کد مقاله | کد نشریه | سال انتشار | مقاله انگلیسی | نسخه تمام متن |
---|---|---|---|---|
4460189 | 1621320 | 2009 | 9 صفحه PDF | دانلود رایگان |
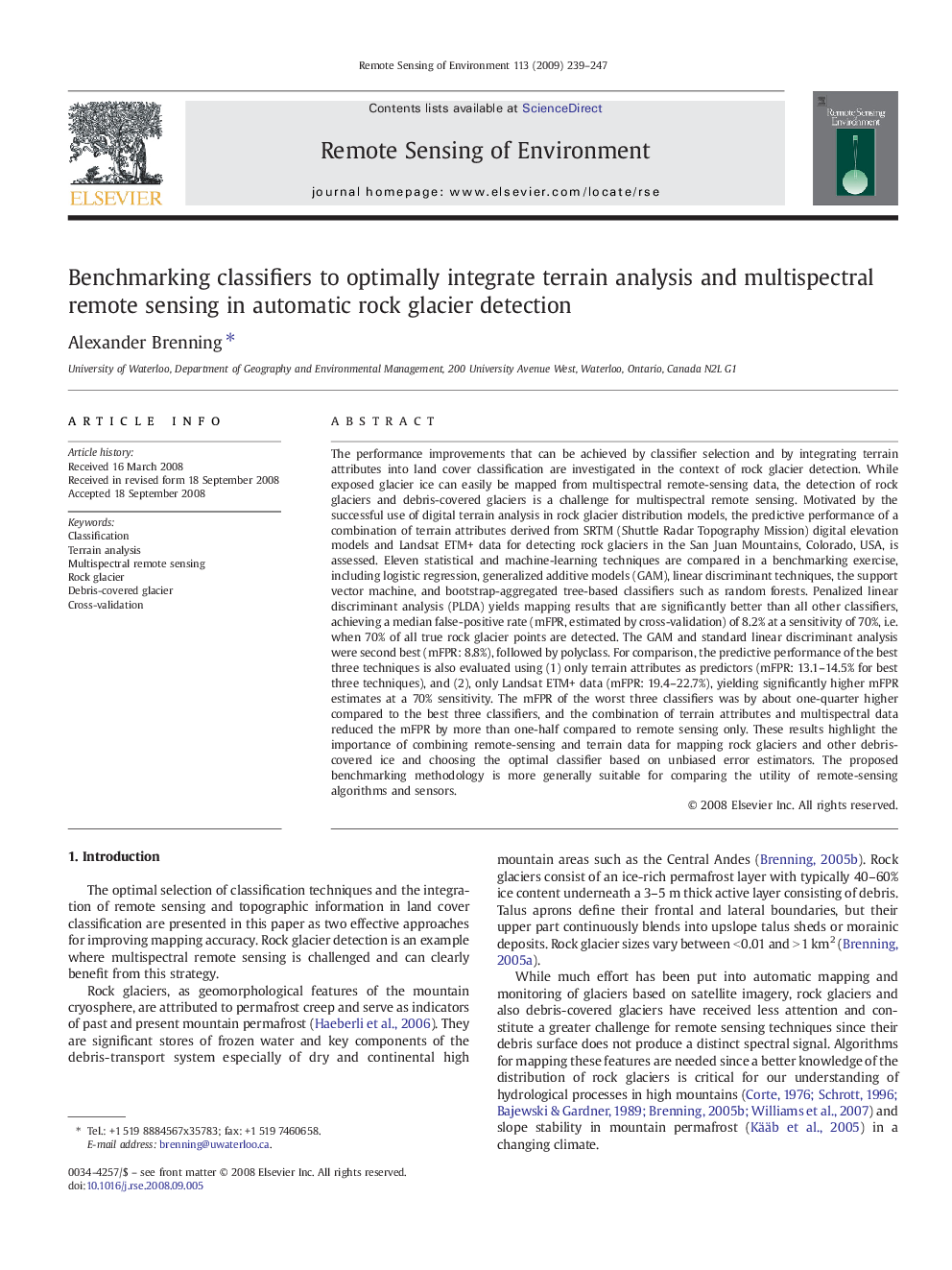
The performance improvements that can be achieved by classifier selection and by integrating terrain attributes into land cover classification are investigated in the context of rock glacier detection. While exposed glacier ice can easily be mapped from multispectral remote-sensing data, the detection of rock glaciers and debris-covered glaciers is a challenge for multispectral remote sensing. Motivated by the successful use of digital terrain analysis in rock glacier distribution models, the predictive performance of a combination of terrain attributes derived from SRTM (Shuttle Radar Topography Mission) digital elevation models and Landsat ETM+ data for detecting rock glaciers in the San Juan Mountains, Colorado, USA, is assessed. Eleven statistical and machine-learning techniques are compared in a benchmarking exercise, including logistic regression, generalized additive models (GAM), linear discriminant techniques, the support vector machine, and bootstrap-aggregated tree-based classifiers such as random forests. Penalized linear discriminant analysis (PLDA) yields mapping results that are significantly better than all other classifiers, achieving a median false-positive rate (mFPR, estimated by cross-validation) of 8.2% at a sensitivity of 70%, i.e. when 70% of all true rock glacier points are detected. The GAM and standard linear discriminant analysis were second best (mFPR: 8.8%), followed by polyclass. For comparison, the predictive performance of the best three techniques is also evaluated using (1) only terrain attributes as predictors (mFPR: 13.1–14.5% for best three techniques), and (2), only Landsat ETM+ data (mFPR: 19.4–22.7%), yielding significantly higher mFPR estimates at a 70% sensitivity. The mFPR of the worst three classifiers was by about one-quarter higher compared to the best three classifiers, and the combination of terrain attributes and multispectral data reduced the mFPR by more than one-half compared to remote sensing only. These results highlight the importance of combining remote-sensing and terrain data for mapping rock glaciers and other debris-covered ice and choosing the optimal classifier based on unbiased error estimators. The proposed benchmarking methodology is more generally suitable for comparing the utility of remote-sensing algorithms and sensors.
Journal: Remote Sensing of Environment - Volume 113, Issue 1, 15 January 2009, Pages 239–247