کد مقاله | کد نشریه | سال انتشار | مقاله انگلیسی | نسخه تمام متن |
---|---|---|---|---|
1181089 | 962898 | 2009 | 11 صفحه PDF | دانلود رایگان |
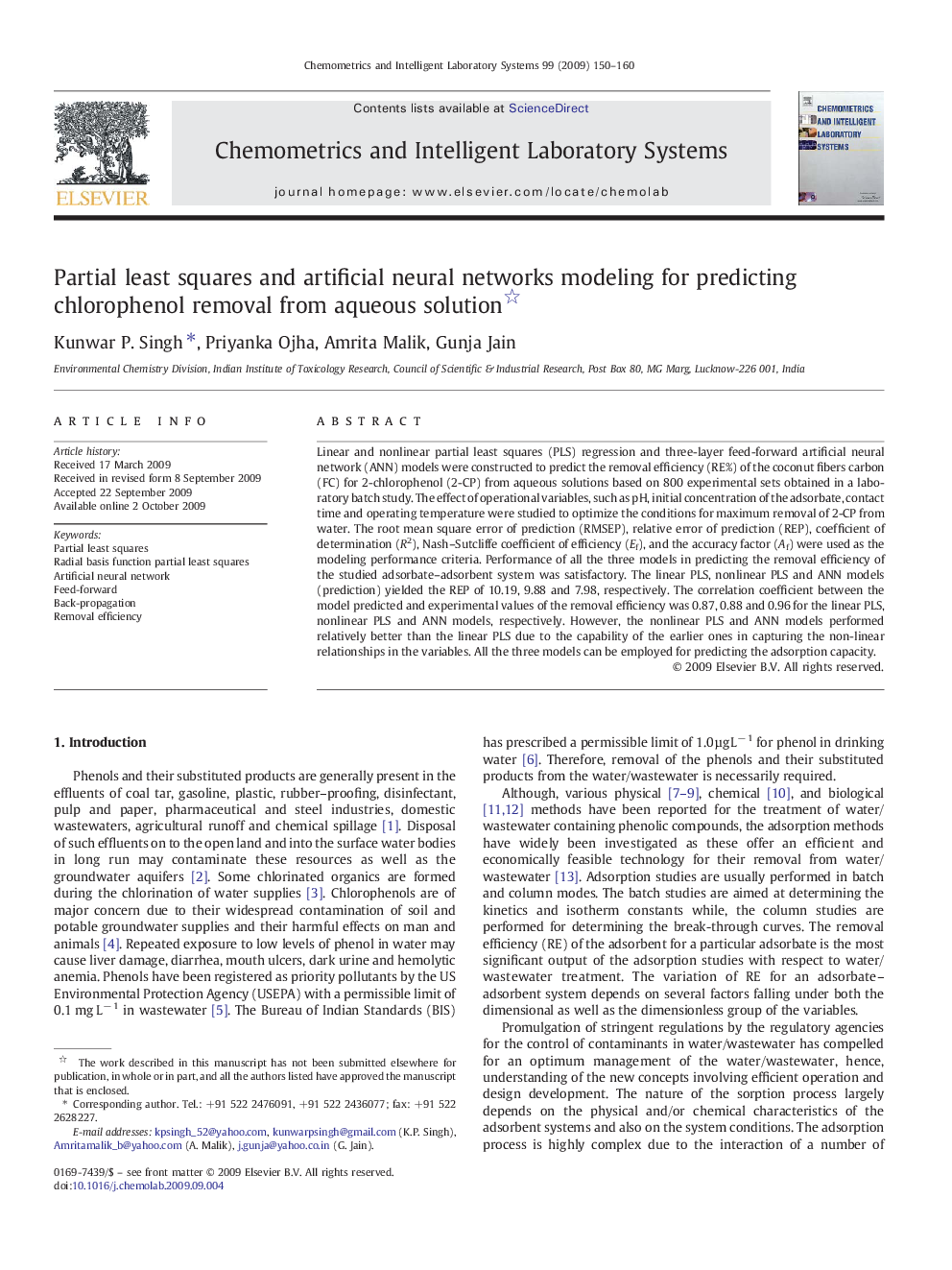
Linear and nonlinear partial least squares (PLS) regression and three-layer feed-forward artificial neural network (ANN) models were constructed to predict the removal efficiency (RE%) of the coconut fibers carbon (FC) for 2-chlorophenol (2-CP) from aqueous solutions based on 800 experimental sets obtained in a laboratory batch study. The effect of operational variables, such as pH, initial concentration of the adsorbate, contact time and operating temperature were studied to optimize the conditions for maximum removal of 2-CP from water. The root mean square error of prediction (RMSEP), relative error of prediction (REP), coefficient of determination (R2), Nash–Sutcliffe coefficient of efficiency (Ef), and the accuracy factor (Af) were used as the modeling performance criteria. Performance of all the three models in predicting the removal efficiency of the studied adsorbate–adsorbent system was satisfactory. The linear PLS, nonlinear PLS and ANN models (prediction) yielded the REP of 10.19, 9.88 and 7.98, respectively. The correlation coefficient between the model predicted and experimental values of the removal efficiency was 0.87, 0.88 and 0.96 for the linear PLS, nonlinear PLS and ANN models, respectively. However, the nonlinear PLS and ANN models performed relatively better than the linear PLS due to the capability of the earlier ones in capturing the non-linear relationships in the variables. All the three models can be employed for predicting the adsorption capacity.
Journal: Chemometrics and Intelligent Laboratory Systems - Volume 99, Issue 2, 15 December 2009, Pages 150–160