کد مقاله | کد نشریه | سال انتشار | مقاله انگلیسی | نسخه تمام متن |
---|---|---|---|---|
5132357 | 1491520 | 2016 | 19 صفحه PDF | دانلود رایگان |
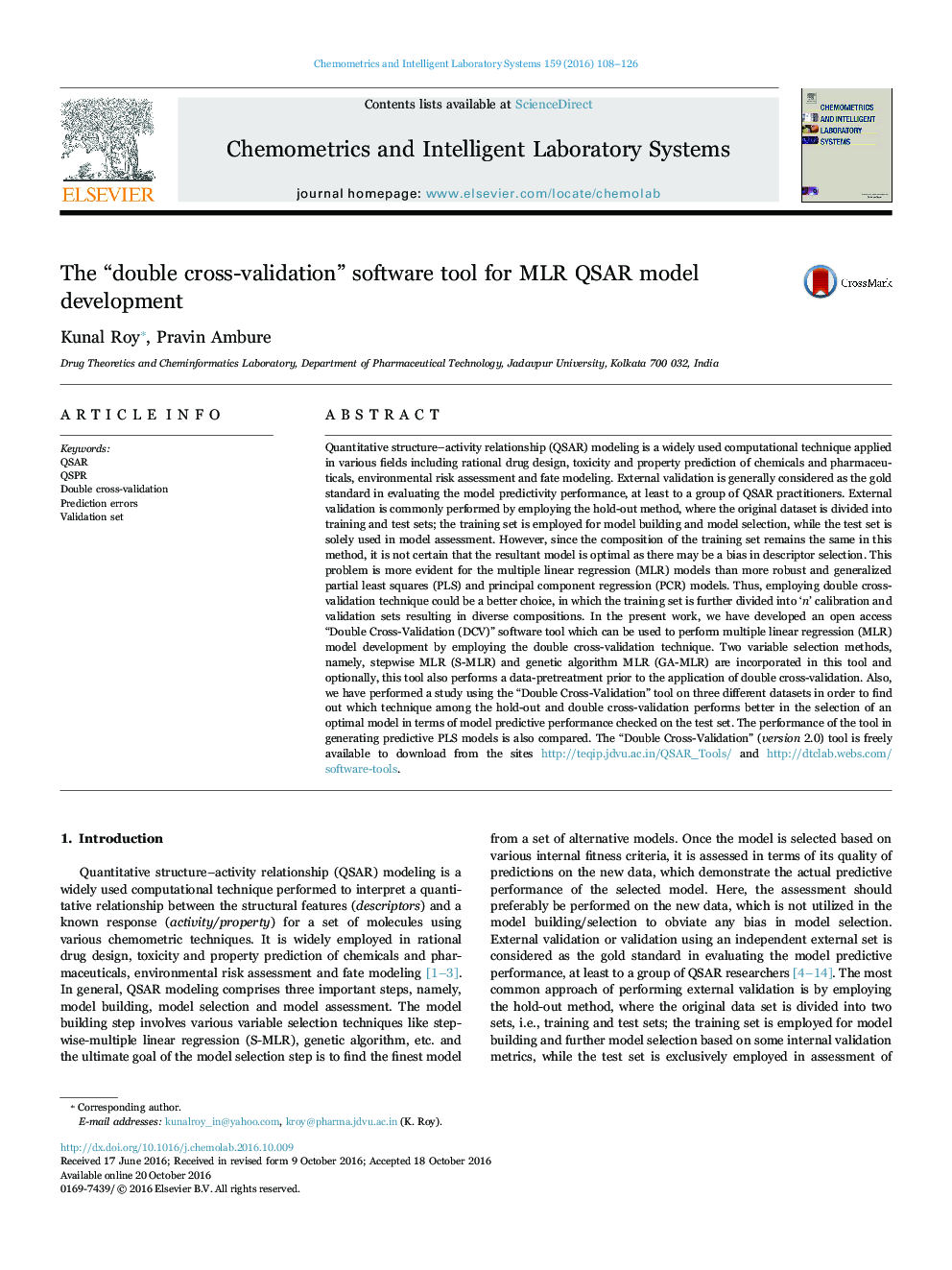
- Openly accessible “Double Cross-Validation (DCV)” software tool for MLR and PLS models has been developed.
- The tool is available via the websites http://teqip.jdvu.ac.in/QSAR_Tools/ and http://dtclab.webs.com/software-tools.
- The DCV tool is utilized to find an appropriate method of selecting the optimal predictive MLR and PLS QSAR models.
- We have compared the DCV technique with the conventional hold-out technique.
- We found that DCV is a better technique compared to the hold-out method for obtaining predictive MLR and PLS models.
Quantitative structure-activity relationship (QSAR) modeling is a widely used computational technique applied in various fields including rational drug design, toxicity and property prediction of chemicals and pharmaceuticals, environmental risk assessment and fate modeling. External validation is generally considered as the gold standard in evaluating the model predictivity performance, at least to a group of QSAR practitioners. External validation is commonly performed by employing the hold-out method, where the original dataset is divided into training and test sets; the training set is employed for model building and model selection, while the test set is solely used in model assessment. However, since the composition of the training set remains the same in this method, it is not certain that the resultant model is optimal as there may be a bias in descriptor selection. This problem is more evident for the multiple linear regression (MLR) models than more robust and generalized partial least squares (PLS) and principal component regression (PCR) models. Thus, employing double cross-validation technique could be a better choice, in which the training set is further divided into 'n' calibration and validation sets resulting in diverse compositions. In the present work, we have developed an open access “Double Cross-Validation (DCV)” software tool which can be used to perform multiple linear regression (MLR) model development by employing the double cross-validation technique. Two variable selection methods, namely, stepwise MLR (S-MLR) and genetic algorithm MLR (GA-MLR) are incorporated in this tool and optionally, this tool also performs a data-pretreatment prior to the application of double cross-validation. Also, we have performed a study using the “Double Cross-Validation” tool on three different datasets in order to find out which technique among the hold-out and double cross-validation performs better in the selection of an optimal model in terms of model predictive performance checked on the test set. The performance of the tool in generating predictive PLS models is also compared. The “Double Cross-Validation” (version 2.0) tool is freely available to download from the sites http://teqip.jdvu.ac.in/QSAR_Tools/ and http://dtclab.webs.com/software-tools.
Journal: Chemometrics and Intelligent Laboratory Systems - Volume 159, 15 December 2016, Pages 108-126