کد مقاله | کد نشریه | سال انتشار | مقاله انگلیسی | نسخه تمام متن |
---|---|---|---|---|
1758053 | 1523026 | 2013 | 12 صفحه PDF | دانلود رایگان |
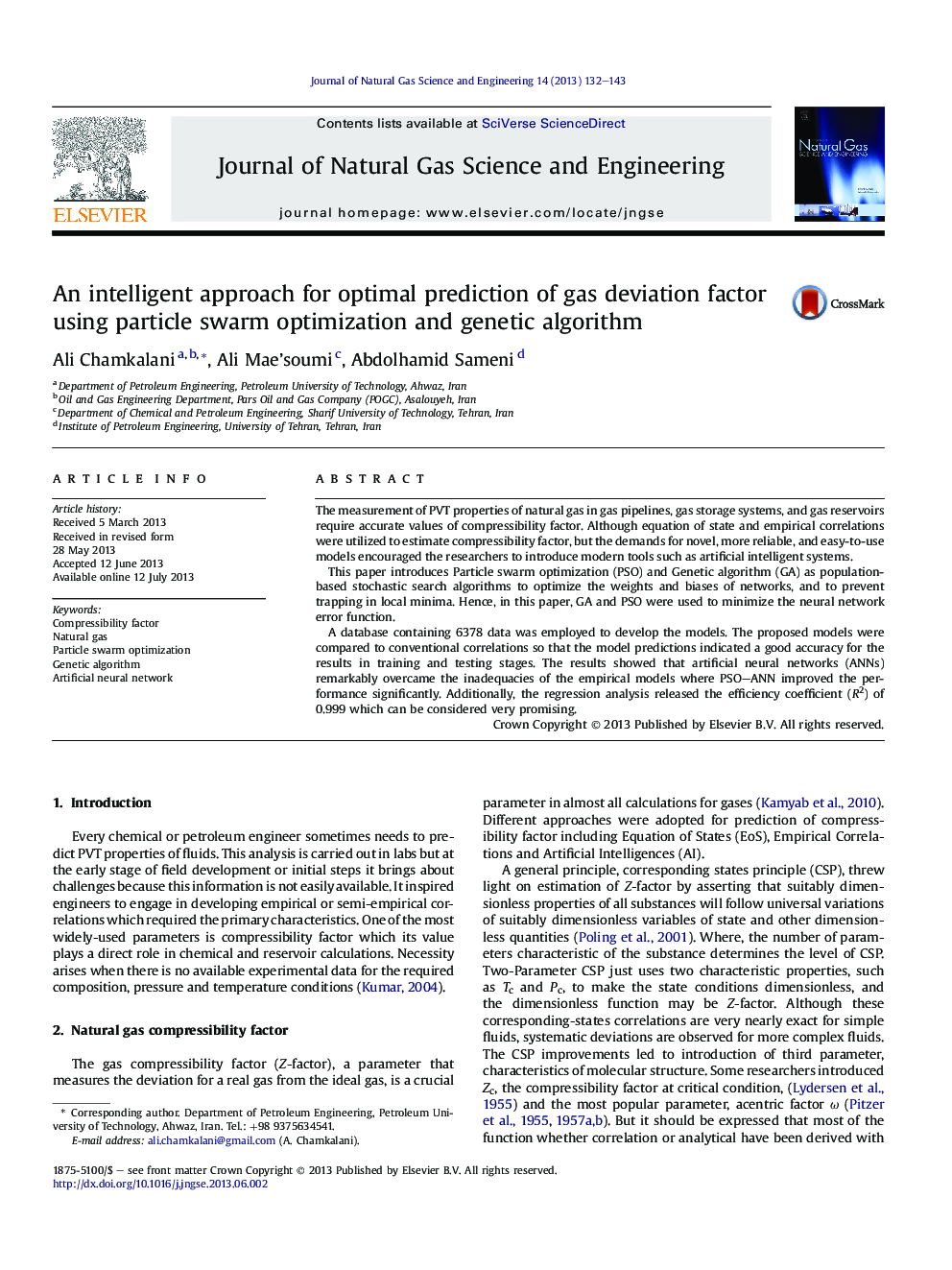
• Our work proposed optimized models for calculation of gas deviation factor.
• This study introduces two optimizations and a smart system for modelling.
• The optimization techniques are particle swarm optimization and genetic algorithm.
• PSO–ANN outperformed all empirical and intelligent models.
The measurement of PVT properties of natural gas in gas pipelines, gas storage systems, and gas reservoirs require accurate values of compressibility factor. Although equation of state and empirical correlations were utilized to estimate compressibility factor, but the demands for novel, more reliable, and easy-to-use models encouraged the researchers to introduce modern tools such as artificial intelligent systems.This paper introduces Particle swarm optimization (PSO) and Genetic algorithm (GA) as population-based stochastic search algorithms to optimize the weights and biases of networks, and to prevent trapping in local minima. Hence, in this paper, GA and PSO were used to minimize the neural network error function.A database containing 6378 data was employed to develop the models. The proposed models were compared to conventional correlations so that the model predictions indicated a good accuracy for the results in training and testing stages. The results showed that artificial neural networks (ANNs) remarkably overcame the inadequacies of the empirical models where PSO–ANN improved the performance significantly. Additionally, the regression analysis released the efficiency coefficient (R2) of 0.999 which can be considered very promising.
Journal: Journal of Natural Gas Science and Engineering - Volume 14, September 2013, Pages 132–143