کد مقاله | کد نشریه | سال انتشار | مقاله انگلیسی | نسخه تمام متن |
---|---|---|---|---|
5822153 | 1557837 | 2014 | 8 صفحه PDF | دانلود رایگان |
عنوان انگلیسی مقاله ISI
Structure-based predictors of resistance to the HIV-1 integrase inhibitor Elvitegravir
دانلود مقاله + سفارش ترجمه
دانلود مقاله ISI انگلیسی
رایگان برای ایرانیان
کلمات کلیدی
موضوعات مرتبط
علوم زیستی و بیوفناوری
ایمنی شناسی و میکروب شناسی
ویروس شناسی
پیش نمایش صفحه اول مقاله
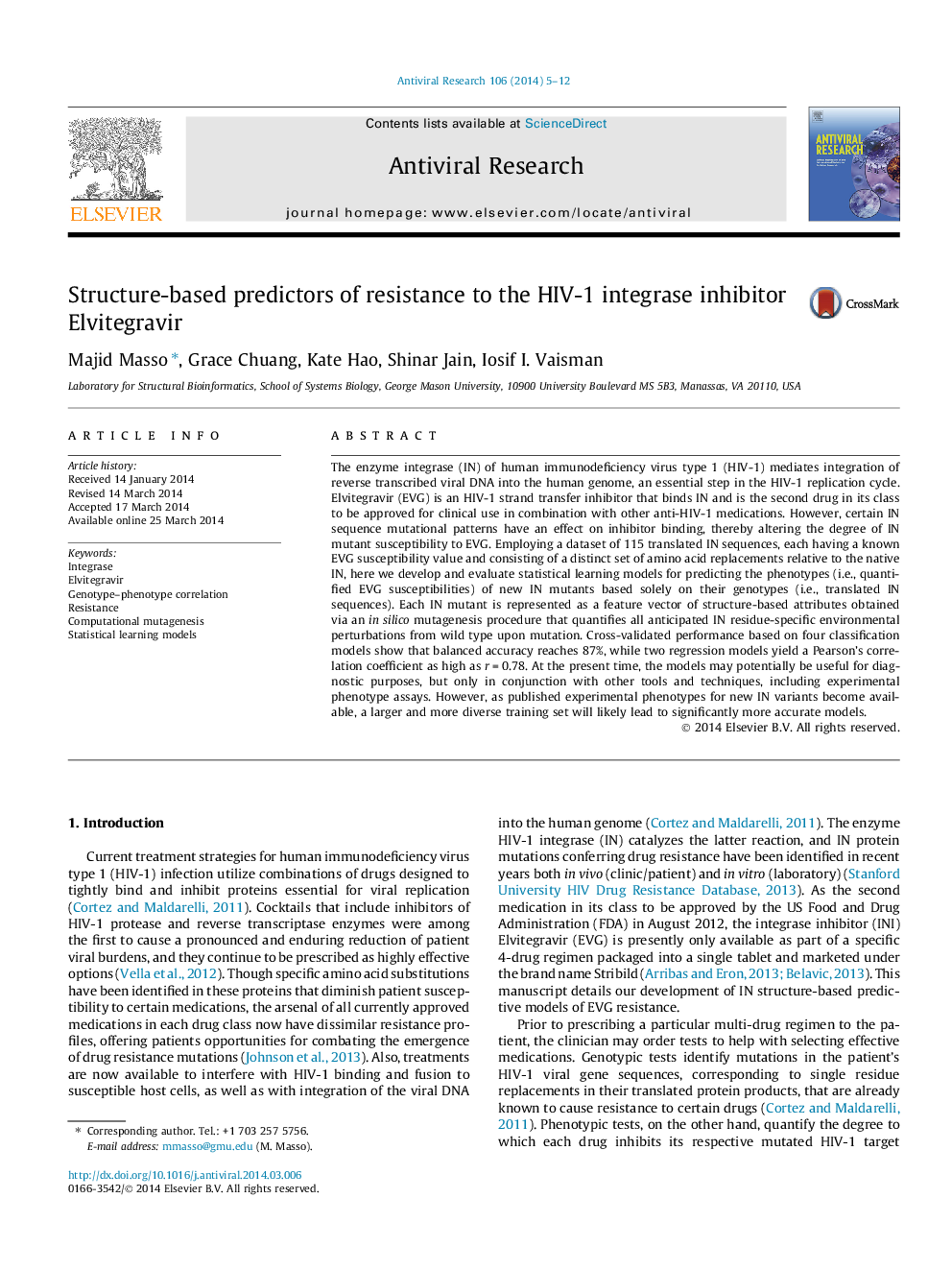
چکیده انگلیسی
The enzyme integrase (IN) of human immunodeficiency virus type 1 (HIV-1) mediates integration of reverse transcribed viral DNA into the human genome, an essential step in the HIV-1 replication cycle. Elvitegravir (EVG) is an HIV-1 strand transfer inhibitor that binds IN and is the second drug in its class to be approved for clinical use in combination with other anti-HIV-1 medications. However, certain IN sequence mutational patterns have an effect on inhibitor binding, thereby altering the degree of IN mutant susceptibility to EVG. Employing a dataset of 115 translated IN sequences, each having a known EVG susceptibility value and consisting of a distinct set of amino acid replacements relative to the native IN, here we develop and evaluate statistical learning models for predicting the phenotypes (i.e., quantified EVG susceptibilities) of new IN mutants based solely on their genotypes (i.e., translated IN sequences). Each IN mutant is represented as a feature vector of structure-based attributes obtained via an in silico mutagenesis procedure that quantifies all anticipated IN residue-specific environmental perturbations from wild type upon mutation. Cross-validated performance based on four classification models show that balanced accuracy reaches 87%, while two regression models yield a Pearson's correlation coefficient as high as r = 0.78. At the present time, the models may potentially be useful for diagnostic purposes, but only in conjunction with other tools and techniques, including experimental phenotype assays. However, as published experimental phenotypes for new IN variants become available, a larger and more diverse training set will likely lead to significantly more accurate models.
ناشر
Database: Elsevier - ScienceDirect (ساینس دایرکت)
Journal: Antiviral Research - Volume 106, June 2014, Pages 5-12
Journal: Antiviral Research - Volume 106, June 2014, Pages 5-12
نویسندگان
Majid Masso, Grace Chuang, Kate Hao, Shinar Jain, Iosif I. Vaisman,