کد مقاله | کد نشریه | سال انتشار | مقاله انگلیسی | نسخه تمام متن |
---|---|---|---|---|
8894892 | 1629893 | 2018 | 35 صفحه PDF | دانلود رایگان |
عنوان انگلیسی مقاله ISI
Developing a Long Short-Term Memory (LSTM) based model for predicting water table depth in agricultural areas
دانلود مقاله + سفارش ترجمه
دانلود مقاله ISI انگلیسی
رایگان برای ایرانیان
کلمات کلیدی
موضوعات مرتبط
مهندسی و علوم پایه
علوم زمین و سیارات
فرآیندهای سطح زمین
پیش نمایش صفحه اول مقاله
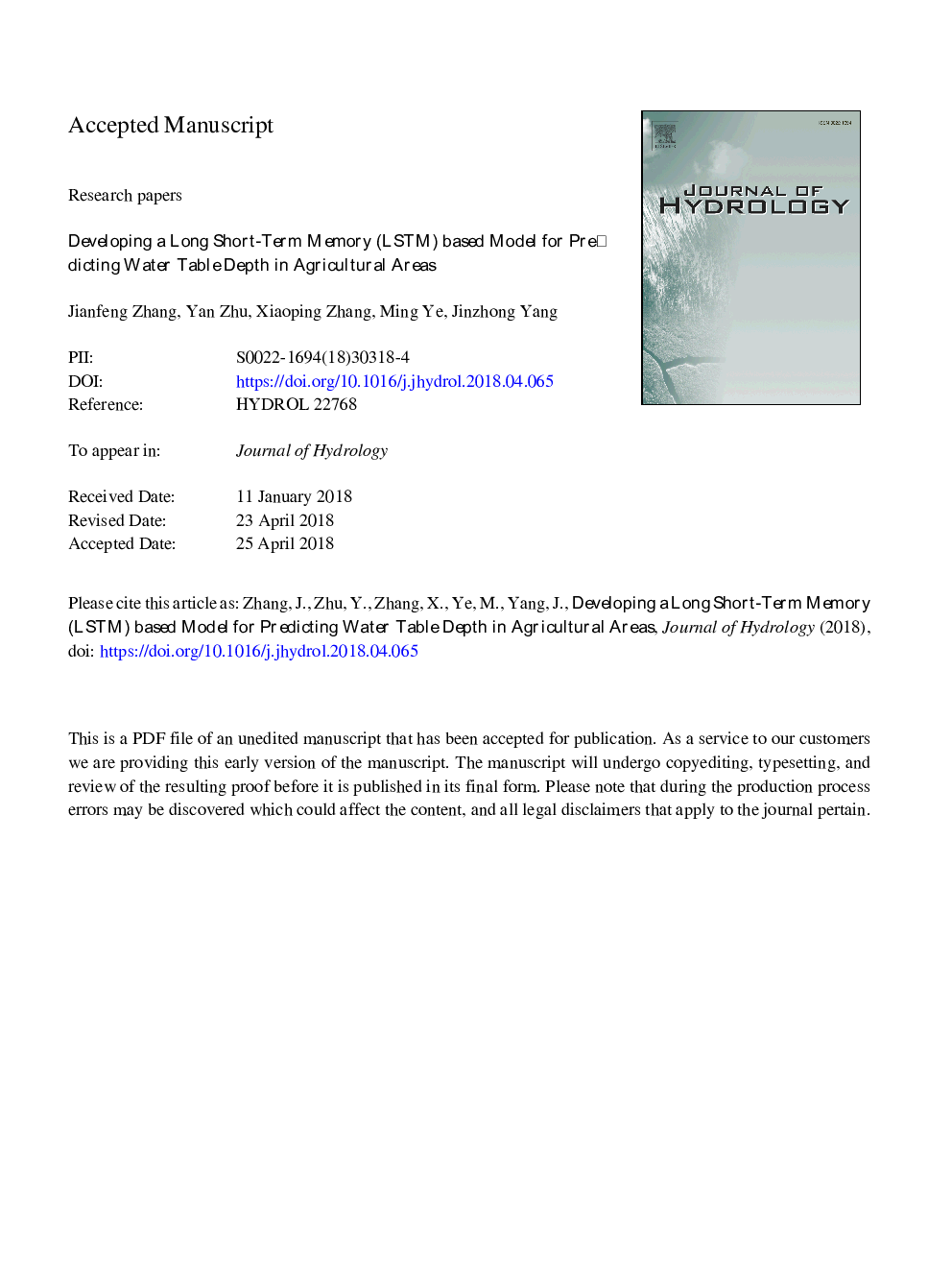
چکیده انگلیسی
Predicting water table depth over the long-term in agricultural areas presents great challenges because these areas have complex and heterogeneous hydrogeological characteristics, boundary conditions, and human activities; also, nonlinear interactions occur among these factors. Therefore, a new time series model based on Long Short-Term Memory (LSTM), was developed in this study as an alternative to computationally expensive physical models. The proposed model is composed of an LSTM layer with another fully connected layer on top of it, with a dropout method applied in the first LSTM layer. In this study, the proposed model was applied and evaluated in five sub-areas of Hetao Irrigation District in arid northwestern China using data of 14â¯years (2000-2013). The proposed model uses monthly water diversion, evaporation, precipitation, temperature, and time as input data to predict water table depth. A simple but effective standardization method was employed to pre-process data to ensure data on the same scale. 14â¯years of data are separated into two sets: training set (2000-2011) and validation set (2012-2013) in the experiment. As expected, the proposed model achieves higher R2 scores (0.789-0.952) in water table depth prediction, when compared with the results of traditional feed-forward neural network (FFNN), which only reaches relatively low R2 scores (0.004-0.495), proving that the proposed model can preserve and learn previous information well. Furthermore, the validity of the dropout method and the proposed model's architecture are discussed. Through experimentation, the results show that the dropout method can prevent overfitting significantly. In addition, comparisons between the R2 scores of the proposed model and Double-LSTM model (R2 scores range from 0.170 to 0.864), further prove that the proposed model's architecture is reasonable and can contribute to a strong learning ability on time series data. Thus, one can conclude that the proposed model can serve as an alternative approach predicting water table depth, especially in areas where hydrogeological data are difficult to obtain.
ناشر
Database: Elsevier - ScienceDirect (ساینس دایرکت)
Journal: Journal of Hydrology - Volume 561, June 2018, Pages 918-929
Journal: Journal of Hydrology - Volume 561, June 2018, Pages 918-929
نویسندگان
Jianfeng Zhang, Yan Zhu, Xiaoping Zhang, Ming Ye, Jinzhong Yang,