کد مقاله | کد نشریه | سال انتشار | مقاله انگلیسی | نسخه تمام متن |
---|---|---|---|---|
5132189 | 1491512 | 2017 | 12 صفحه PDF | دانلود رایگان |
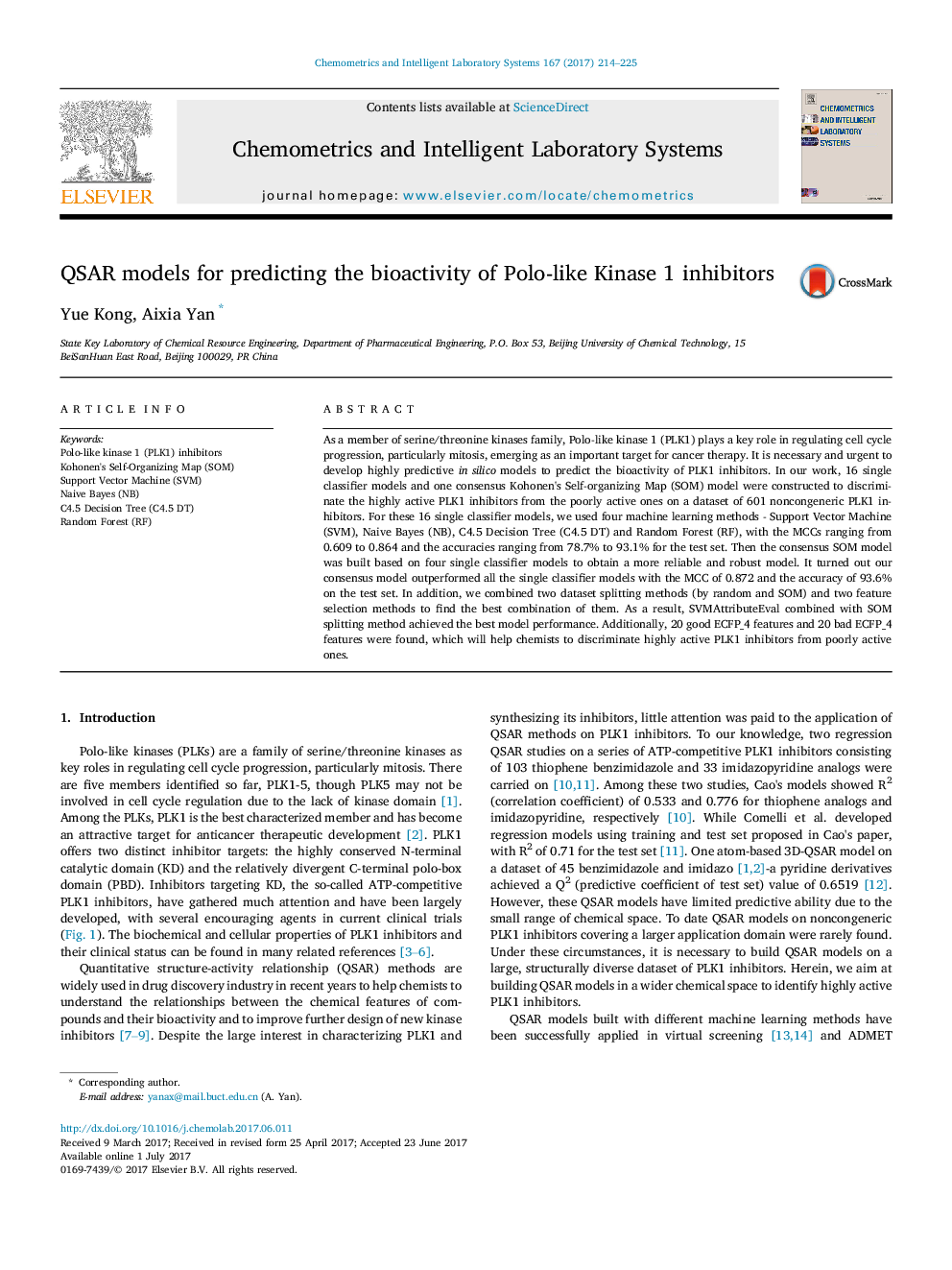
- Developed in silico models to predict the bioactivity of Polo-like kinase 1 (PLK1) inhibitors.
- Constructed 16 single classifier models and one consensus Kohonen's Self-organizing Map (SOM) model.
- MCCs range from 0.609 to 0.864 and accuracies range from 78.7% to 93.1% for test set of 16 single classifier models.
- Consensus SOM model based on single classifiers obtained a more reliable model with MCC of 0.872 for test set.
- Feature selected by SVMAttributeEval and split by SOM achieved the best model performance.
As a member of serine/threonine kinases family, Polo-like kinase 1 (PLK1) plays a key role in regulating cell cycle progression, particularly mitosis, emerging as an important target for cancer therapy. It is necessary and urgent to develop highly predictive in silico models to predict the bioactivity of PLK1 inhibitors. In our work, 16 single classifier models and one consensus Kohonen's Self-organizing Map (SOM) model were constructed to discriminate the highly active PLK1 inhibitors from the poorly active ones on a dataset of 601 noncongeneric PLK1 inhibitors. For these 16 single classifier models, we used four machine learning methods - Support Vector Machine (SVM), Naive Bayes (NB), C4.5 Decision Tree (C4.5 DT) and Random Forest (RF), with the MCCs ranging from 0.609 to 0.864 and the accuracies ranging from 78.7% to 93.1% for the test set. Then the consensus SOM model was built based on four single classifier models to obtain a more reliable and robust model. It turned out our consensus model outperformed all the single classifier models with the MCC of 0.872 and the accuracy of 93.6% on the test set. In addition, we combined two dataset splitting methods (by random and SOM) and two feature selection methods to find the best combination of them. As a result, SVMAttributeEval combined with SOM splitting method achieved the best model performance. Additionally, 20 good ECFP_4 features and 20 bad ECFP_4 features were found, which will help chemists to discriminate highly active PLK1 inhibitors from poorly active ones.
Journal: Chemometrics and Intelligent Laboratory Systems - Volume 167, 15 August 2017, Pages 214-225