کد مقاله | کد نشریه | سال انتشار | مقاله انگلیسی | نسخه تمام متن |
---|---|---|---|---|
5132237 | 1491514 | 2017 | 9 صفحه PDF | دانلود رایگان |
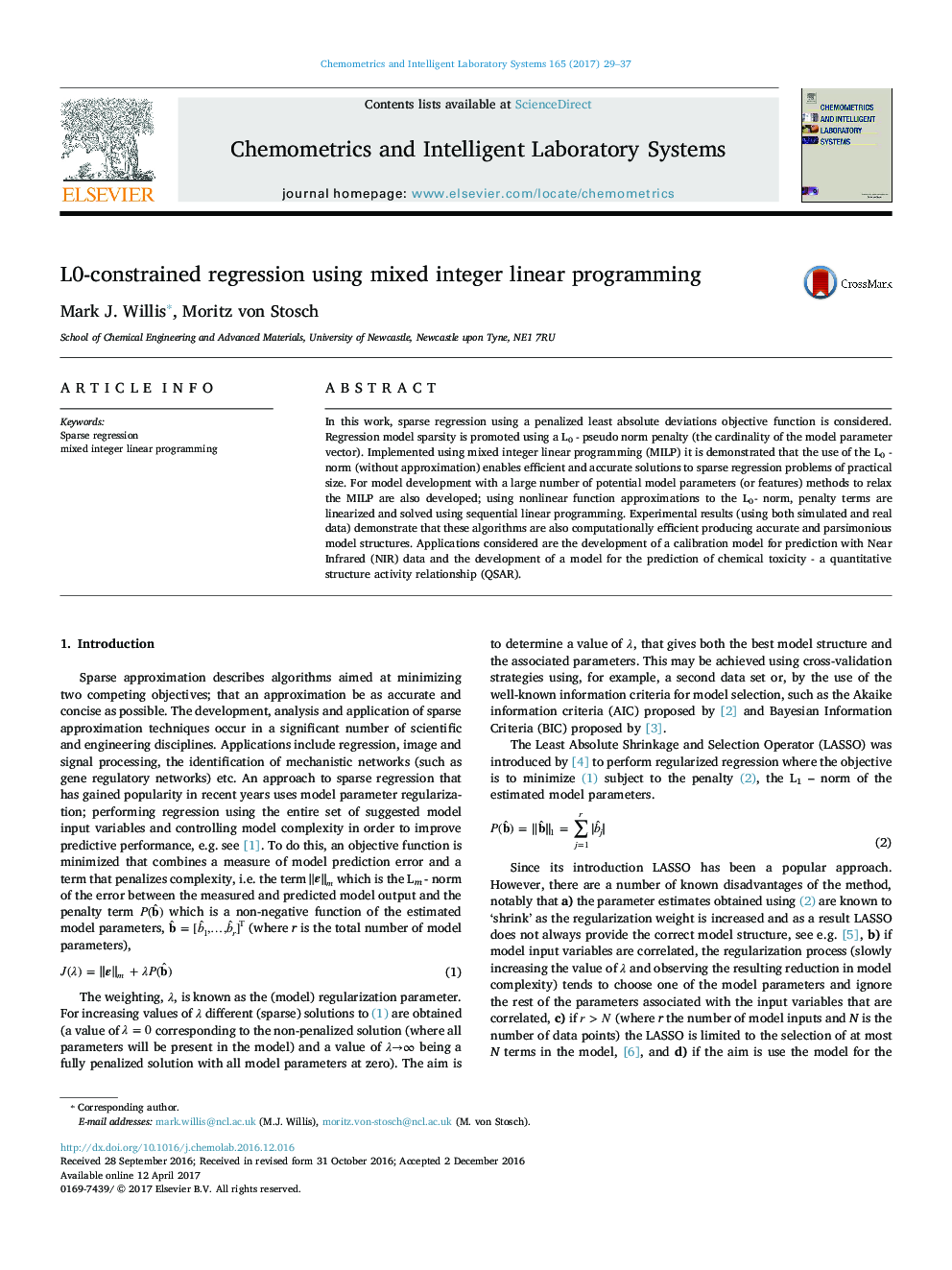
- A mixed integer linear programming (MILP) implementation of the sparse regression problem that uses the cardinality constraint directly is proposed. This provides accurate and parsimonious model structures for sparse regression problems of practical size.
- For model development with a large number of potential model parameters (or features) methods to relax the MILP are also developed; using nonlinear function approximations to the cardinality constraint, penalty terms are linearized and solved using sequential linear programming.
- Applications considered are the development of a calibration model for prediction with Near Infrared (NIR) data and the development of a model for the prediction of chemical toxicity - a quantitative structure activity relationship (QSAR).
In this work, sparse regression using a penalized least absolute deviations objective function is considered. Regression model sparsity is promoted using a L0 - pseudo norm penalty (the cardinality of the model parameter vector). Implemented using mixed integer linear programming (MILP) it is demonstrated that the use of the L0 - norm (without approximation) enables efficient and accurate solutions to sparse regression problems of practical size. For model development with a large number of potential model parameters (or features) methods to relax the MILP are also developed; using nonlinear function approximations to the L0- norm, penalty terms are linearized and solved using sequential linear programming. Experimental results (using both simulated and real data) demonstrate that these algorithms are also computationally efficient producing accurate and parsimonious model structures. Applications considered are the development of a calibration model for prediction with Near Infrared (NIR) data and the development of a model for the prediction of chemical toxicity - a quantitative structure activity relationship (QSAR).
Journal: Chemometrics and Intelligent Laboratory Systems - Volume 165, 15 June 2017, Pages 29-37