کد مقاله | کد نشریه | سال انتشار | مقاله انگلیسی | نسخه تمام متن |
---|---|---|---|---|
432741 | 689058 | 2013 | 13 صفحه PDF | دانلود رایگان |
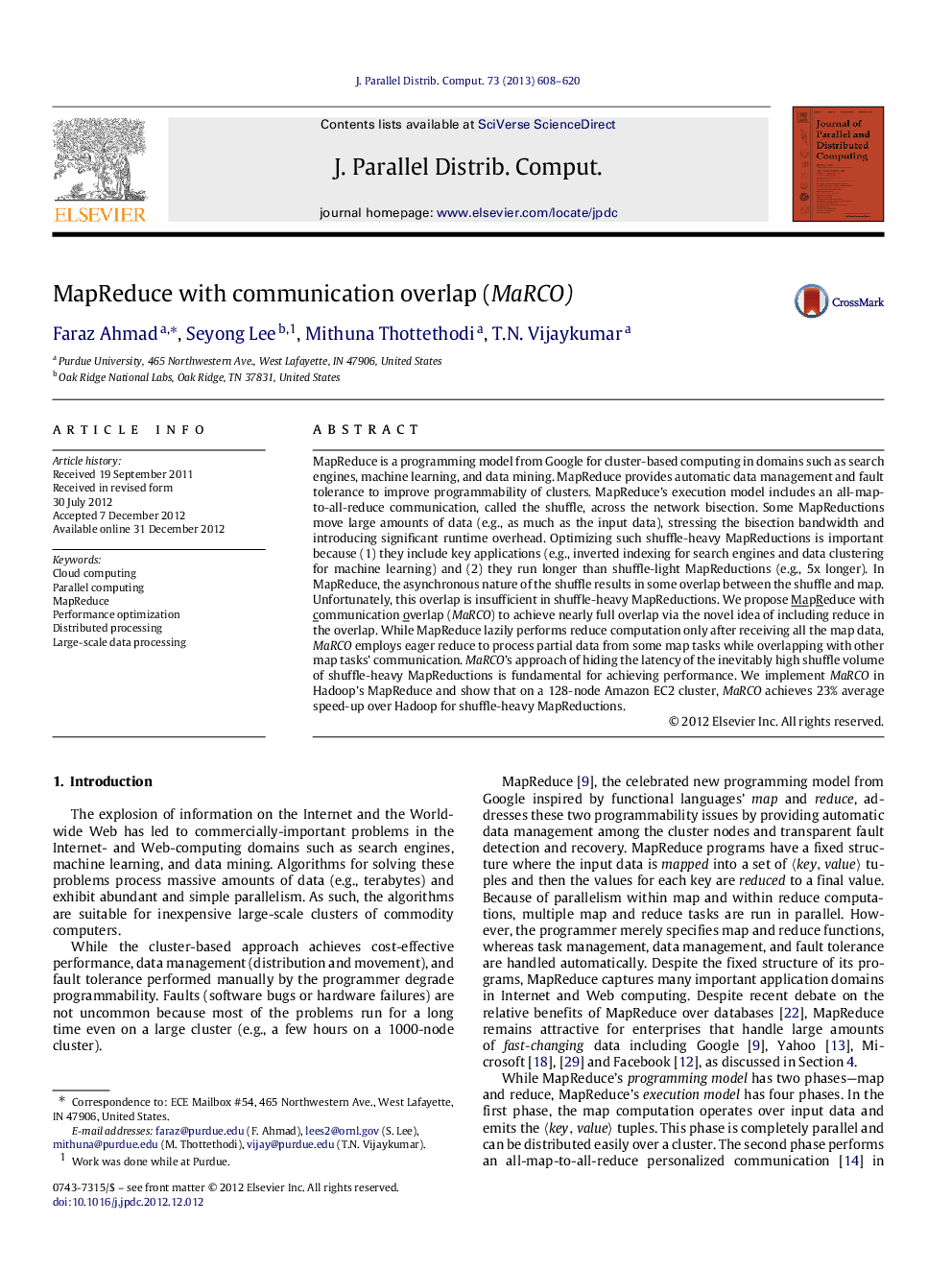
MapReduce is a programming model from Google for cluster-based computing in domains such as search engines, machine learning, and data mining. MapReduce provides automatic data management and fault tolerance to improve programmability of clusters. MapReduce’s execution model includes an all-map-to-all-reduce communication, called the shuffle, across the network bisection. Some MapReductions move large amounts of data (e.g., as much as the input data), stressing the bisection bandwidth and introducing significant runtime overhead. Optimizing such shuffle-heavy MapReductions is important because (1) they include key applications (e.g., inverted indexing for search engines and data clustering for machine learning) and (2) they run longer than shuffle-light MapReductions (e.g., 5x longer). In MapReduce, the asynchronous nature of the shuffle results in some overlap between the shuffle and map. Unfortunately, this overlap is insufficient in shuffle-heavy MapReductions. We propose MapReduce with communication overlap (MaRCO) to achieve nearly full overlap via the novel idea of including reduce in the overlap. While MapReduce lazily performs reduce computation only after receiving all the map data, MaRCO employs eager reduce to process partial data from some map tasks while overlapping with other map tasks’ communication. MaRCO’s approach of hiding the latency of the inevitably high shuffle volume of shuffle-heavy MapReductions is fundamental for achieving performance. We implement MaRCO in Hadoop’s MapReduce and show that on a 128-node Amazon EC2 cluster, MaRCO achieves 23% average speed-up over Hadoop for shuffle-heavy MapReductions.
► Identifying the problem of exposed shuffle in shuffle-heavy MapReductions.
► Addressing the problem via the novel idea of fully overlapping the shuffle with the reduce.
► Employing control mechanisms to avoid overheads due to this overlap.
► Introducing a benchmark suite (MapReductions) covering most of the MapReduce application domains.
► Significantly speeding up shuffle-heavy MapReductions in a complete MapReduce implementation running on a real-world cluster.
Journal: Journal of Parallel and Distributed Computing - Volume 73, Issue 5, May 2013, Pages 608–620