کد مقاله | کد نشریه | سال انتشار | مقاله انگلیسی | نسخه تمام متن |
---|---|---|---|---|
6411412 | 1629926 | 2015 | 15 صفحه PDF | دانلود رایگان |
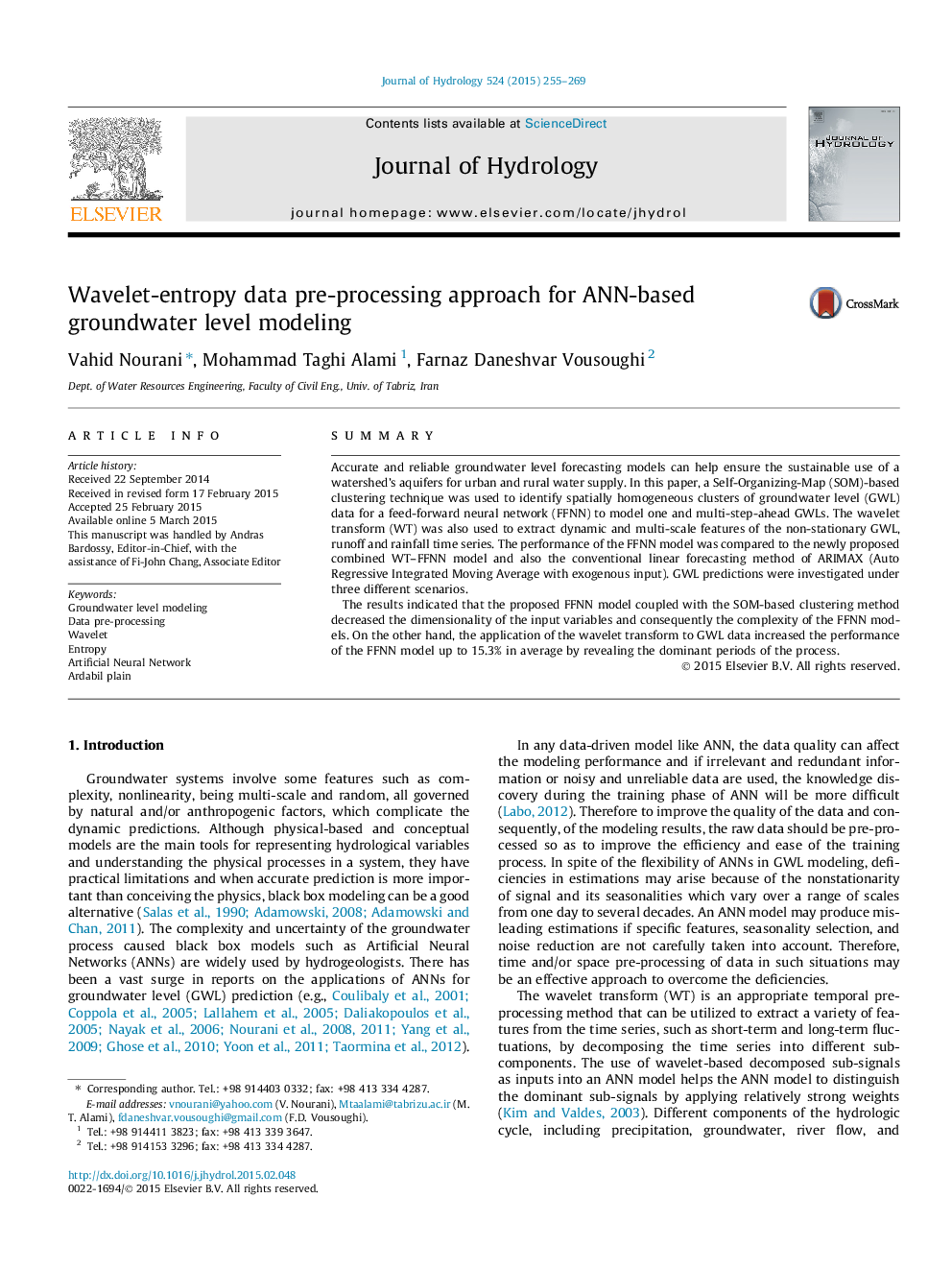
- ANN-based models developed for multi-step-ahead groundwater level predictions.
- Clustering and wavelet-based data pre-processing methods were employed.
- Dominant inputs were selected via Mutual Information criterion.
- Spatial data processing by SOM clustering decreased dimensionality of input data.
- Wavelet temporal pre-processing improved performance of multi-step-ahead modeling.
SummaryAccurate and reliable groundwater level forecasting models can help ensure the sustainable use of a watershed's aquifers for urban and rural water supply. In this paper, a Self-Organizing-Map (SOM)-based clustering technique was used to identify spatially homogeneous clusters of groundwater level (GWL) data for a feed-forward neural network (FFNN) to model one and multi-step-ahead GWLs. The wavelet transform (WT) was also used to extract dynamic and multi-scale features of the non-stationary GWL, runoff and rainfall time series. The performance of the FFNN model was compared to the newly proposed combined WT-FFNN model and also the conventional linear forecasting method of ARIMAX (Auto Regressive Integrated Moving Average with exogenous input). GWL predictions were investigated under three different scenarios.The results indicated that the proposed FFNN model coupled with the SOM-based clustering method decreased the dimensionality of the input variables and consequently the complexity of the FFNN models. On the other hand, the application of the wavelet transform to GWL data increased the performance of the FFNN model up to 15.3% in average by revealing the dominant periods of the process.
Journal: Journal of Hydrology - Volume 524, May 2015, Pages 255-269