کد مقاله | کد نشریه | سال انتشار | مقاله انگلیسی | نسخه تمام متن |
---|---|---|---|---|
15381 | 1408 | 2009 | 8 صفحه PDF | دانلود رایگان |
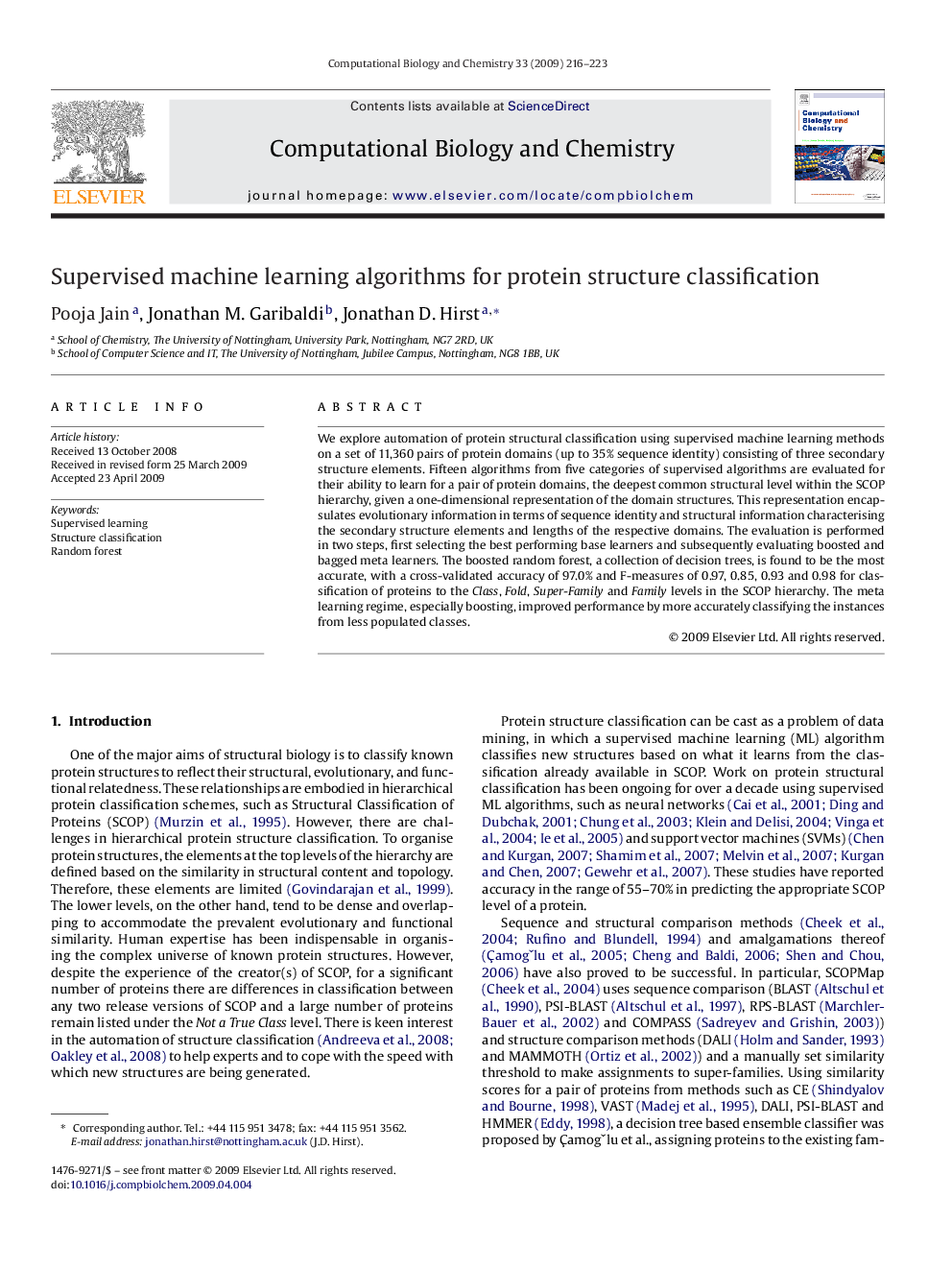
We explore automation of protein structural classification using supervised machine learning methods on a set of 11,360 pairs of protein domains (up to 35% sequence identity) consisting of three secondary structure elements. Fifteen algorithms from five categories of supervised algorithms are evaluated for their ability to learn for a pair of protein domains, the deepest common structural level within the SCOP hierarchy, given a one-dimensional representation of the domain structures. This representation encapsulates evolutionary information in terms of sequence identity and structural information characterising the secondary structure elements and lengths of the respective domains. The evaluation is performed in two steps, first selecting the best performing base learners and subsequently evaluating boosted and bagged meta learners. The boosted random forest, a collection of decision trees, is found to be the most accurate, with a cross-validated accuracy of 97.0% and F-measures of 0.97, 0.85, 0.93 and 0.98 for classification of proteins to the Class, Fold, Super-Family and Family levels in the SCOP hierarchy. The meta learning regime, especially boosting, improved performance by more accurately classifying the instances from less populated classes.
Journal: Computational Biology and Chemistry - Volume 33, Issue 3, June 2009, Pages 216–223