کد مقاله | کد نشریه | سال انتشار | مقاله انگلیسی | نسخه تمام متن |
---|---|---|---|---|
5132172 | 1491512 | 2017 | 6 صفحه PDF | دانلود رایگان |
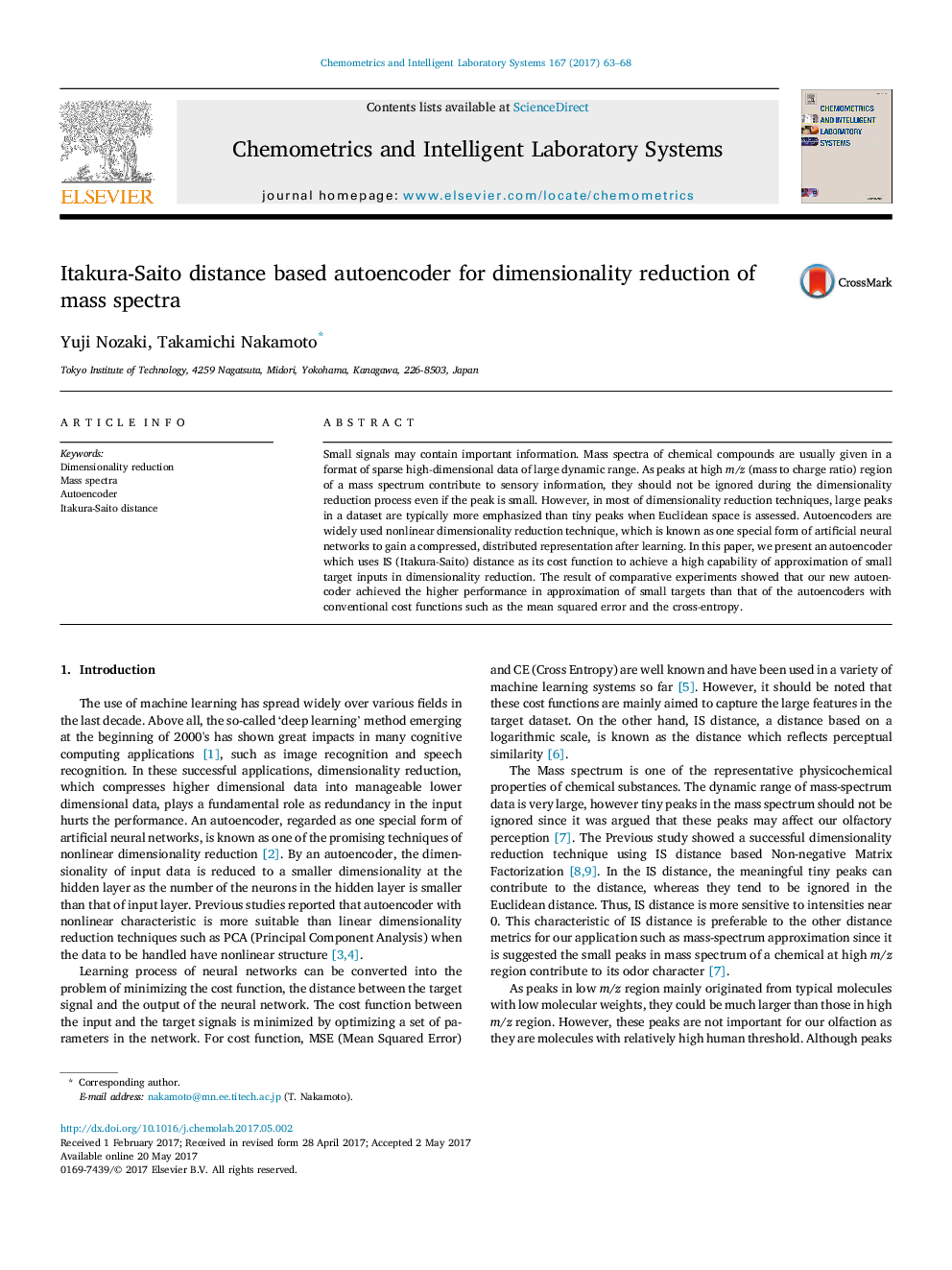
- An autoencoder using Itakura-Saito distance as its cost function was proposed.
- It was experimentally verified that accuracy of reproduction of small values can be improved by proposed autoencoder.
- The IS distance based autoencoder resolves gradient vanishing problem observed when conventional cost functions were used.
Small signals may contain important information. Mass spectra of chemical compounds are usually given in a format of sparse high-dimensional data of large dynamic range. As peaks at high m/z (mass to charge ratio) region of a mass spectrum contribute to sensory information, they should not be ignored during the dimensionality reduction process even if the peak is small. However, in most of dimensionality reduction techniques, large peaks in a dataset are typically more emphasized than tiny peaks when Euclidean space is assessed. Autoencoders are widely used nonlinear dimensionality reduction technique, which is known as one special form of artificial neural networks to gain a compressed, distributed representation after learning. In this paper, we present an autoencoder which uses IS (Itakura-Saito) distance as its cost function to achieve a high capability of approximation of small target inputs in dimensionality reduction. The result of comparative experiments showed that our new autoencoder achieved the higher performance in approximation of small targets than that of the autoencoders with conventional cost functions such as the mean squared error and the cross-entropy.
Journal: Chemometrics and Intelligent Laboratory Systems - Volume 167, 15 August 2017, Pages 63-68