کد مقاله | کد نشریه | سال انتشار | مقاله انگلیسی | نسخه تمام متن |
---|---|---|---|---|
4578609 | 1630072 | 2009 | 15 صفحه PDF | دانلود رایگان |
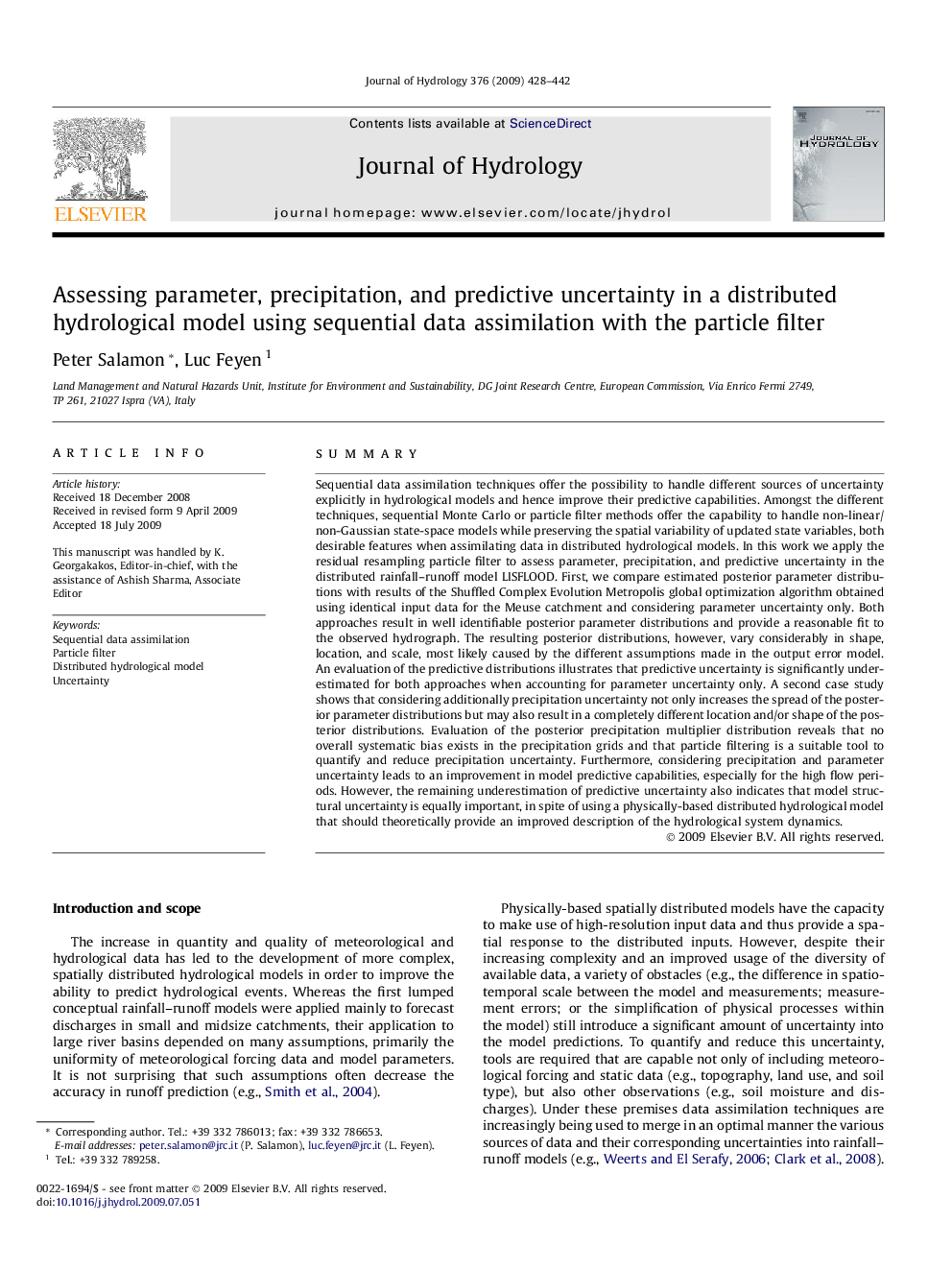
SummarySequential data assimilation techniques offer the possibility to handle different sources of uncertainty explicitly in hydrological models and hence improve their predictive capabilities. Amongst the different techniques, sequential Monte Carlo or particle filter methods offer the capability to handle non-linear/non-Gaussian state-space models while preserving the spatial variability of updated state variables, both desirable features when assimilating data in distributed hydrological models. In this work we apply the residual resampling particle filter to assess parameter, precipitation, and predictive uncertainty in the distributed rainfall–runoff model LISFLOOD. First, we compare estimated posterior parameter distributions with results of the Shuffled Complex Evolution Metropolis global optimization algorithm obtained using identical input data for the Meuse catchment and considering parameter uncertainty only. Both approaches result in well identifiable posterior parameter distributions and provide a reasonable fit to the observed hydrograph. The resulting posterior distributions, however, vary considerably in shape, location, and scale, most likely caused by the different assumptions made in the output error model. An evaluation of the predictive distributions illustrates that predictive uncertainty is significantly underestimated for both approaches when accounting for parameter uncertainty only. A second case study shows that considering additionally precipitation uncertainty not only increases the spread of the posterior parameter distributions but may also result in a completely different location and/or shape of the posterior distributions. Evaluation of the posterior precipitation multiplier distribution reveals that no overall systematic bias exists in the precipitation grids and that particle filtering is a suitable tool to quantify and reduce precipitation uncertainty. Furthermore, considering precipitation and parameter uncertainty leads to an improvement in model predictive capabilities, especially for the high flow periods. However, the remaining underestimation of predictive uncertainty also indicates that model structural uncertainty is equally important, in spite of using a physically-based distributed hydrological model that should theoretically provide an improved description of the hydrological system dynamics.
Journal: Journal of Hydrology - Volume 376, Issues 3–4, 15 October 2009, Pages 428–442