کد مقاله | کد نشریه | سال انتشار | مقاله انگلیسی | نسخه تمام متن |
---|---|---|---|---|
432415 | 688884 | 2013 | 17 صفحه PDF | دانلود رایگان |
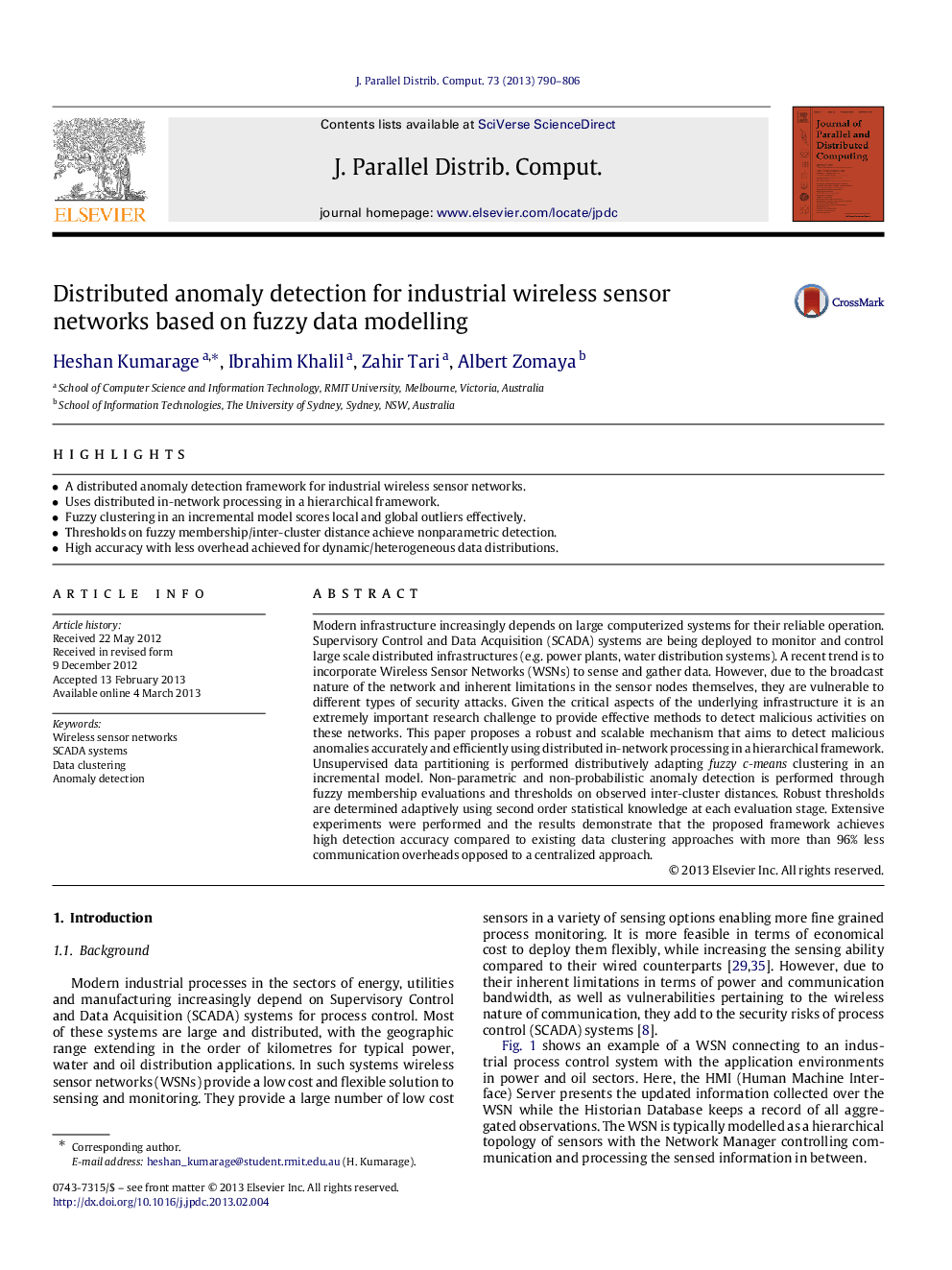
• A distributed anomaly detection framework for industrial wireless sensor networks.
• Uses distributed in-network processing in a hierarchical framework.
• Fuzzy clustering in an incremental model scores local and global outliers effectively.
• Thresholds on fuzzy membership/inter-cluster distance achieve nonparametric detection.
• High accuracy with less overhead achieved for dynamic/heterogeneous data distributions.
Modern infrastructure increasingly depends on large computerized systems for their reliable operation. Supervisory Control and Data Acquisition (SCADA) systems are being deployed to monitor and control large scale distributed infrastructures (e.g. power plants, water distribution systems). A recent trend is to incorporate Wireless Sensor Networks (WSNs) to sense and gather data. However, due to the broadcast nature of the network and inherent limitations in the sensor nodes themselves, they are vulnerable to different types of security attacks. Given the critical aspects of the underlying infrastructure it is an extremely important research challenge to provide effective methods to detect malicious activities on these networks. This paper proposes a robust and scalable mechanism that aims to detect malicious anomalies accurately and efficiently using distributed in-network processing in a hierarchical framework. Unsupervised data partitioning is performed distributively adapting fuzzy c-means clustering in an incremental model. Non-parametric and non-probabilistic anomaly detection is performed through fuzzy membership evaluations and thresholds on observed inter-cluster distances. Robust thresholds are determined adaptively using second order statistical knowledge at each evaluation stage. Extensive experiments were performed and the results demonstrate that the proposed framework achieves high detection accuracy compared to existing data clustering approaches with more than 96% less communication overheads opposed to a centralized approach.
Journal: Journal of Parallel and Distributed Computing - Volume 73, Issue 6, June 2013, Pages 790–806