کد مقاله | کد نشریه | سال انتشار | مقاله انگلیسی | نسخه تمام متن |
---|---|---|---|---|
10118270 | 1629890 | 2018 | 50 صفحه PDF | دانلود رایگان |
عنوان انگلیسی مقاله ISI
A spatial downscaling of soil moisture from rainfall, temperature, and AMSR2 using a Gaussian-mixture nonstationary hidden Markov model
دانلود مقاله + سفارش ترجمه
دانلود مقاله ISI انگلیسی
رایگان برای ایرانیان
کلمات کلیدی
موضوعات مرتبط
مهندسی و علوم پایه
علوم زمین و سیارات
فرآیندهای سطح زمین
پیش نمایش صفحه اول مقاله
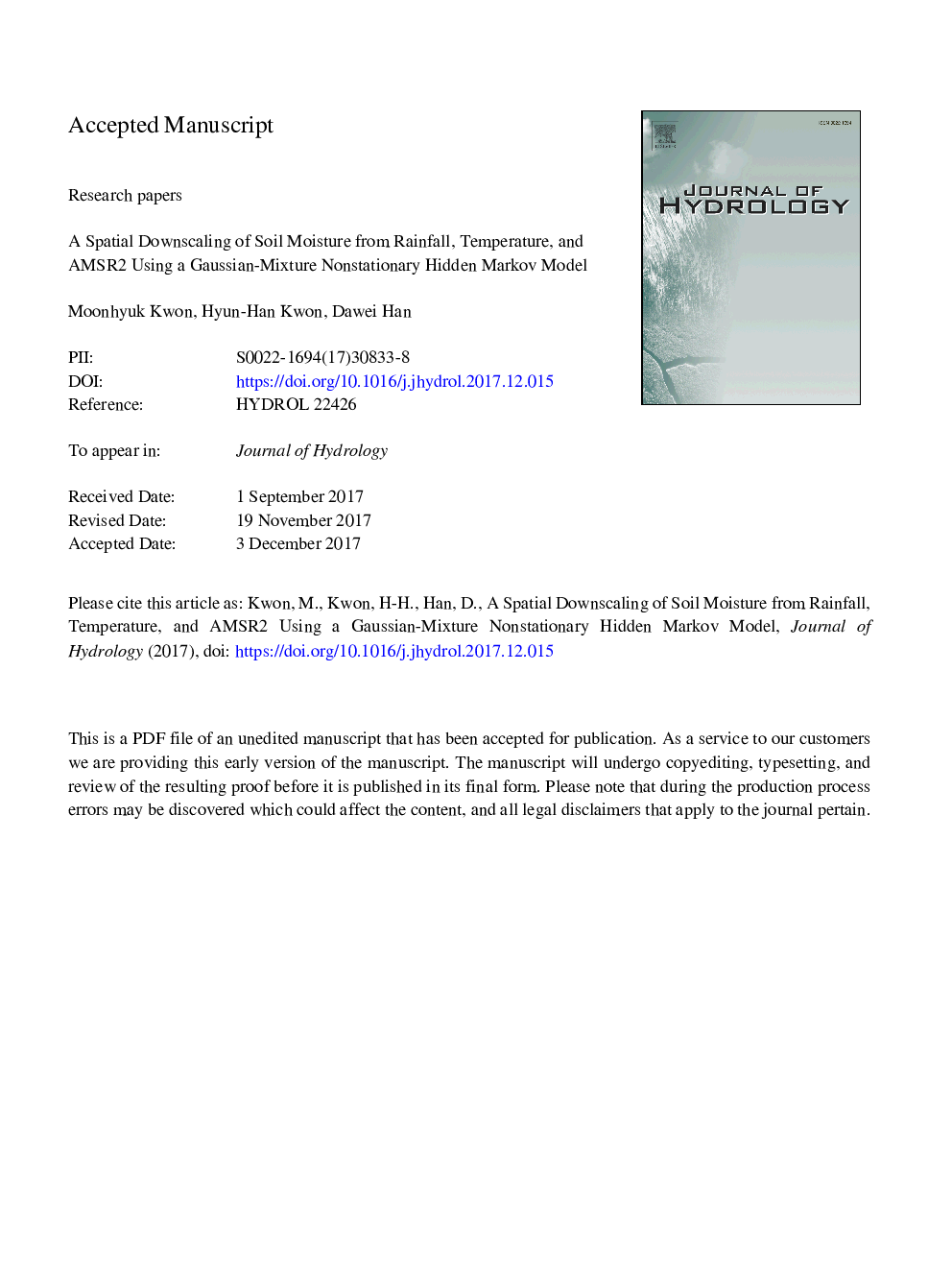
چکیده انگلیسی
A multivariate stochastic soil moisture (SM) estimation approach based on a Gaussian-mixture nonstationary hidden Markov model (GM-NHMM) is introduced in this study to spatially disaggregate the AMSR2 SM data for multiple locations in the Yongdam dam watershed in South Korea. Rainfall and air temperature are considered as additional predictors in the proposed modeling framework. In GM-NHMM, a six-state model is constructed with three predictors representing an unobserved state associated with SM. It is clearly seen that the rainfall predictor plays a substantial role in achieving the overall predictability. Using weather variables (i.e., rainfall and temperature) can be effective in picking up some of the predictability of local SM that is not captured by the AMSR2 data. On the other hand, larger scale dynamic features identified from the AMSR2 data seem to facilitate the identification of regional spatial patterns of SM. The efficiency of the proposed model is compared with that of an ordinary regression model (OLR) using the same predictors. The mean correlation coefficient of the proposed model is about 0.78, which is significantly greater than that of the OLR at about 0.49. The proposed GM-NHMM method not only provides a better representation of the observed SM than the OLR model but also preserves the spatial coherence across all stations reasonably well.
ناشر
Database: Elsevier - ScienceDirect (ساینس دایرکت)
Journal: Journal of Hydrology - Volume 564, September 2018, Pages 1194-1207
Journal: Journal of Hydrology - Volume 564, September 2018, Pages 1194-1207
نویسندگان
Moonhyuk Kwon, Hyun-Han Kwon, Dawei Han,