کد مقاله | کد نشریه | سال انتشار | مقاله انگلیسی | نسخه تمام متن |
---|---|---|---|---|
10138831 | 1645909 | 2019 | 39 صفحه PDF | دانلود رایگان |
عنوان انگلیسی مقاله ISI
Robust optimization of mixed CVaR STARR ratio using copulas
دانلود مقاله + سفارش ترجمه
دانلود مقاله ISI انگلیسی
رایگان برای ایرانیان
موضوعات مرتبط
مهندسی و علوم پایه
ریاضیات
ریاضیات کاربردی
پیش نمایش صفحه اول مقاله
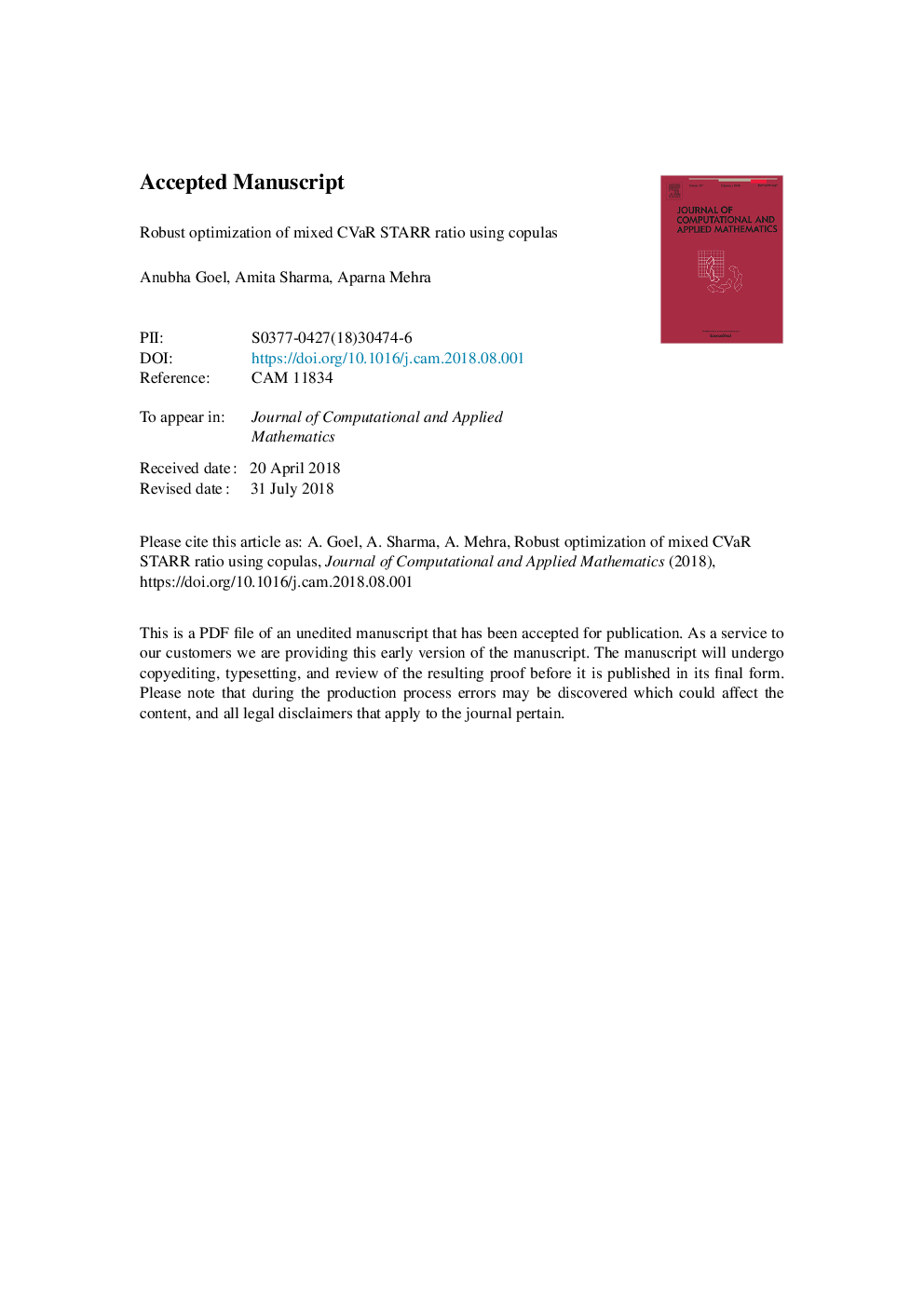
چکیده انگلیسی
We introduce the robust optimization models for two variants of stable tail-adjusted return ratio (STARR), one with mixed conditional value-at-risk (MCVaR) and the other with deviation MCVaR (DMCVaR), under joint ambiguity in the distribution modeled using copulas. The two models are shown to be computationally tractable linear programs. We apply a two-step procedure to capture the joint dependence structure among the assets. We first extract the filtered residuals from the return series of each asset using AutoRegressive Moving Average Glosten Jagannathan Runkle Generalized Autoregressive Conditional Heteroscedastic (ARMA-GJR-GARCH) model. Subsequently, we exploit the regular vine copulas to model the joint dependence among the transformed residuals. The tree structure in the regular vines is accomplished using Kendall's tau. We compare the performance of the proposed two robust models with their conventional counterparts when the joint distribution in the latter is described using Gaussian copula only. We also examine the performance of the obtained portfolios against those from the Markowitz model and multivariate GARCH models using the rolling window analysis. We illustrate the superior performance of the proposed robust models than their conventional counterpart models on excess mean returns, Sortino ratio, Rachev ratio, VaR ratio, and Treynor ratio, on three data sets comprising of indices across the globe.
ناشر
Database: Elsevier - ScienceDirect (ساینس دایرکت)
Journal: Journal of Computational and Applied Mathematics - Volume 347, February 2019, Pages 62-83
Journal: Journal of Computational and Applied Mathematics - Volume 347, February 2019, Pages 62-83
نویسندگان
Anubha Goel, Amita Sharma, Aparna Mehra,