کد مقاله | کد نشریه | سال انتشار | مقاله انگلیسی | نسخه تمام متن |
---|---|---|---|---|
5132181 | 1491512 | 2017 | 13 صفحه PDF | دانلود رایگان |
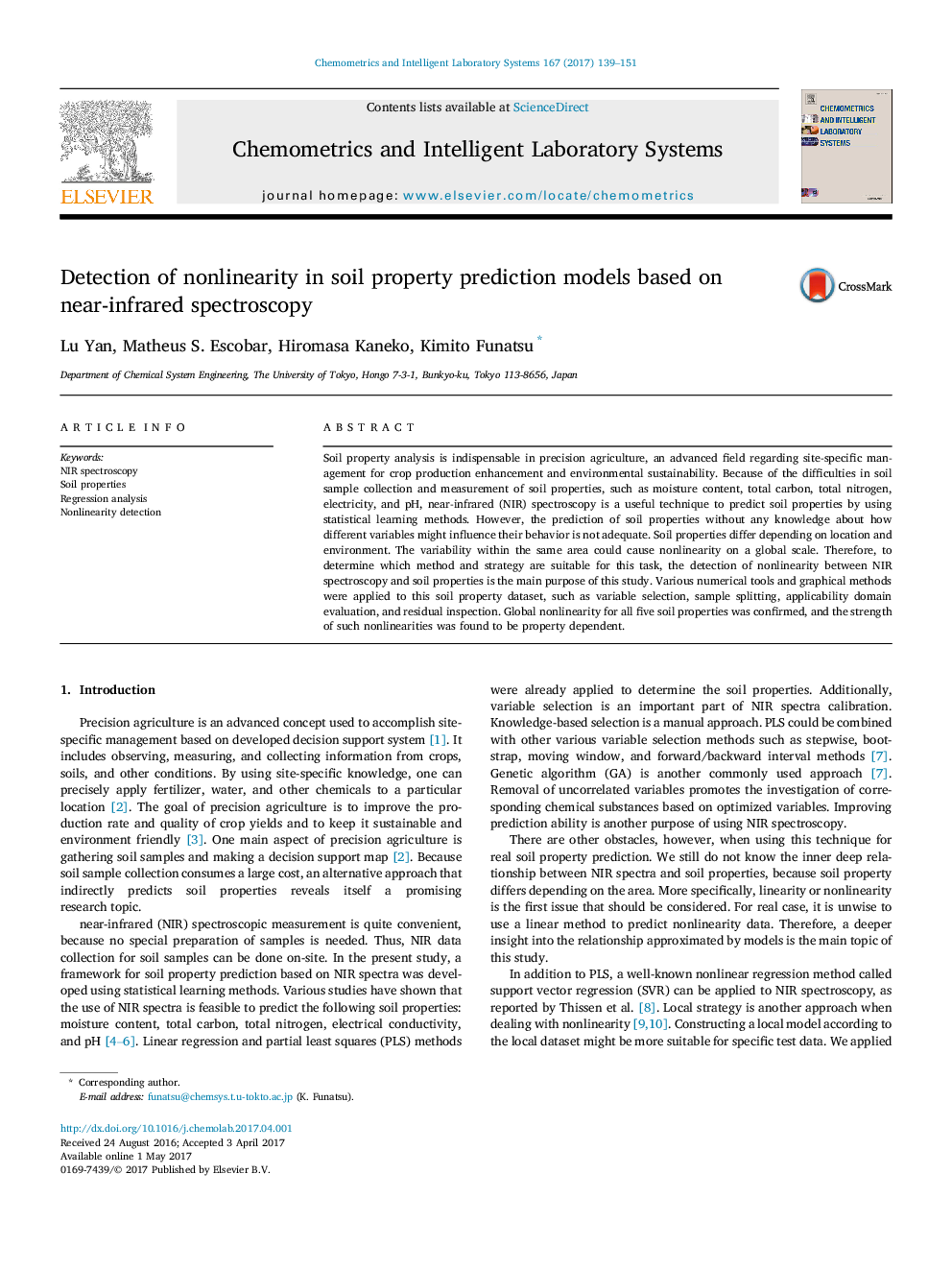
- Soil property was predicted by statistical models based on NIR-spectroscopy.
- This study focused on detecting nonlinearity between NIR and soil properties.
- Numerical tools and graphic methods were applied to this soil property dataset.
- Nonlinearity and its property dependence were confirmed for all five soil properties.
Soil property analysis is indispensable in precision agriculture, an advanced field regarding site-specific management for crop production enhancement and environmental sustainability. Because of the difficulties in soil sample collection and measurement of soil properties, such as moisture content, total carbon, total nitrogen, electricity, and pH, near-infrared (NIR) spectroscopy is a useful technique to predict soil properties by using statistical learning methods. However, the prediction of soil properties without any knowledge about how different variables might influence their behavior is not adequate. Soil properties differ depending on location and environment. The variability within the same area could cause nonlinearity on a global scale. Therefore, to determine which method and strategy are suitable for this task, the detection of nonlinearity between NIR spectroscopy and soil properties is the main purpose of this study. Various numerical tools and graphical methods were applied to this soil property dataset, such as variable selection, sample splitting, applicability domain evaluation, and residual inspection. Global nonlinearity for all five soil properties was confirmed, and the strength of such nonlinearities was found to be property dependent.
Journal: Chemometrics and Intelligent Laboratory Systems - Volume 167, 15 August 2017, Pages 139-151