کد مقاله | کد نشریه | سال انتشار | مقاله انگلیسی | نسخه تمام متن |
---|---|---|---|---|
312495 | 534221 | 2012 | 15 صفحه PDF | دانلود رایگان |
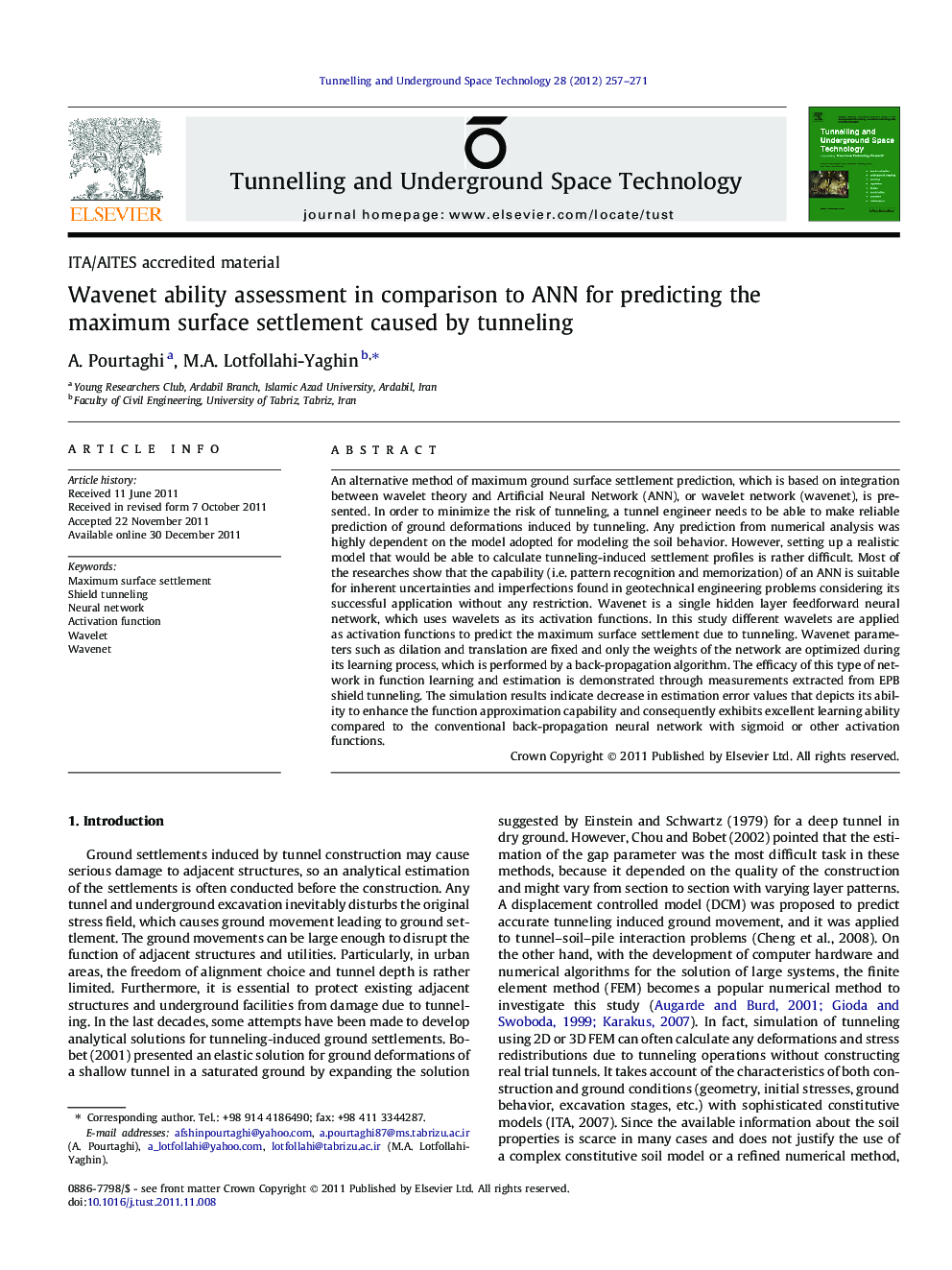
An alternative method of maximum ground surface settlement prediction, which is based on integration between wavelet theory and Artificial Neural Network (ANN), or wavelet network (wavenet), is presented. In order to minimize the risk of tunneling, a tunnel engineer needs to be able to make reliable prediction of ground deformations induced by tunneling. Any prediction from numerical analysis was highly dependent on the model adopted for modeling the soil behavior. However, setting up a realistic model that would be able to calculate tunneling-induced settlement profiles is rather difficult. Most of the researches show that the capability (i.e. pattern recognition and memorization) of an ANN is suitable for inherent uncertainties and imperfections found in geotechnical engineering problems considering its successful application without any restriction. Wavenet is a single hidden layer feedforward neural network, which uses wavelets as its activation functions. In this study different wavelets are applied as activation functions to predict the maximum surface settlement due to tunneling. Wavenet parameters such as dilation and translation are fixed and only the weights of the network are optimized during its learning process, which is performed by a back-propagation algorithm. The efficacy of this type of network in function learning and estimation is demonstrated through measurements extracted from EPB shield tunneling. The simulation results indicate decrease in estimation error values that depicts its ability to enhance the function approximation capability and consequently exhibits excellent learning ability compared to the conventional back-propagation neural network with sigmoid or other activation functions.
► The potential of applying ANN and its adaptive wavelets has shown in this analysis.
► Using wavelets as ANN transfer functions can enhance the network efficiency.
► Wavenet convergence rate and learning ability is much faster than ANN approach.
► Wavenet has universal approximation properties and is a capable function estimator.
► Morlet wavenet is the best network in terms of performance in this case study.
Journal: Tunnelling and Underground Space Technology - Volume 28, March 2012, Pages 257–271