کد مقاله | کد نشریه | سال انتشار | مقاله انگلیسی | نسخه تمام متن |
---|---|---|---|---|
5131150 | 1490878 | 2017 | 10 صفحه PDF | دانلود رایگان |
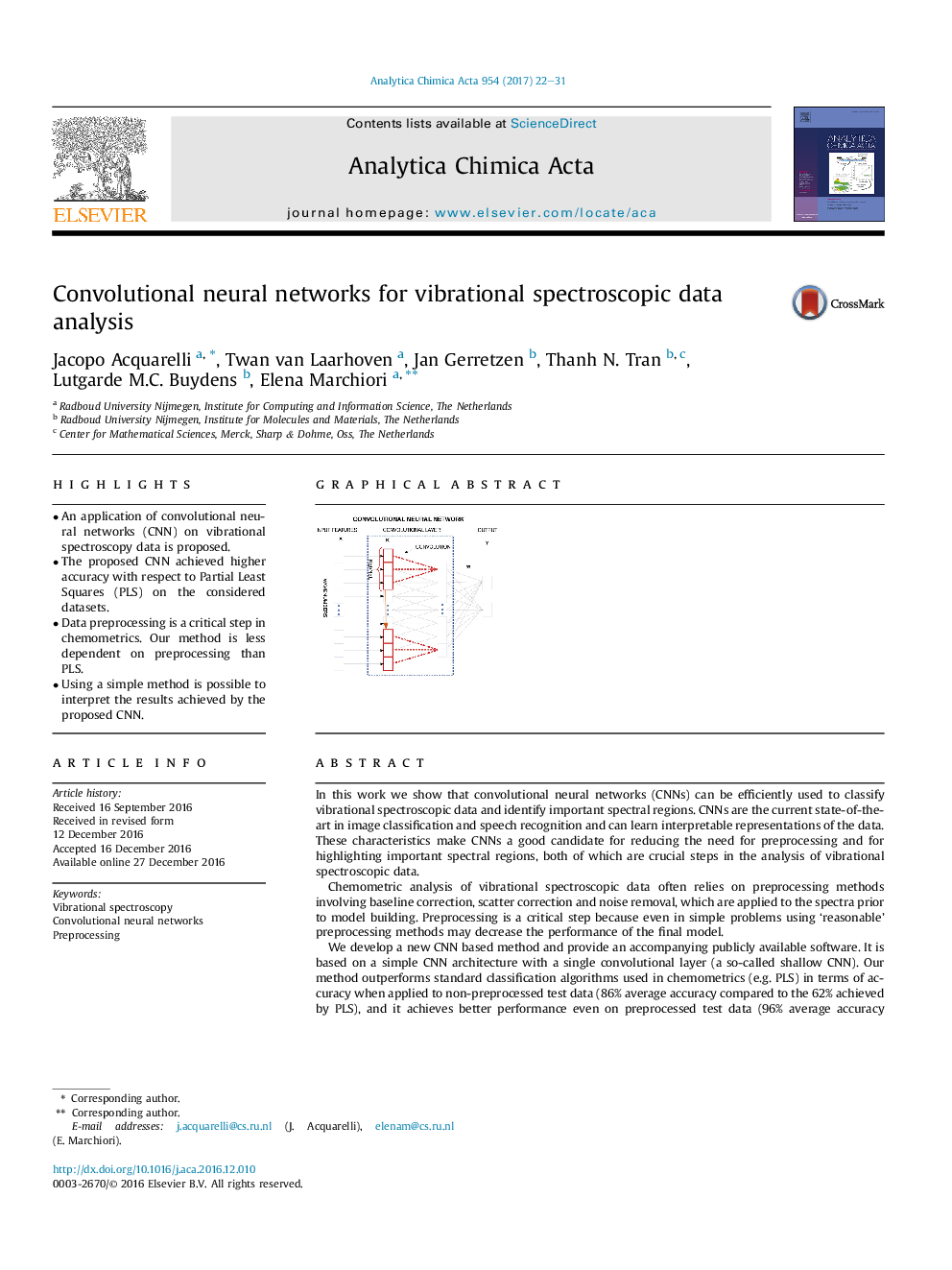
- An application of convolutional neural networks (CNN) on vibrational spectroscopy data is proposed.
- The proposed CNN achieved higher accuracy with respect to Partial Least Squares (PLS) on the considered datasets.
- Data preprocessing is a critical step in chemometrics. Our method is less dependent on preprocessing than PLS.
- Using a simple method is possible to interpret the results achieved by the proposed CNN.
In this work we show that convolutional neural networks (CNNs) can be efficiently used to classify vibrational spectroscopic data and identify important spectral regions. CNNs are the current state-of-the-art in image classification and speech recognition and can learn interpretable representations of the data. These characteristics make CNNs a good candidate for reducing the need for preprocessing and for highlighting important spectral regions, both of which are crucial steps in the analysis of vibrational spectroscopic data.Chemometric analysis of vibrational spectroscopic data often relies on preprocessing methods involving baseline correction, scatter correction and noise removal, which are applied to the spectra prior to model building. Preprocessing is a critical step because even in simple problems using 'reasonable' preprocessing methods may decrease the performance of the final model.We develop a new CNN based method and provide an accompanying publicly available software. It is based on a simple CNN architecture with a single convolutional layer (a so-called shallow CNN). Our method outperforms standard classification algorithms used in chemometrics (e.g. PLS) in terms of accuracy when applied to non-preprocessed test data (86% average accuracy compared to the 62% achieved by PLS), and it achieves better performance even on preprocessed test data (96% average accuracy compared to the 89% achieved by PLS). For interpretability purposes, our method includes a procedure for finding important spectral regions, thereby facilitating qualitative interpretation of results.
196
Journal: Analytica Chimica Acta - Volume 954, 15 February 2017, Pages 22-31