کد مقاله | کد نشریه | سال انتشار | مقاله انگلیسی | نسخه تمام متن |
---|---|---|---|---|
1181588 | 962960 | 2010 | 12 صفحه PDF | دانلود رایگان |
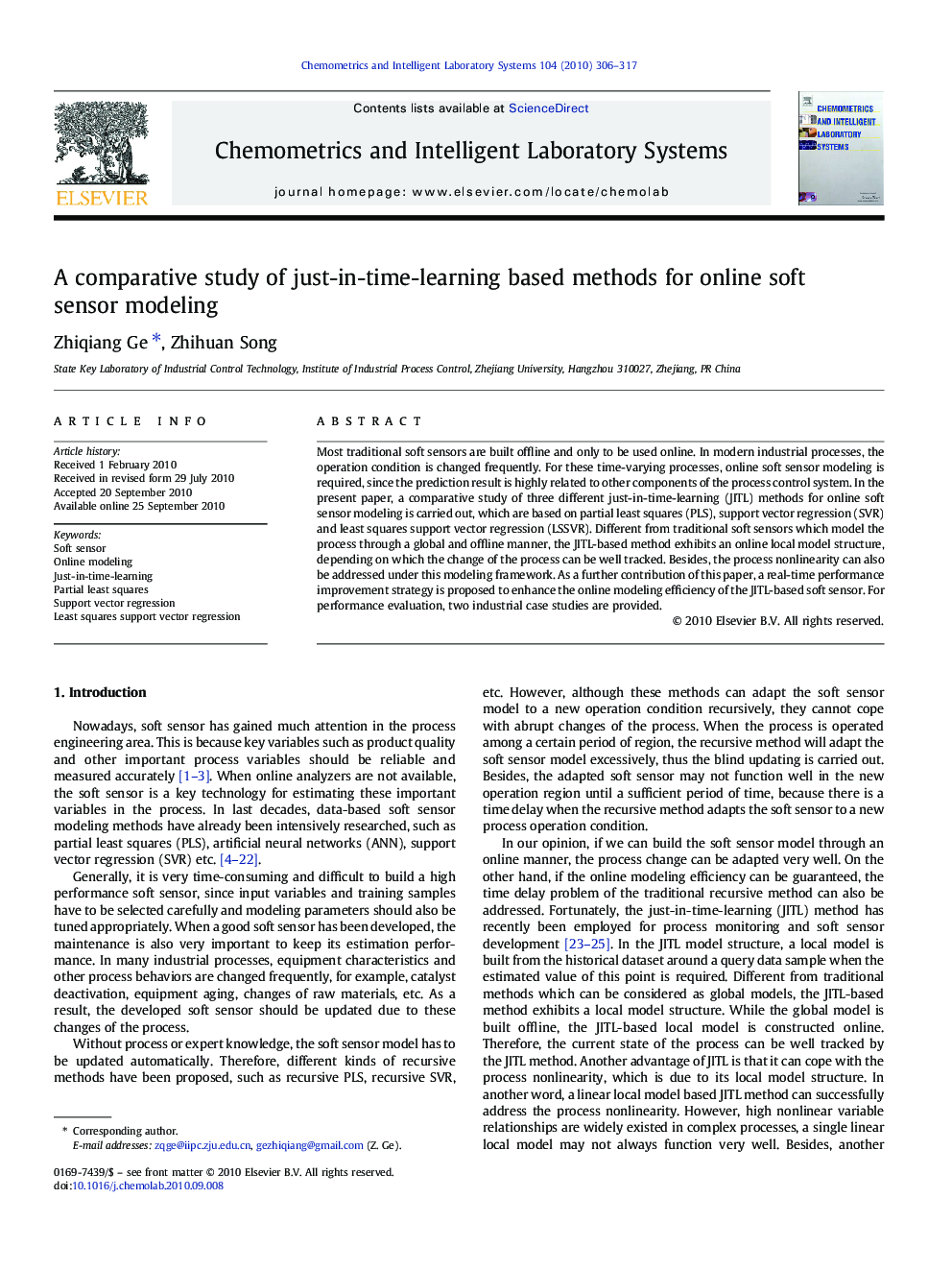
Most traditional soft sensors are built offline and only to be used online. In modern industrial processes, the operation condition is changed frequently. For these time-varying processes, online soft sensor modeling is required, since the prediction result is highly related to other components of the process control system. In the present paper, a comparative study of three different just-in-time-learning (JITL) methods for online soft sensor modeling is carried out, which are based on partial least squares (PLS), support vector regression (SVR) and least squares support vector regression (LSSVR). Different from traditional soft sensors which model the process through a global and offline manner, the JITL-based method exhibits an online local model structure, depending on which the change of the process can be well tracked. Besides, the process nonlinearity can also be addressed under this modeling framework. As a further contribution of this paper, a real-time performance improvement strategy is proposed to enhance the online modeling efficiency of the JITL-based soft sensor. For performance evaluation, two industrial case studies are provided.
Journal: Chemometrics and Intelligent Laboratory Systems - Volume 104, Issue 2, 15 December 2010, Pages 306–317