کد مقاله | کد نشریه | سال انتشار | مقاله انگلیسی | نسخه تمام متن |
---|---|---|---|---|
4577977 | 1630036 | 2011 | 11 صفحه PDF | دانلود رایگان |
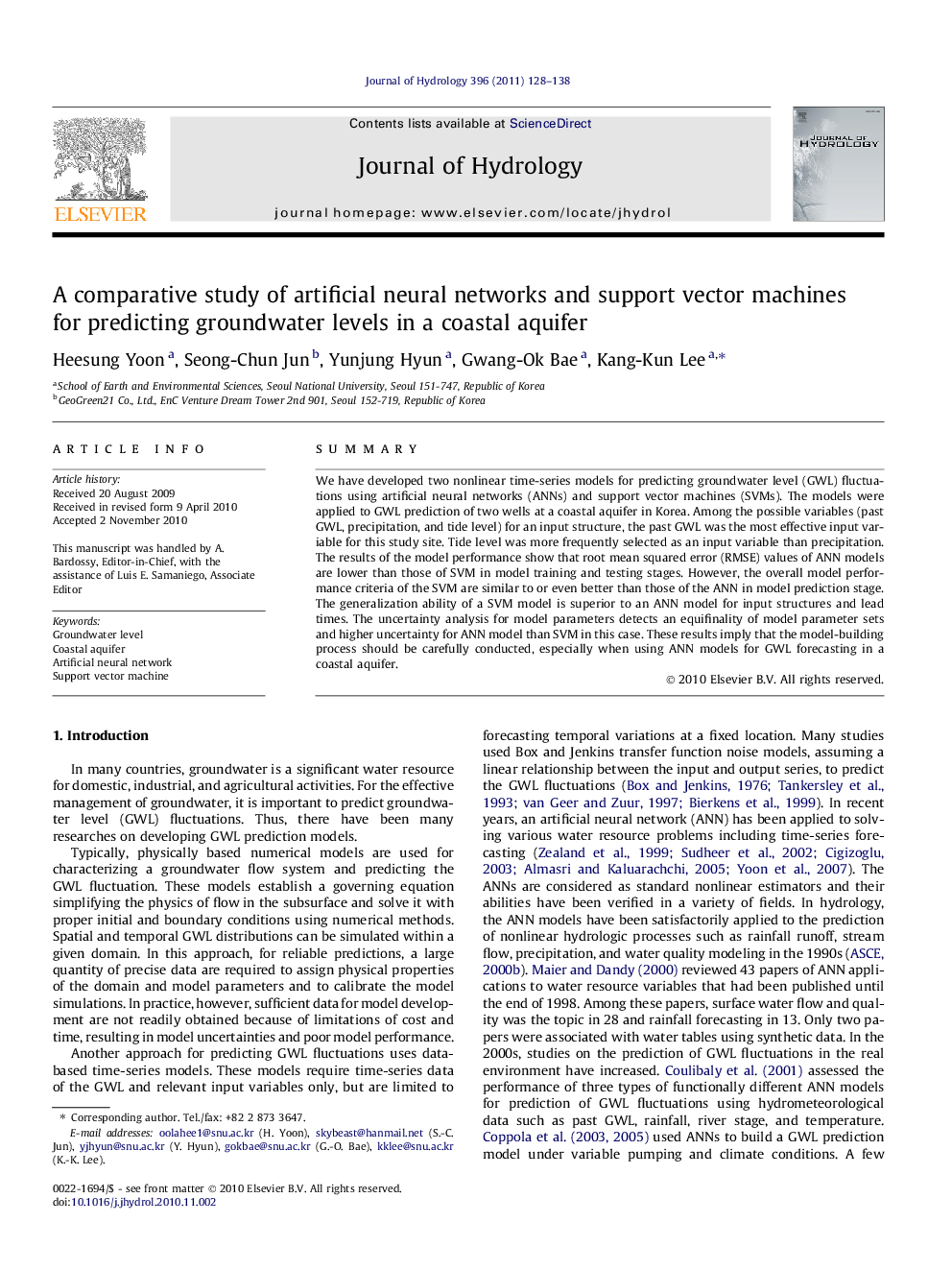
SummaryWe have developed two nonlinear time-series models for predicting groundwater level (GWL) fluctuations using artificial neural networks (ANNs) and support vector machines (SVMs). The models were applied to GWL prediction of two wells at a coastal aquifer in Korea. Among the possible variables (past GWL, precipitation, and tide level) for an input structure, the past GWL was the most effective input variable for this study site. Tide level was more frequently selected as an input variable than precipitation. The results of the model performance show that root mean squared error (RMSE) values of ANN models are lower than those of SVM in model training and testing stages. However, the overall model performance criteria of the SVM are similar to or even better than those of the ANN in model prediction stage. The generalization ability of a SVM model is superior to an ANN model for input structures and lead times. The uncertainty analysis for model parameters detects an equifinality of model parameter sets and higher uncertainty for ANN model than SVM in this case. These results imply that the model-building process should be carefully conducted, especially when using ANN models for GWL forecasting in a coastal aquifer.
Research highlights
► We compare ANN and SVM for groundwater level fluctuations in a coastal aquifer.
► The past groundwater level is the most effective input variable.
► Tide level is more frequently selected as an input variable than precipitation.
► SVM is superior to ANN for generalization ability and uncertainty analysis.
Journal: Journal of Hydrology - Volume 396, Issues 1–2, 5 January 2011, Pages 128–138