کد مقاله | کد نشریه | سال انتشار | مقاله انگلیسی | نسخه تمام متن |
---|---|---|---|---|
87751 | 159265 | 2012 | 10 صفحه PDF | دانلود رایگان |
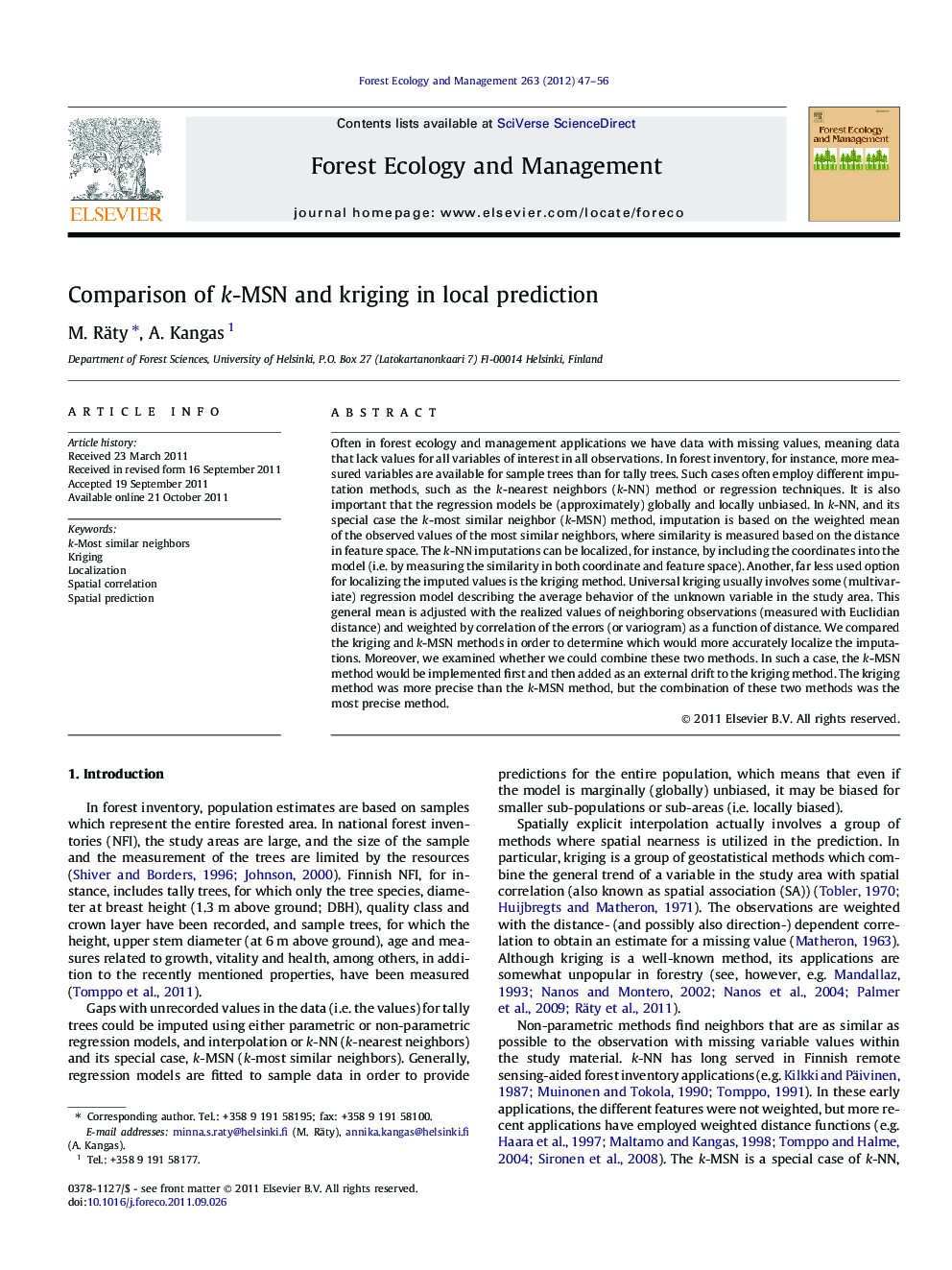
Often in forest ecology and management applications we have data with missing values, meaning data that lack values for all variables of interest in all observations. In forest inventory, for instance, more measured variables are available for sample trees than for tally trees. Such cases often employ different imputation methods, such as the k-nearest neighbors (k-NN) method or regression techniques. It is also important that the regression models be (approximately) globally and locally unbiased. In k-NN, and its special case the k-most similar neighbor (k-MSN) method, imputation is based on the weighted mean of the observed values of the most similar neighbors, where similarity is measured based on the distance in feature space. The k-NN imputations can be localized, for instance, by including the coordinates into the model (i.e. by measuring the similarity in both coordinate and feature space). Another, far less used option for localizing the imputed values is the kriging method. Universal kriging usually involves some (multivariate) regression model describing the average behavior of the unknown variable in the study area. This general mean is adjusted with the realized values of neighboring observations (measured with Euclidian distance) and weighted by correlation of the errors (or variogram) as a function of distance. We compared the kriging and k-MSN methods in order to determine which would more accurately localize the imputations. Moreover, we examined whether we could combine these two methods. In such a case, the k-MSN method would be implemented first and then added as an external drift to the kriging method. The kriging method was more precise than the k-MSN method, but the combination of these two methods was the most precise method.
Journal: Forest Ecology and Management - Volume 263, 1 January 2012, Pages 47–56