کد مقاله | کد نشریه | سال انتشار | مقاله انگلیسی | نسخه تمام متن |
---|---|---|---|---|
4459109 | 1621273 | 2012 | 13 صفحه PDF | دانلود رایگان |
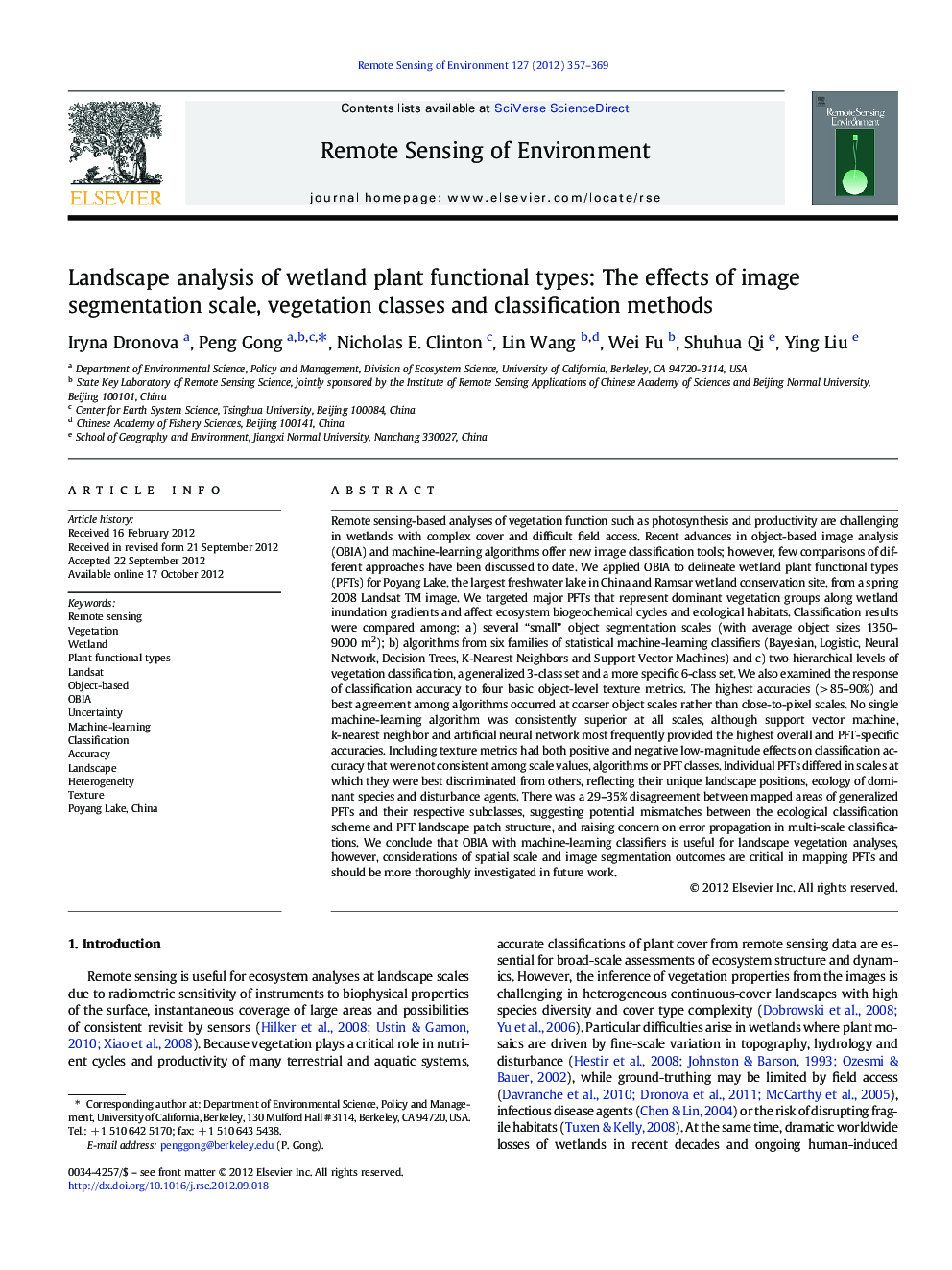
Remote sensing-based analyses of vegetation function such as photosynthesis and productivity are challenging in wetlands with complex cover and difficult field access. Recent advances in object-based image analysis (OBIA) and machine-learning algorithms offer new image classification tools; however, few comparisons of different approaches have been discussed to date. We applied OBIA to delineate wetland plant functional types (PFTs) for Poyang Lake, the largest freshwater lake in China and Ramsar wetland conservation site, from a spring 2008 Landsat TM image. We targeted major PFTs that represent dominant vegetation groups along wetland inundation gradients and affect ecosystem biogeochemical cycles and ecological habitats. Classification results were compared among: a) several “small” object segmentation scales (with average object sizes 1350–9000 m2); b) algorithms from six families of statistical machine-learning classifiers (Bayesian, Logistic, Neural Network, Decision Trees, K-Nearest Neighbors and Support Vector Machines) and c) two hierarchical levels of vegetation classification, a generalized 3-class set and a more specific 6-class set. We also examined the response of classification accuracy to four basic object-level texture metrics. The highest accuracies (> 85–90%) and best agreement among algorithms occurred at coarser object scales rather than close-to-pixel scales. No single machine-learning algorithm was consistently superior at all scales, although support vector machine, k-nearest neighbor and artificial neural network most frequently provided the highest overall and PFT-specific accuracies. Including texture metrics had both positive and negative low-magnitude effects on classification accuracy that were not consistent among scale values, algorithms or PFT classes. Individual PFTs differed in scales at which they were best discriminated from others, reflecting their unique landscape positions, ecology of dominant species and disturbance agents. There was a 29–35% disagreement between mapped areas of generalized PFTs and their respective subclasses, suggesting potential mismatches between the ecological classification scheme and PFT landscape patch structure, and raising concern on error propagation in multi-scale classifications. We conclude that OBIA with machine-learning classifiers is useful for landscape vegetation analyses, however, considerations of spatial scale and image segmentation outcomes are critical in mapping PFTs and should be more thoroughly investigated in future work.
► We used object-based image analysis to map wetland plant functional types (PFTs).
► Highest classification accuracy occurred at object scales coarser than pixel level.
► Classes differed in segmentation scales which maximized their individual accuracy.
► Success of several machine-learning classifiers varied among PFTs and object scales.
► Mismatches among mapped PFT levels suggest error propagation in multi-scale OBIA.
Journal: Remote Sensing of Environment - Volume 127, December 2012, Pages 357–369