کد مقاله | کد نشریه | سال انتشار | مقاله انگلیسی | نسخه تمام متن |
---|---|---|---|---|
395766 | 666012 | 2006 | 24 صفحه PDF | دانلود رایگان |
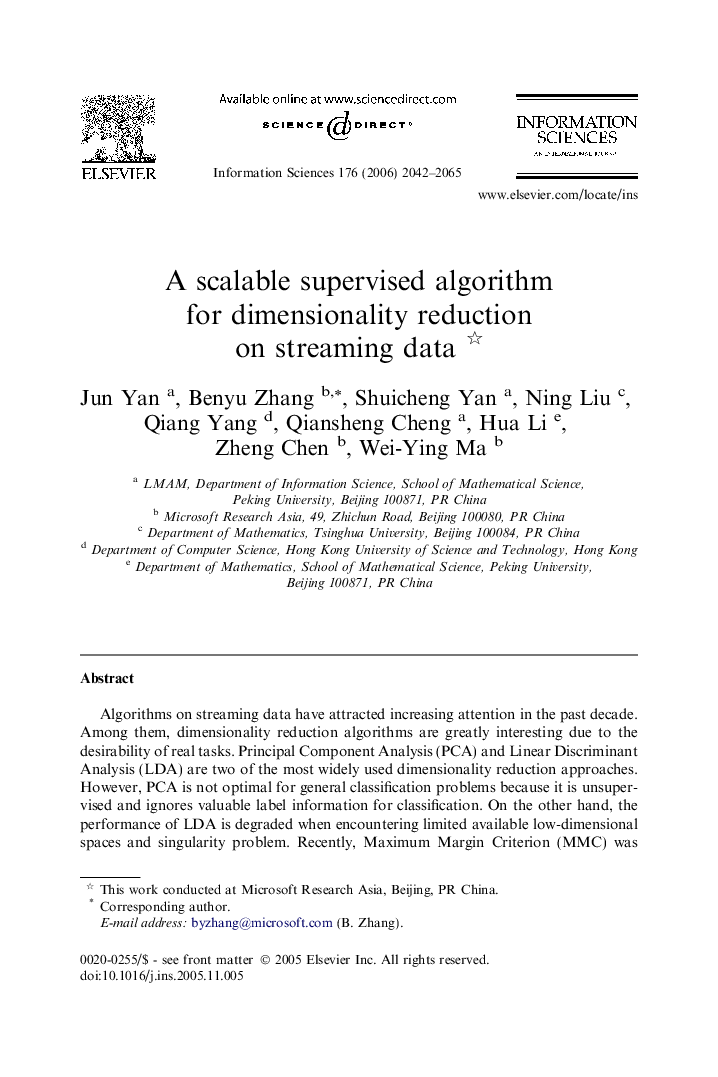
Algorithms on streaming data have attracted increasing attention in the past decade. Among them, dimensionality reduction algorithms are greatly interesting due to the desirability of real tasks. Principal Component Analysis (PCA) and Linear Discriminant Analysis (LDA) are two of the most widely used dimensionality reduction approaches. However, PCA is not optimal for general classification problems because it is unsupervised and ignores valuable label information for classification. On the other hand, the performance of LDA is degraded when encountering limited available low-dimensional spaces and singularity problem. Recently, Maximum Margin Criterion (MMC) was proposed to overcome the shortcomings of PCA and LDA. Nevertheless, the original MMC algorithm could not satisfy the streaming data model to handle large-scale high-dimensional data set. Thus an effective, efficient and scalable approach is needed. In this paper, we propose a supervised incremental dimensionality reduction algorithm and its extension to infer adaptive low-dimensional spaces by optimizing the maximum margin criterion. Experimental results on a synthetic dataset and real datasets demonstrate the superior performance of our proposed algorithm on streaming data.
Journal: Information Sciences - Volume 176, Issue 14, 22 July 2006, Pages 2042–2065