کد مقاله | کد نشریه | سال انتشار | مقاله انگلیسی | نسخه تمام متن |
---|---|---|---|---|
310864 | 533392 | 2010 | 9 صفحه PDF | دانلود رایگان |
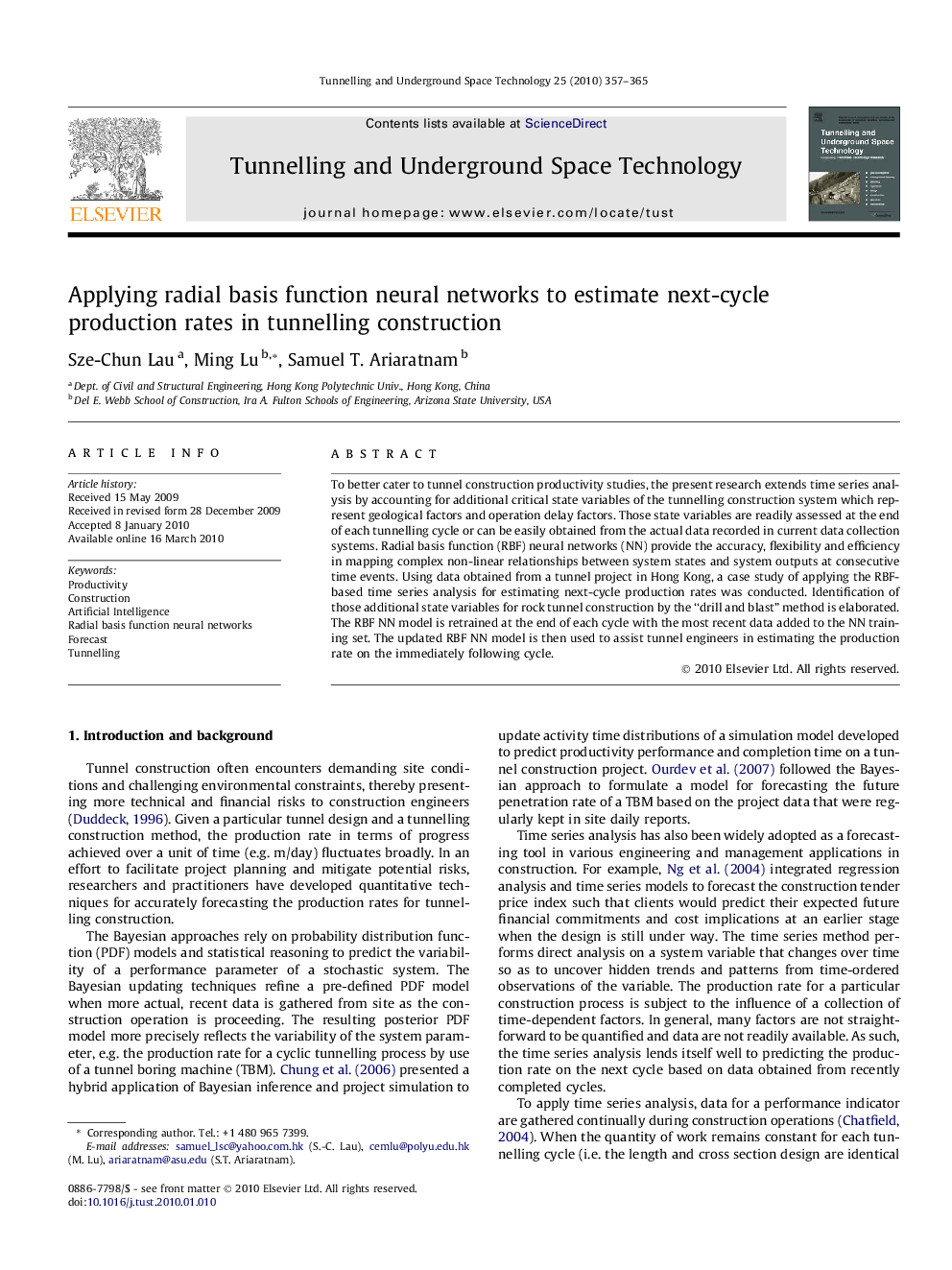
To better cater to tunnel construction productivity studies, the present research extends time series analysis by accounting for additional critical state variables of the tunnelling construction system which represent geological factors and operation delay factors. Those state variables are readily assessed at the end of each tunnelling cycle or can be easily obtained from the actual data recorded in current data collection systems. Radial basis function (RBF) neural networks (NN) provide the accuracy, flexibility and efficiency in mapping complex non-linear relationships between system states and system outputs at consecutive time events. Using data obtained from a tunnel project in Hong Kong, a case study of applying the RBF-based time series analysis for estimating next-cycle production rates was conducted. Identification of those additional state variables for rock tunnel construction by the “drill and blast” method is elaborated. The RBF NN model is retrained at the end of each cycle with the most recent data added to the NN training set. The updated RBF NN model is then used to assist tunnel engineers in estimating the production rate on the immediately following cycle.
Journal: Tunnelling and Underground Space Technology - Volume 25, Issue 4, July 2010, Pages 357–365