کد مقاله | کد نشریه | سال انتشار | مقاله انگلیسی | نسخه تمام متن |
---|---|---|---|---|
5132157 | 1491509 | 2017 | 13 صفحه PDF | دانلود رایگان |
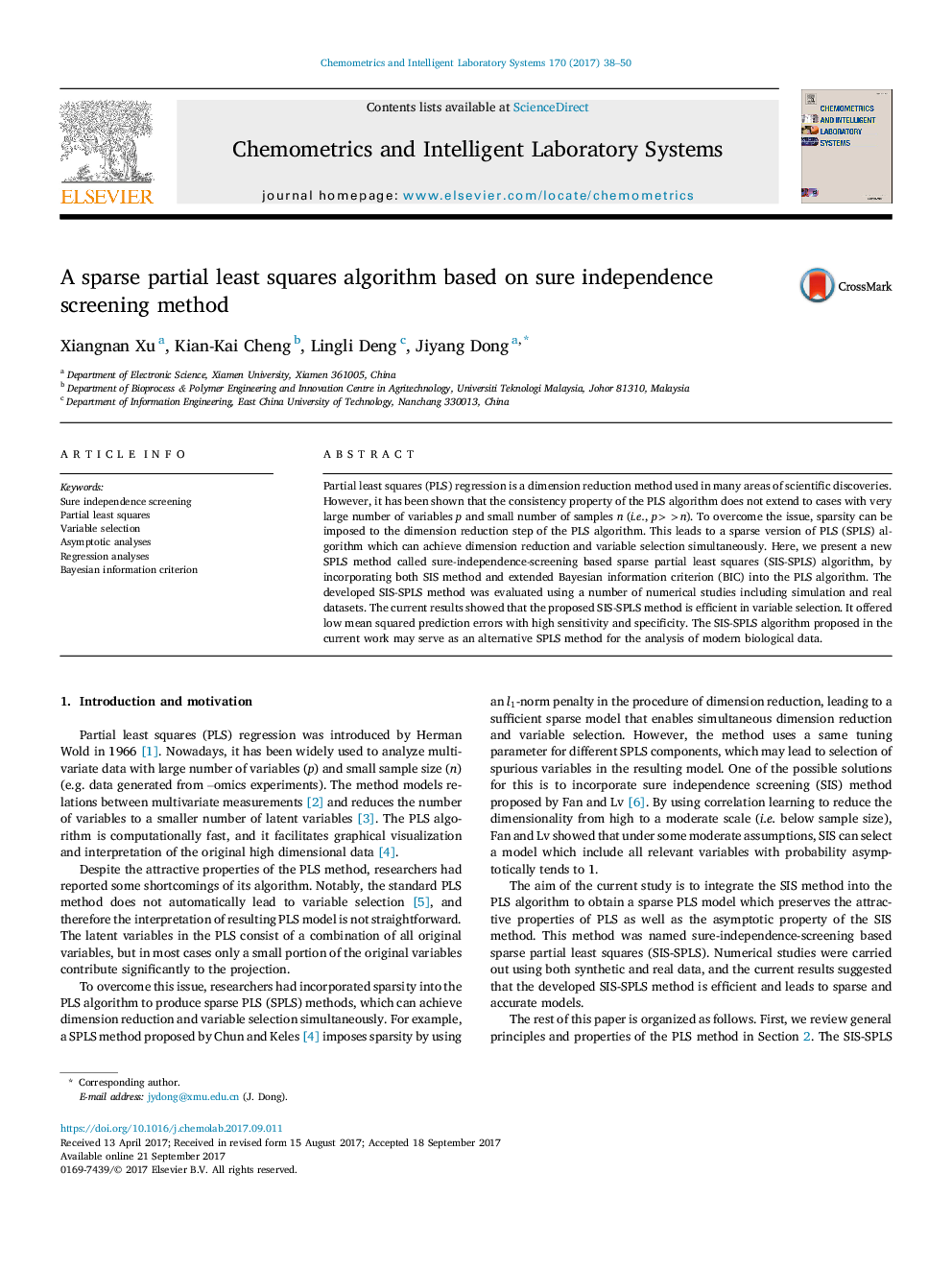
- A new sparse PLS method called sure independence screening based sparse partial least squares is proposed in this paper.
- SIS-SPLS is suitable for ill-posed problem and enjoys the properties of orthogonality in PLS and sure screening in SIS.
- SIS-SPLS is implemented by extended BIC instead of using tuning parameters as in published SPLS methods.
- SIS-SPLS outperforms the other methods on both prediction and variable selection.
Partial least squares (PLS) regression is a dimension reduction method used in many areas of scientific discoveries. However, it has been shown that the consistency property of the PLS algorithm does not extend to cases with very large number of variables p and small number of samples n (i.e., p>>n). To overcome the issue, sparsity can be imposed to the dimension reduction step of the PLS algorithm. This leads to a sparse version of PLS (SPLS) algorithm which can achieve dimension reduction and variable selection simultaneously. Here, we present a new SPLS method called sure-independence-screening based sparse partial least squares (SIS-SPLS) algorithm, by incorporating both SIS method and extended Bayesian information criterion (BIC) into the PLS algorithm. The developed SIS-SPLS method was evaluated using a number of numerical studies including simulation and real datasets. The current results showed that the proposed SIS-SPLS method is efficient in variable selection. It offered low mean squared prediction errors with high sensitivity and specificity. The SIS-SPLS algorithm proposed in the current work may serve as an alternative SPLS method for the analysis of modern biological data.
Journal: Chemometrics and Intelligent Laboratory Systems - Volume 170, 15 November 2017, Pages 38-50