کد مقاله | کد نشریه | سال انتشار | مقاله انگلیسی | نسخه تمام متن |
---|---|---|---|---|
5132244 | 1491517 | 2017 | 11 صفحه PDF | دانلود رایگان |
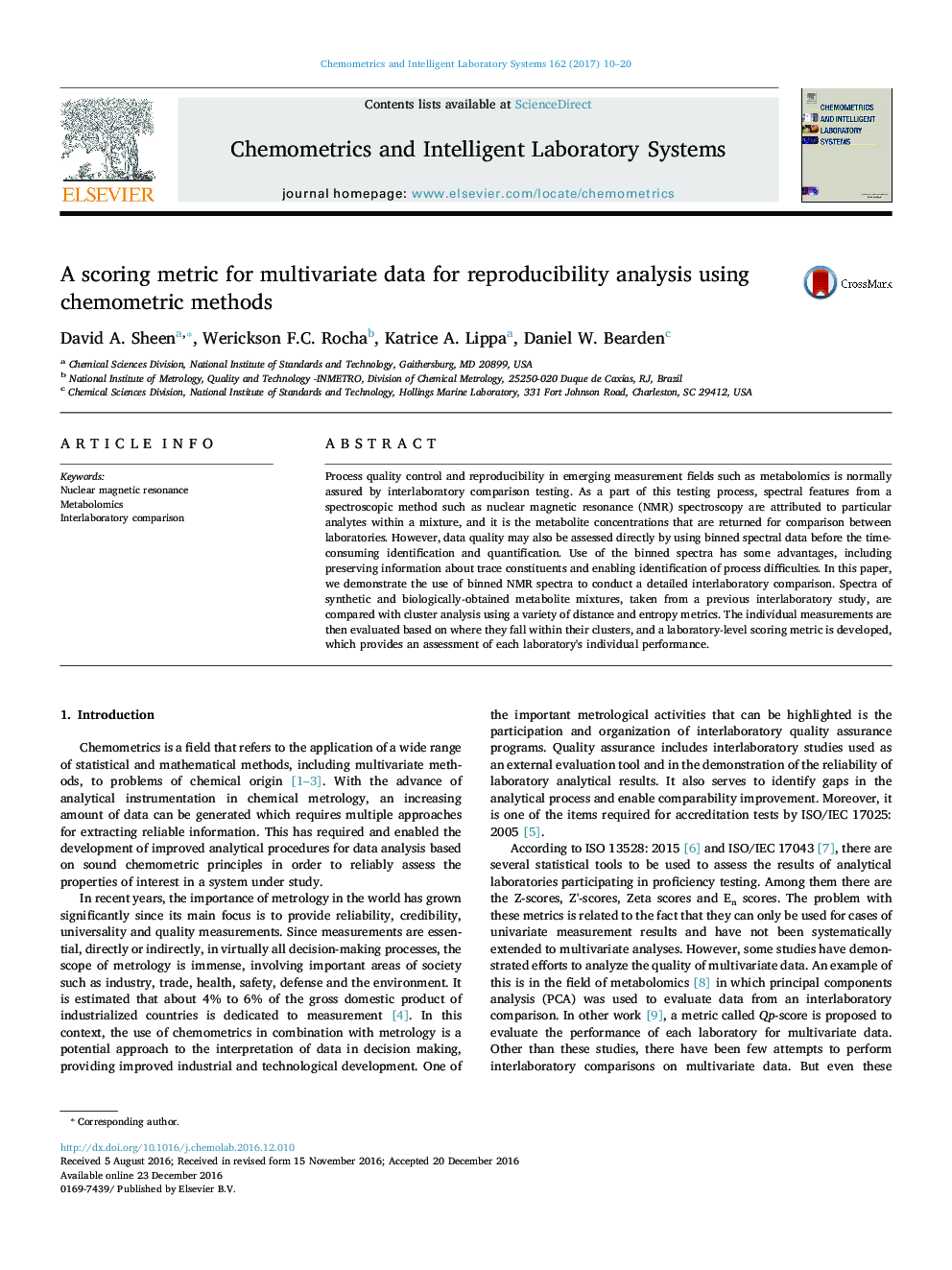
- We propose a method for scoring multivariate data in interlaboratory comparisons.
- A set of trusted labs is determined whose data are closest to consensus.
- The method is applied to a study using spectra of natural mixtures and synthetic mixtures.
- Spectral data with commonly-encountered experimental issues are consistently identified.
- The method works best if trusted labs are pre-determined using synthetic sample data.
Process quality control and reproducibility in emerging measurement fields such as metabolomics is normally assured by interlaboratory comparison testing. As a part of this testing process, spectral features from a spectroscopic method such as nuclear magnetic resonance (NMR) spectroscopy are attributed to particular analytes within a mixture, and it is the metabolite concentrations that are returned for comparison between laboratories. However, data quality may also be assessed directly by using binned spectral data before the time-consuming identification and quantification. Use of the binned spectra has some advantages, including preserving information about trace constituents and enabling identification of process difficulties. In this paper, we demonstrate the use of binned NMR spectra to conduct a detailed interlaboratory comparison. Spectra of synthetic and biologically-obtained metabolite mixtures, taken from a previous interlaboratory study, are compared with cluster analysis using a variety of distance and entropy metrics. The individual measurements are then evaluated based on where they fall within their clusters, and a laboratory-level scoring metric is developed, which provides an assessment of each laboratory's individual performance.
Journal: Chemometrics and Intelligent Laboratory Systems - Volume 162, 15 March 2017, Pages 10-20