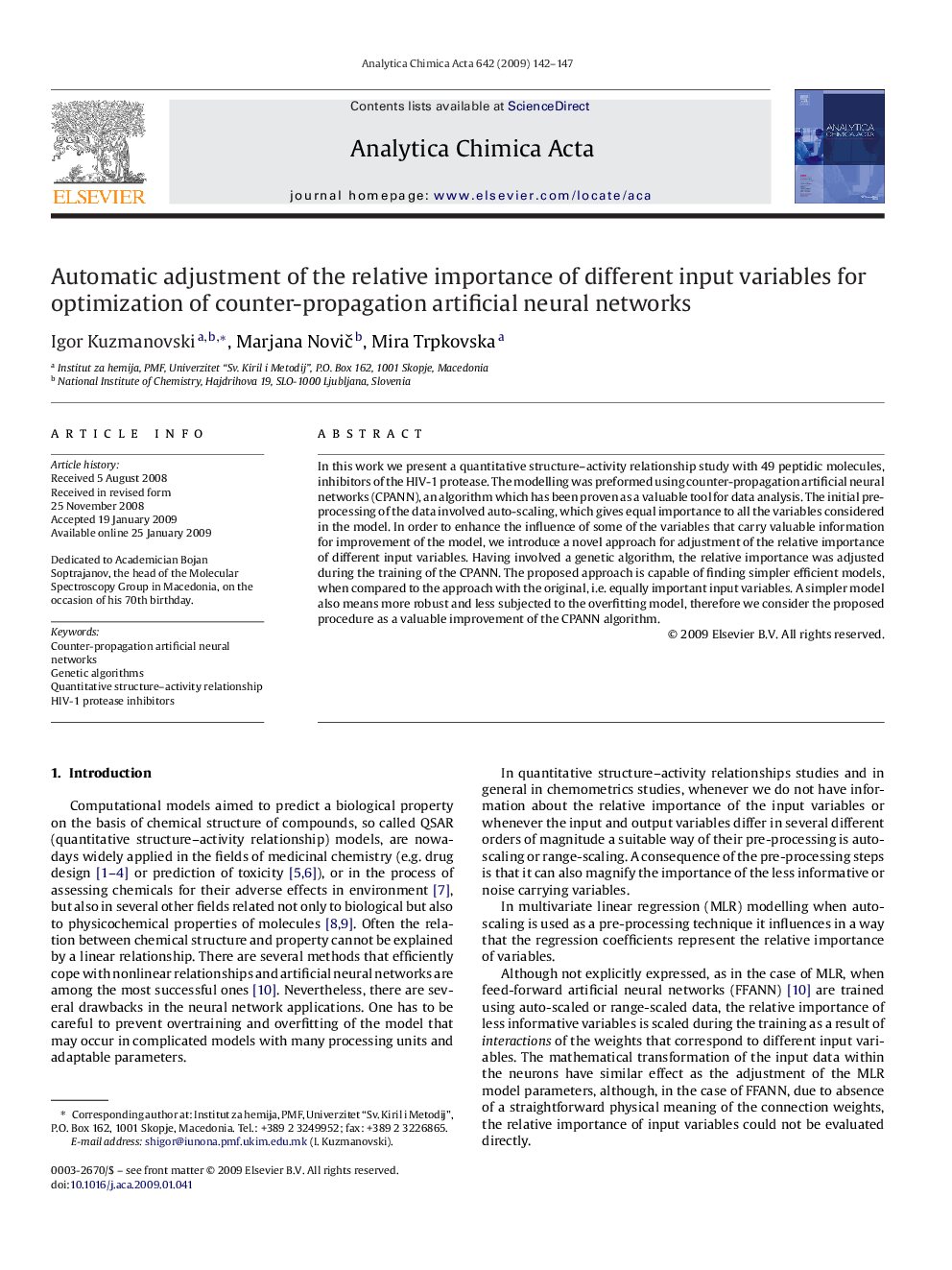
In this work we present a quantitative structure–activity relationship study with 49 peptidic molecules, inhibitors of the HIV-1 protease. The modelling was preformed using counter-propagation artificial neural networks (CPANN), an algorithm which has been proven as a valuable tool for data analysis. The initial pre-processing of the data involved auto-scaling, which gives equal importance to all the variables considered in the model. In order to enhance the influence of some of the variables that carry valuable information for improvement of the model, we introduce a novel approach for adjustment of the relative importance of different input variables. Having involved a genetic algorithm, the relative importance was adjusted during the training of the CPANN. The proposed approach is capable of finding simpler efficient models, when compared to the approach with the original, i.e. equally important input variables. A simpler model also means more robust and less subjected to the overfitting model, therefore we consider the proposed procedure as a valuable improvement of the CPANN algorithm.
Journal: Analytica Chimica Acta - Volume 642, Issues 1–2, 29 May 2009, Pages 142–147