کد مقاله | کد نشریه | سال انتشار | مقاله انگلیسی | نسخه تمام متن |
---|---|---|---|---|
6679608 | 1428034 | 2018 | 16 صفحه PDF | دانلود رایگان |
عنوان انگلیسی مقاله ISI
Short-term electricity demand forecasting with MARS, SVR and ARIMA models using aggregated demand data in Queensland, Australia
دانلود مقاله + سفارش ترجمه
دانلود مقاله ISI انگلیسی
رایگان برای ایرانیان
کلمات کلیدی
σ2PACFENSAEMOGCVAustralian Energy Market OperatorMSEACFARIMAAICQ50Q25National Electricity MarketRMSERBFSVRANNlog likelihood - احتمال ورودGeneralized cross-validation - اعتبار کلی متناوبELM - المMAE - بلهAuto-correlation function - تابع خودکار همبستگیMultivariate adaptive regression splines - تنوع رگرسیون چند متغیره انطباقیSupport vector regression - رگرسیون بردار پشتیبانیMars - سیاره مریخArtificial Neural Network - شبکه عصبی مصنوعیcoefficient of determination - ضریب تعیینCorrelation coefficient - ضریب همبستگیAkaike information criterion - معیار اطلاعاتی آکائیکmegawatt - مگاواتAutoregressive Integrated Moving Average - میانگین متحرک متحرک AutoregressiveNEM - نهVariance - واریانس، ورداییElectricity demand forecasting - پیش بینی تقاضای برقMachine learning - یادگیری ماشین
موضوعات مرتبط
مهندسی و علوم پایه
مهندسی کامپیوتر
هوش مصنوعی
پیش نمایش صفحه اول مقاله
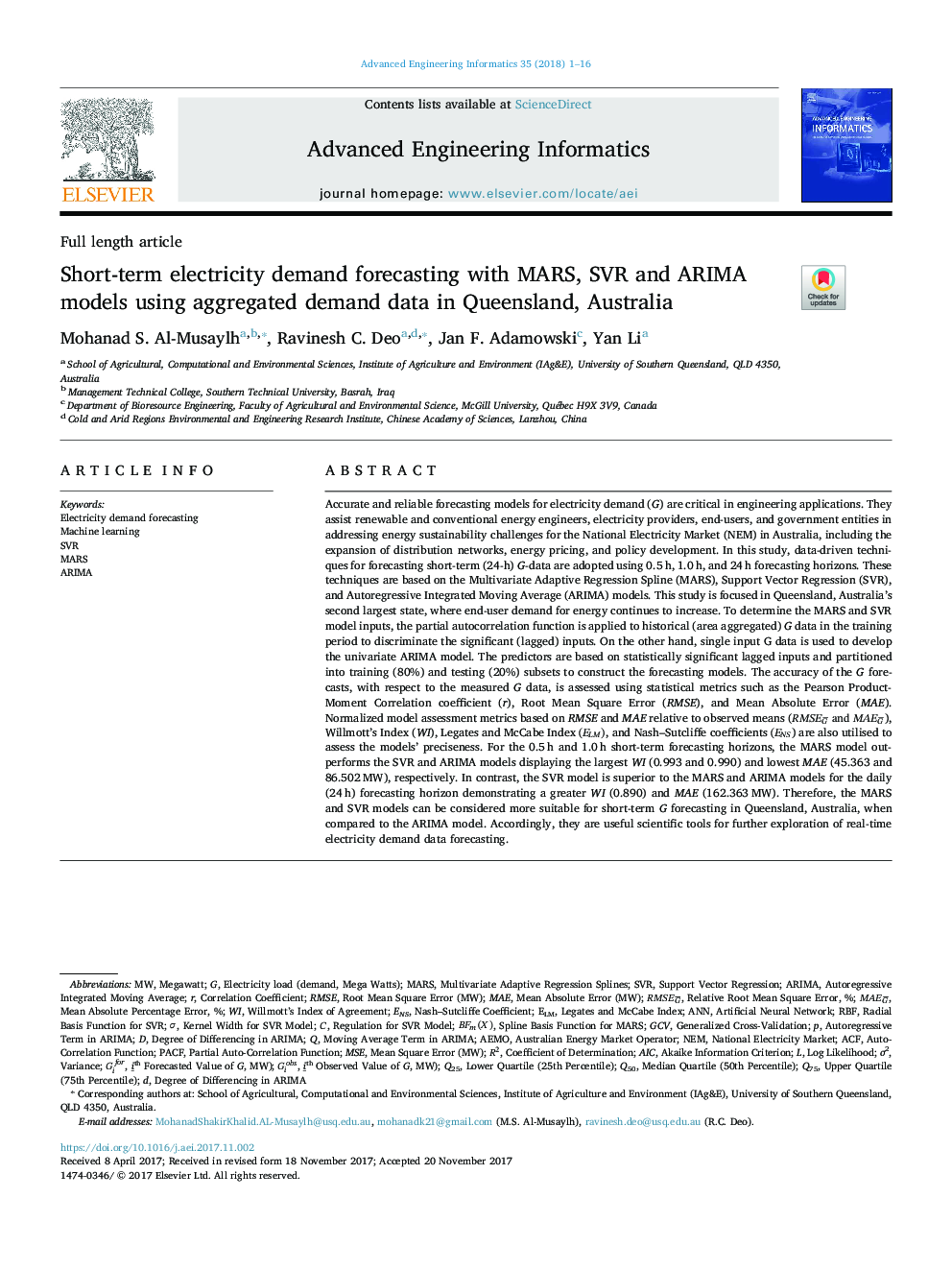
چکیده انگلیسی
Accurate and reliable forecasting models for electricity demand (G) are critical in engineering applications. They assist renewable and conventional energy engineers, electricity providers, end-users, and government entities in addressing energy sustainability challenges for the National Electricity Market (NEM) in Australia, including the expansion of distribution networks, energy pricing, and policy development. In this study, data-driven techniques for forecasting short-term (24-h) G-data are adopted using 0.5â¯h, 1.0â¯h, and 24â¯h forecasting horizons. These techniques are based on the Multivariate Adaptive Regression Spline (MARS), Support Vector Regression (SVR), and Autoregressive Integrated Moving Average (ARIMA) models. This study is focused in Queensland, Australia's second largest state, where end-user demand for energy continues to increase. To determine the MARS and SVR model inputs, the partial autocorrelation function is applied to historical (area aggregated) G data in the training period to discriminate the significant (lagged) inputs. On the other hand, single input G data is used to develop the univariate ARIMA model. The predictors are based on statistically significant lagged inputs and partitioned into training (80%) and testing (20%) subsets to construct the forecasting models. The accuracy of the G forecasts, with respect to the measured G data, is assessed using statistical metrics such as the Pearson Product-Moment Correlation coefficient (r), Root Mean Square Error (RMSE), and Mean Absolute Error (MAE). Normalized model assessment metrics based on RMSE and MAE relative to observed means (RMSEG¯andMAEG¯), Willmott's Index (WI), Legates and McCabe Index (ELM), and Nash-Sutcliffe coefficients (ENS) are also utilised to assess the models' preciseness. For the 0.5â¯h and 1.0â¯h short-term forecasting horizons, the MARS model outperforms the SVR and ARIMA models displaying the largest WI (0.993 and 0.990) and lowest MAE (45.363 and 86.502â¯MW), respectively. In contrast, the SVR model is superior to the MARS and ARIMA models for the daily (24â¯h) forecasting horizon demonstrating a greater WI (0.890) and MAE (162.363â¯MW). Therefore, the MARS and SVR models can be considered more suitable for short-term G forecasting in Queensland, Australia, when compared to the ARIMA model. Accordingly, they are useful scientific tools for further exploration of real-time electricity demand data forecasting.
ناشر
Database: Elsevier - ScienceDirect (ساینس دایرکت)
Journal: Advanced Engineering Informatics - Volume 35, January 2018, Pages 1-16
Journal: Advanced Engineering Informatics - Volume 35, January 2018, Pages 1-16
نویسندگان
Mohanad S. Al-Musaylh, Ravinesh C. Deo, Jan F. Adamowski, Yan Li,