کد مقاله | کد نشریه | سال انتشار | مقاله انگلیسی | نسخه تمام متن |
---|---|---|---|---|
6346971 | 1621260 | 2014 | 11 صفحه PDF | دانلود رایگان |
عنوان انگلیسی مقاله ISI
Estimation of soil surface roughness of agricultural soils using airborne LiDAR
دانلود مقاله + سفارش ترجمه
دانلود مقاله ISI انگلیسی
رایگان برای ایرانیان
کلمات کلیدی
موضوعات مرتبط
مهندسی و علوم پایه
علوم زمین و سیارات
کامپیوتر در علوم زمین
پیش نمایش صفحه اول مقاله
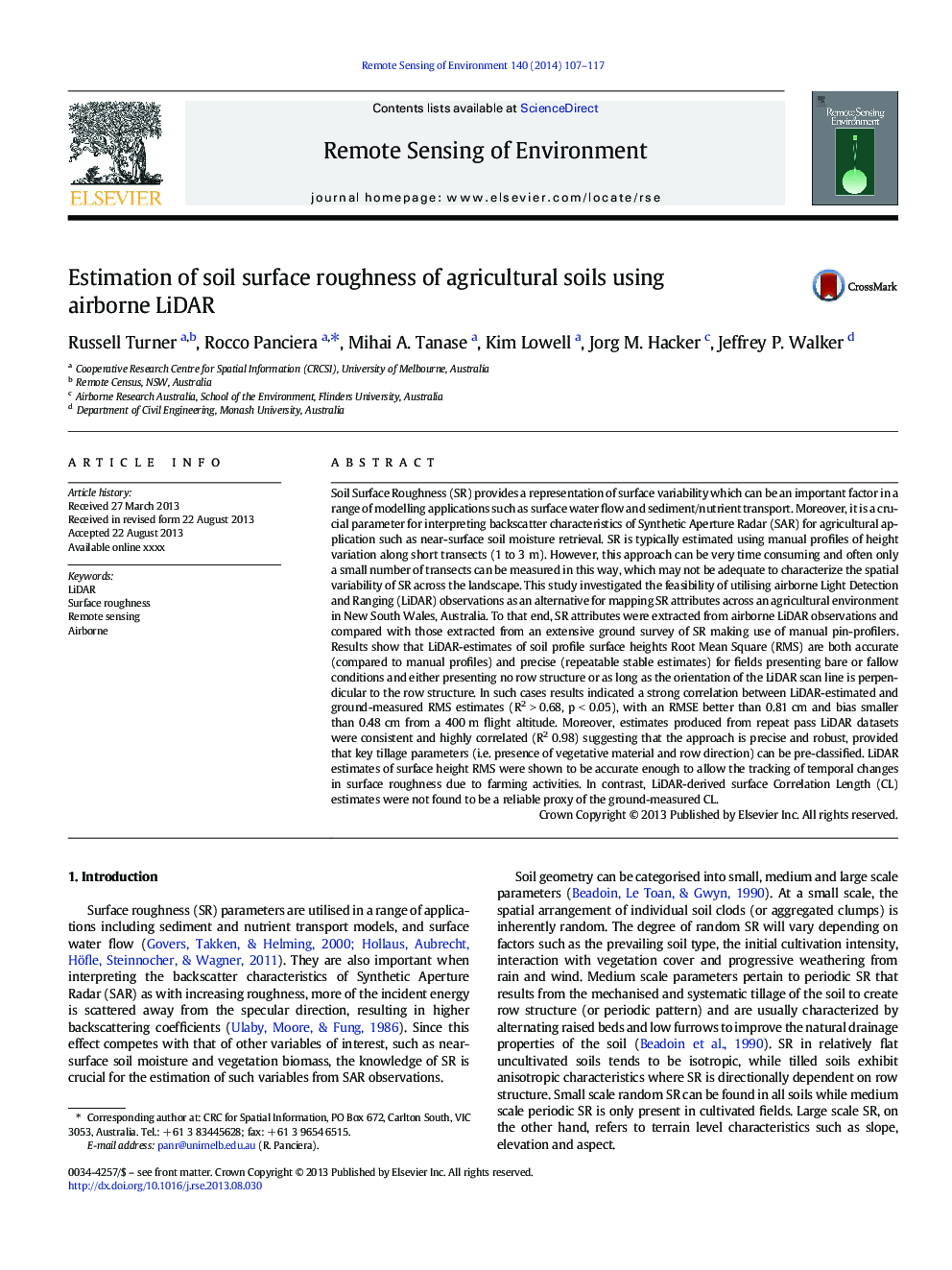
چکیده انگلیسی
Soil Surface Roughness (SR) provides a representation of surface variability which can be an important factor in a range of modelling applications such as surface water flow and sediment/nutrient transport. Moreover, it is a crucial parameter for interpreting backscatter characteristics of Synthetic Aperture Radar (SAR) for agricultural application such as near-surface soil moisture retrieval. SR is typically estimated using manual profiles of height variation along short transects (1 to 3 m). However, this approach can be very time consuming and often only a small number of transects can be measured in this way, which may not be adequate to characterize the spatial variability of SR across the landscape. This study investigated the feasibility of utilising airborne Light Detection and Ranging (LiDAR) observations as an alternative for mapping SR attributes across an agricultural environment in New South Wales, Australia. To that end, SR attributes were extracted from airborne LiDAR observations and compared with those extracted from an extensive ground survey of SR making use of manual pin-profilers. Results show that LiDAR-estimates of soil profile surface heights Root Mean Square (RMS) are both accurate (compared to manual profiles) and precise (repeatable stable estimates) for fields presenting bare or fallow conditions and either presenting no row structure or as long as the orientation of the LiDAR scan line is perpendicular to the row structure. In such cases results indicated a strong correlation between LiDAR-estimated and ground-measured RMS estimates (R2 > 0.68, p < 0.05), with an RMSE better than 0.81 cm and bias smaller than 0.48 cm from a 400 m flight altitude. Moreover, estimates produced from repeat pass LiDAR datasets were consistent and highly correlated (R2 0.98) suggesting that the approach is precise and robust, provided that key tillage parameters (i.e. presence of vegetative material and row direction) can be pre-classified. LiDAR estimates of surface height RMS were shown to be accurate enough to allow the tracking of temporal changes in surface roughness due to farming activities. In contrast, LiDAR-derived surface Correlation Length (CL) estimates were not found to be a reliable proxy of the ground-measured CL.
ناشر
Database: Elsevier - ScienceDirect (ساینس دایرکت)
Journal: Remote Sensing of Environment - Volume 140, January 2014, Pages 107-117
Journal: Remote Sensing of Environment - Volume 140, January 2014, Pages 107-117
نویسندگان
Russell Turner, Rocco Panciera, Mihai A. Tanase, Kim Lowell, Jorg M. Hacker, Jeffrey P. Walker,