کد مقاله | کد نشریه | سال انتشار | مقاله انگلیسی | نسخه تمام متن |
---|---|---|---|---|
1181017 | 1491550 | 2013 | 12 صفحه PDF | دانلود رایگان |
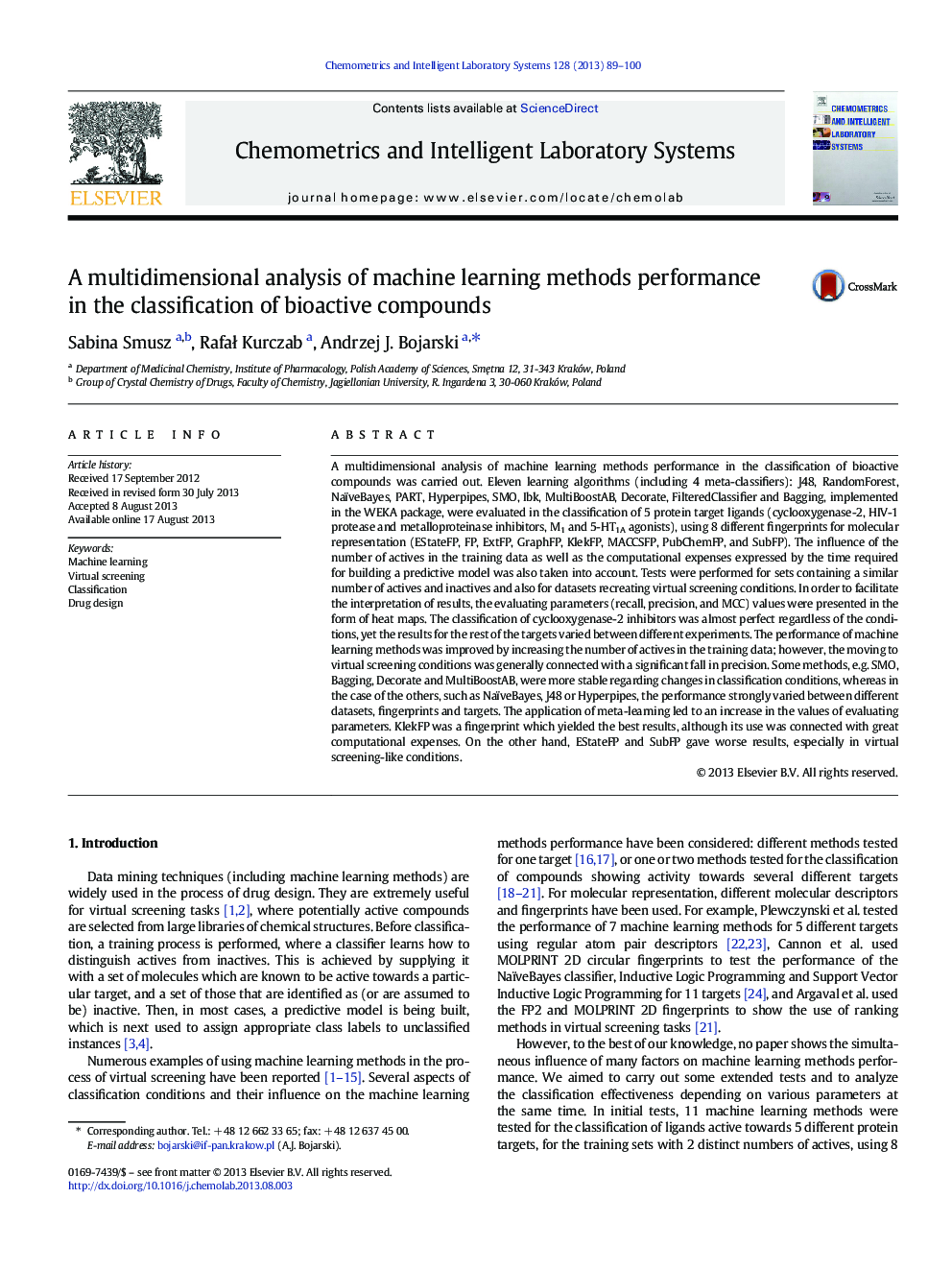
• Presents a multidimensional analysis of machine learning methods performance
• Explores the influence of various parameters
• Provides some useful tips for people using machine learning methods
A multidimensional analysis of machine learning methods performance in the classification of bioactive compounds was carried out. Eleven learning algorithms (including 4 meta-classifiers): J48, RandomForest, NaïveBayes, PART, Hyperpipes, SMO, Ibk, MultiBoostAB, Decorate, FilteredClassifier and Bagging, implemented in the WEKA package, were evaluated in the classification of 5 protein target ligands (cyclooxygenase-2, HIV-1 protease and metalloproteinase inhibitors, M1 and 5-HT1A agonists), using 8 different fingerprints for molecular representation (EStateFP, FP, ExtFP, GraphFP, KlekFP, MACCSFP, PubChemFP, and SubFP). The influence of the number of actives in the training data as well as the computational expenses expressed by the time required for building a predictive model was also taken into account. Tests were performed for sets containing a similar number of actives and inactives and also for datasets recreating virtual screening conditions. In order to facilitate the interpretation of results, the evaluating parameters (recall, precision, and MCC) values were presented in the form of heat maps. The classification of cyclooxygenase-2 inhibitors was almost perfect regardless of the conditions, yet the results for the rest of the targets varied between different experiments. The performance of machine learning methods was improved by increasing the number of actives in the training data; however, the moving to virtual screening conditions was generally connected with a significant fall in precision. Some methods, e.g. SMO, Bagging, Decorate and MultiBoostAB, were more stable regarding changes in classification conditions, whereas in the case of the others, such as NaïveBayes, J48 or Hyperpipes, the performance strongly varied between different datasets, fingerprints and targets. The application of meta-learning led to an increase in the values of evaluating parameters. KlekFP was a fingerprint which yielded the best results, although its use was connected with great computational expenses. On the other hand, EStateFP and SubFP gave worse results, especially in virtual screening-like conditions.
Figure optionsDownload as PowerPoint slide
Journal: Chemometrics and Intelligent Laboratory Systems - Volume 128, 15 October 2013, Pages 89–100