کد مقاله | کد نشریه | سال انتشار | مقاله انگلیسی | نسخه تمام متن |
---|---|---|---|---|
688891 | 1460377 | 2015 | 17 صفحه PDF | دانلود رایگان |
عنوان انگلیسی مقاله ISI
Comparison of variable selection methods for PLS-based soft sensor modeling
دانلود مقاله + سفارش ترجمه
دانلود مقاله ISI انگلیسی
رایگان برای ایرانیان
کلمات کلیدی
موضوعات مرتبط
مهندسی و علوم پایه
مهندسی شیمی
تکنولوژی و شیمی فرآیندی
پیش نمایش صفحه اول مقاله
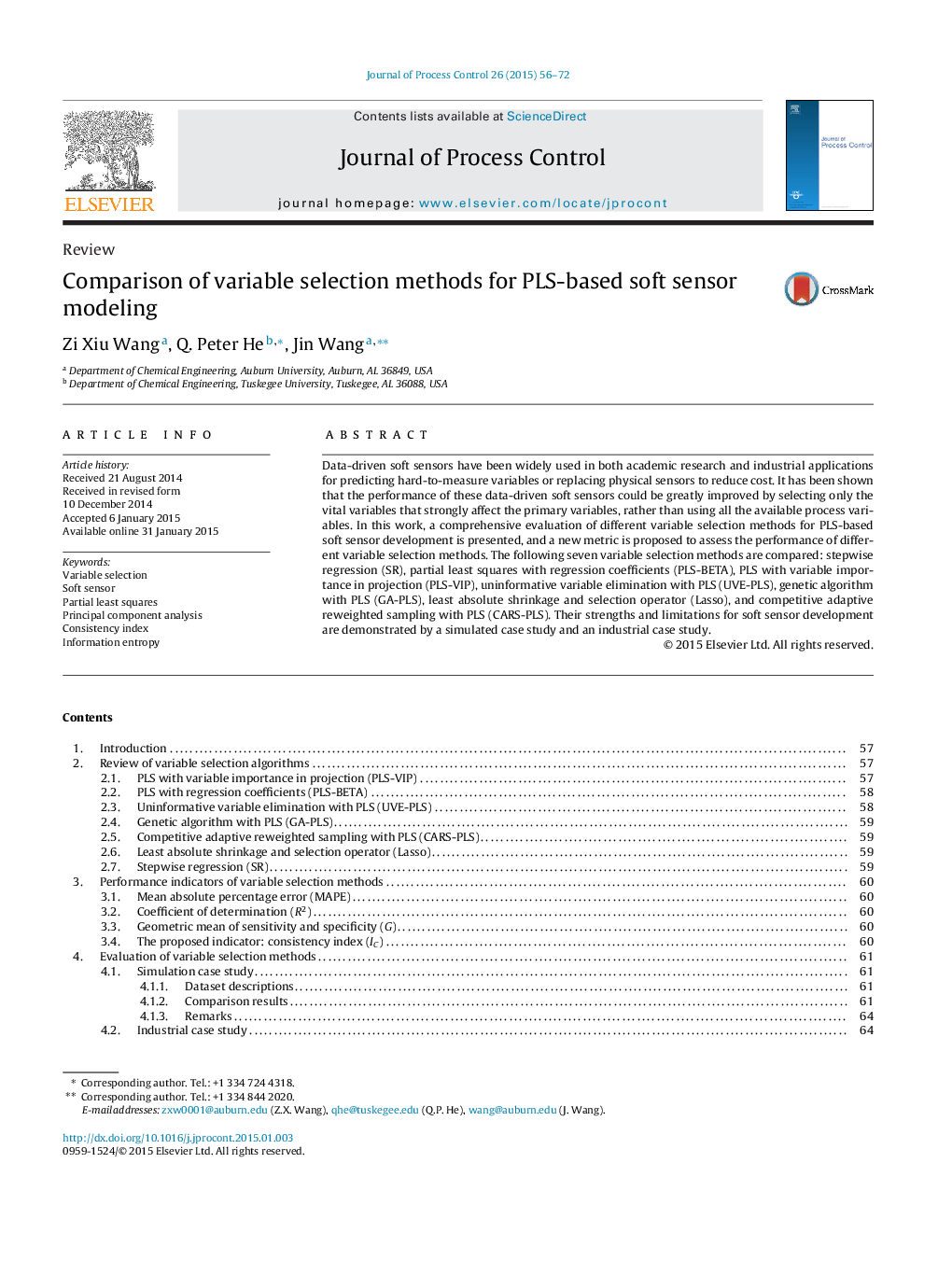
چکیده انگلیسی
Data-driven soft sensors have been widely used in both academic research and industrial applications for predicting hard-to-measure variables or replacing physical sensors to reduce cost. It has been shown that the performance of these data-driven soft sensors could be greatly improved by selecting only the vital variables that strongly affect the primary variables, rather than using all the available process variables. In this work, a comprehensive evaluation of different variable selection methods for PLS-based soft sensor development is presented, and a new metric is proposed to assess the performance of different variable selection methods. The following seven variable selection methods are compared: stepwise regression (SR), partial least squares with regression coefficients (PLS-BETA), PLS with variable importance in projection (PLS-VIP), uninformative variable elimination with PLS (UVE-PLS), genetic algorithm with PLS (GA-PLS), least absolute shrinkage and selection operator (Lasso), and competitive adaptive reweighted sampling with PLS (CARS-PLS). Their strengths and limitations for soft sensor development are demonstrated by a simulated case study and an industrial case study.
ناشر
Database: Elsevier - ScienceDirect (ساینس دایرکت)
Journal: Journal of Process Control - Volume 26, February 2015, Pages 56-72
Journal: Journal of Process Control - Volume 26, February 2015, Pages 56-72
نویسندگان
Zi Xiu Wang, Q. Peter He, Jin Wang,