کد مقاله | کد نشریه | سال انتشار | مقاله انگلیسی | نسخه تمام متن |
---|---|---|---|---|
5132259 | 1491517 | 2017 | 12 صفحه PDF | دانلود رایگان |
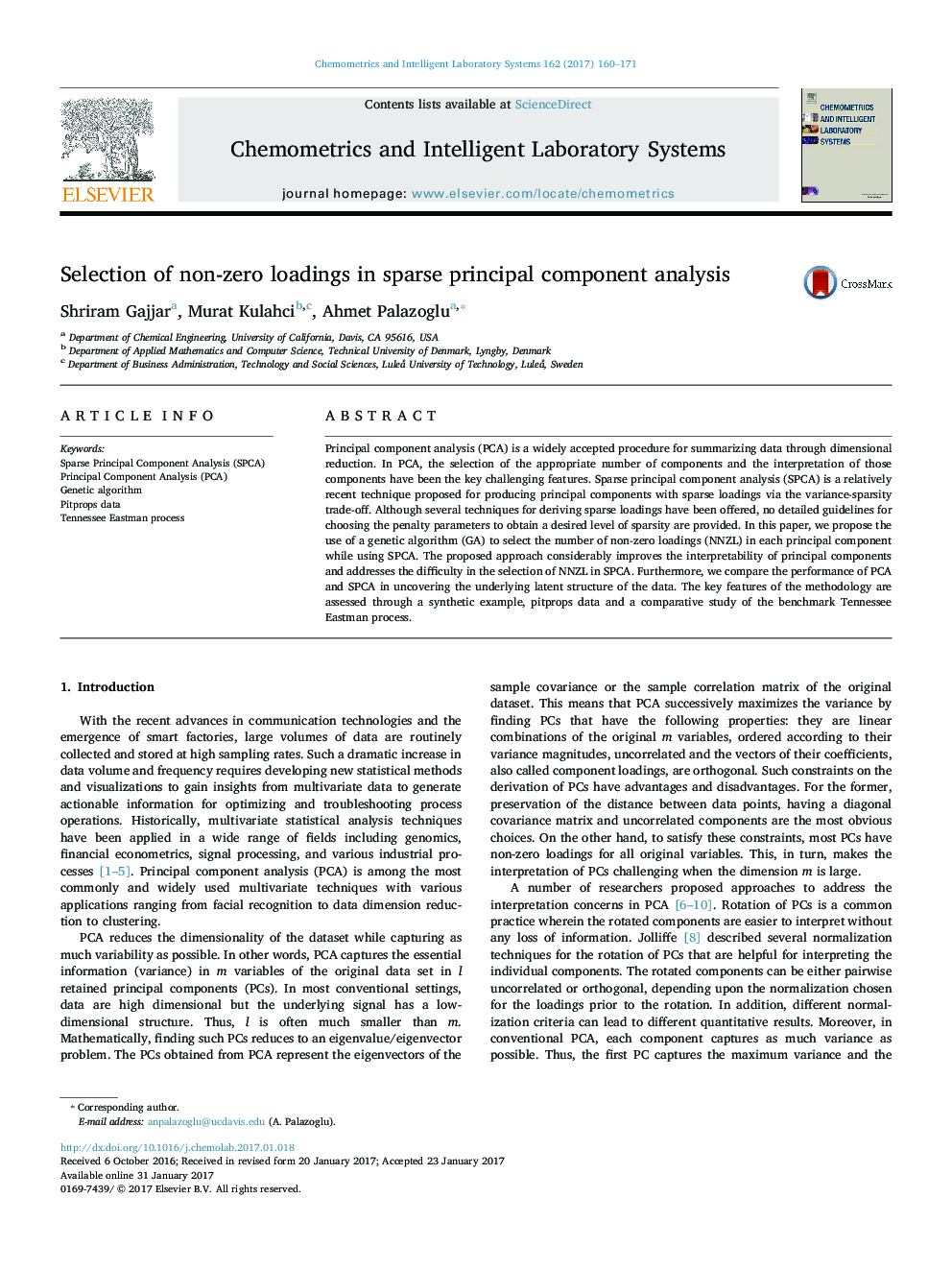
Principal component analysis (PCA) is a widely accepted procedure for summarizing data through dimensional reduction. In PCA, the selection of the appropriate number of components and the interpretation of those components have been the key challenging features. Sparse principal component analysis (SPCA) is a relatively recent technique proposed for producing principal components with sparse loadings via the variance-sparsity trade-off. Although several techniques for deriving sparse loadings have been offered, no detailed guidelines for choosing the penalty parameters to obtain a desired level of sparsity are provided. In this paper, we propose the use of a genetic algorithm (GA) to select the number of non-zero loadings (NNZL) in each principal component while using SPCA. The proposed approach considerably improves the interpretability of principal components and addresses the difficulty in the selection of NNZL in SPCA. Furthermore, we compare the performance of PCA and SPCA in uncovering the underlying latent structure of the data. The key features of the methodology are assessed through a synthetic example, pitprops data and a comparative study of the benchmark Tennessee Eastman process.
Journal: Chemometrics and Intelligent Laboratory Systems - Volume 162, 15 March 2017, Pages 160-171