کد مقاله | کد نشریه | سال انتشار | مقاله انگلیسی | نسخه تمام متن |
---|---|---|---|---|
173530 | 458597 | 2008 | 9 صفحه PDF | دانلود رایگان |
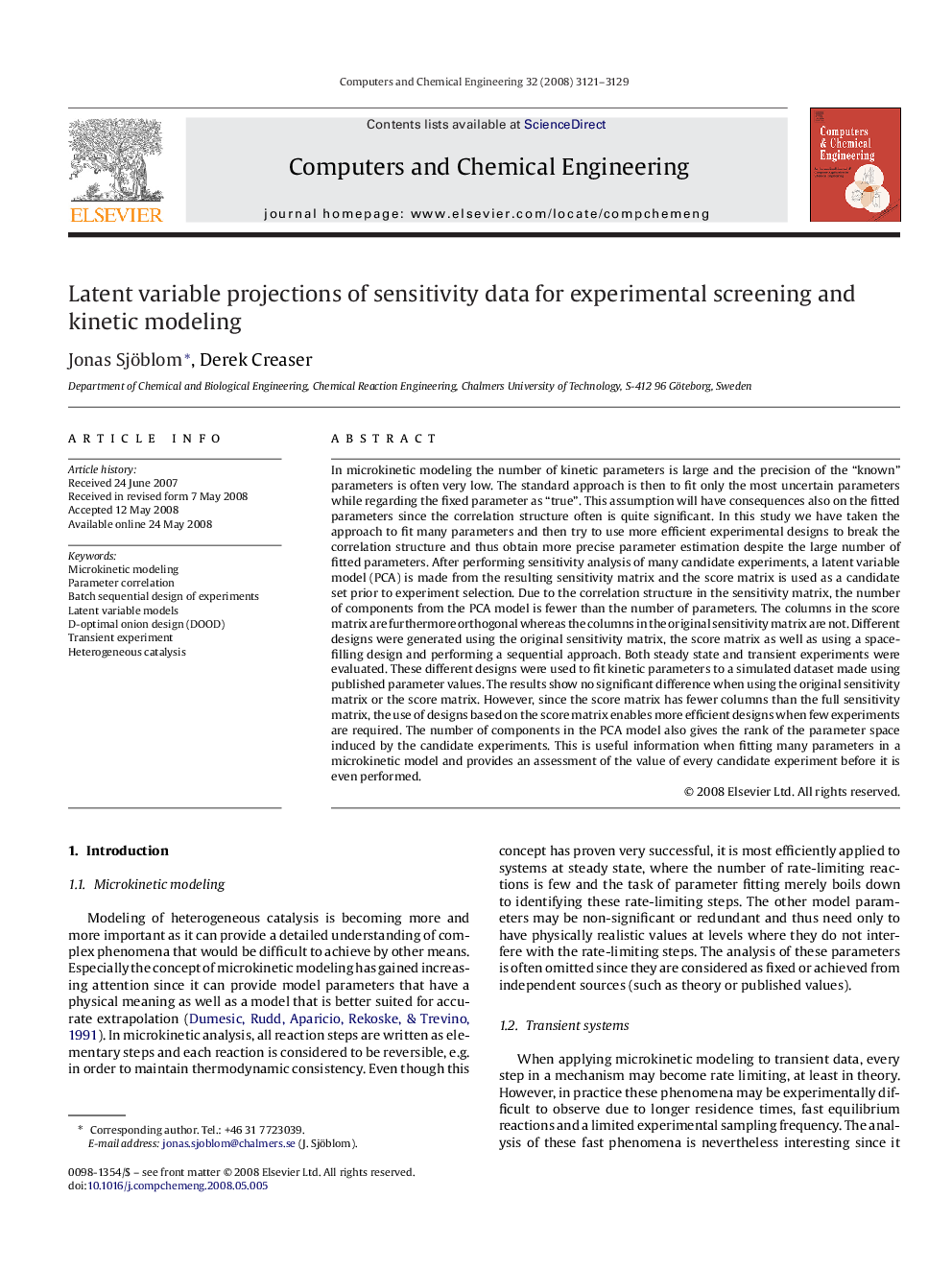
In microkinetic modeling the number of kinetic parameters is large and the precision of the “known” parameters is often very low. The standard approach is then to fit only the most uncertain parameters while regarding the fixed parameter as “true”. This assumption will have consequences also on the fitted parameters since the correlation structure often is quite significant. In this study we have taken the approach to fit many parameters and then try to use more efficient experimental designs to break the correlation structure and thus obtain more precise parameter estimation despite the large number of fitted parameters. After performing sensitivity analysis of many candidate experiments, a latent variable model (PCA) is made from the resulting sensitivity matrix and the score matrix is used as a candidate set prior to experiment selection. Due to the correlation structure in the sensitivity matrix, the number of components from the PCA model is fewer than the number of parameters. The columns in the score matrix are furthermore orthogonal whereas the columns in the original sensitivity matrix are not. Different designs were generated using the original sensitivity matrix, the score matrix as well as using a space-filling design and performing a sequential approach. Both steady state and transient experiments were evaluated. These different designs were used to fit kinetic parameters to a simulated dataset made using published parameter values. The results show no significant difference when using the original sensitivity matrix or the score matrix. However, since the score matrix has fewer columns than the full sensitivity matrix, the use of designs based on the score matrix enables more efficient designs when few experiments are required. The number of components in the PCA model also gives the rank of the parameter space induced by the candidate experiments. This is useful information when fitting many parameters in a microkinetic model and provides an assessment of the value of every candidate experiment before it is even performed.
Journal: Computers & Chemical Engineering - Volume 32, Issue 12, 22 December 2008, Pages 3121–3129