کد مقاله | کد نشریه | سال انتشار | مقاله انگلیسی | نسخه تمام متن |
---|---|---|---|---|
10537847 | 962851 | 2005 | 13 صفحه PDF | دانلود رایگان |
عنوان انگلیسی مقاله ISI
Multivariate data analysis in classification of vegetable oils characterized by the content of fatty acids
دانلود مقاله + سفارش ترجمه
دانلود مقاله ISI انگلیسی
رایگان برای ایرانیان
کلمات کلیدی
موضوعات مرتبط
مهندسی و علوم پایه
شیمی
شیمی آنالیزی یا شیمی تجزیه
پیش نمایش صفحه اول مقاله
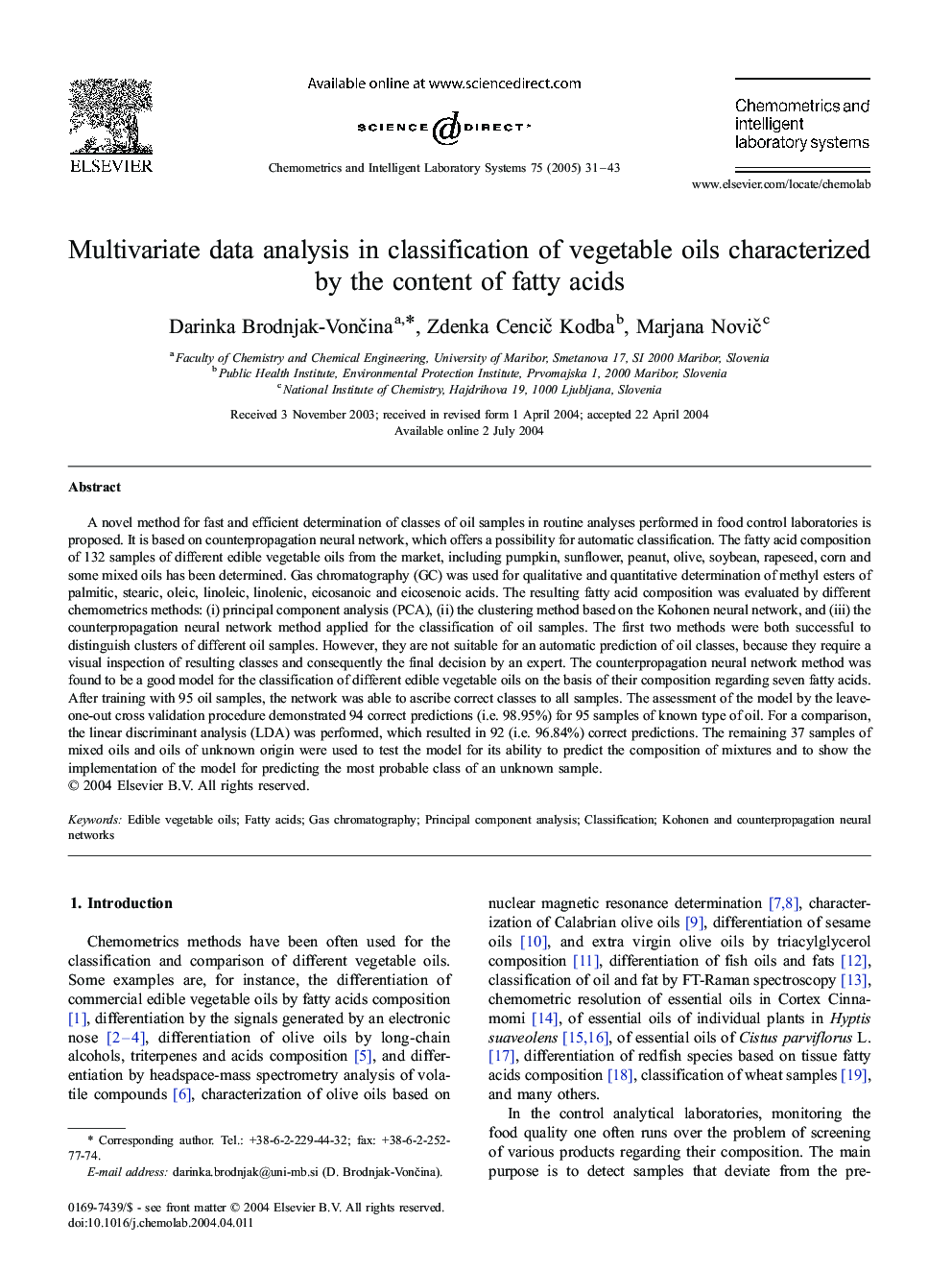
چکیده انگلیسی
A novel method for fast and efficient determination of classes of oil samples in routine analyses performed in food control laboratories is proposed. It is based on counterpropagation neural network, which offers a possibility for automatic classification. The fatty acid composition of 132 samples of different edible vegetable oils from the market, including pumpkin, sunflower, peanut, olive, soybean, rapeseed, corn and some mixed oils has been determined. Gas chromatography (GC) was used for qualitative and quantitative determination of methyl esters of palmitic, stearic, oleic, linoleic, linolenic, eicosanoic and eicosenoic acids. The resulting fatty acid composition was evaluated by different chemometrics methods: (i) principal component analysis (PCA), (ii) the clustering method based on the Kohonen neural network, and (iii) the counterpropagation neural network method applied for the classification of oil samples. The first two methods were both successful to distinguish clusters of different oil samples. However, they are not suitable for an automatic prediction of oil classes, because they require a visual inspection of resulting classes and consequently the final decision by an expert. The counterpropagation neural network method was found to be a good model for the classification of different edible vegetable oils on the basis of their composition regarding seven fatty acids. After training with 95 oil samples, the network was able to ascribe correct classes to all samples. The assessment of the model by the leave-one-out cross validation procedure demonstrated 94 correct predictions (i.e. 98.95%) for 95 samples of known type of oil. For a comparison, the linear discriminant analysis (LDA) was performed, which resulted in 92 (i.e. 96.84%) correct predictions. The remaining 37 samples of mixed oils and oils of unknown origin were used to test the model for its ability to predict the composition of mixtures and to show the implementation of the model for predicting the most probable class of an unknown sample.
ناشر
Database: Elsevier - ScienceDirect (ساینس دایرکت)
Journal: Chemometrics and Intelligent Laboratory Systems - Volume 75, Issue 1, 28 January 2005, Pages 31-43
Journal: Chemometrics and Intelligent Laboratory Systems - Volume 75, Issue 1, 28 January 2005, Pages 31-43
نویسندگان
Darinka Brodnjak-VonÄina, Zdenka CenciÄ Kodba, Marjana NoviÄ,