کد مقاله | کد نشریه | سال انتشار | مقاله انگلیسی | نسخه تمام متن |
---|---|---|---|---|
1181501 | 1491564 | 2012 | 10 صفحه PDF | دانلود رایگان |
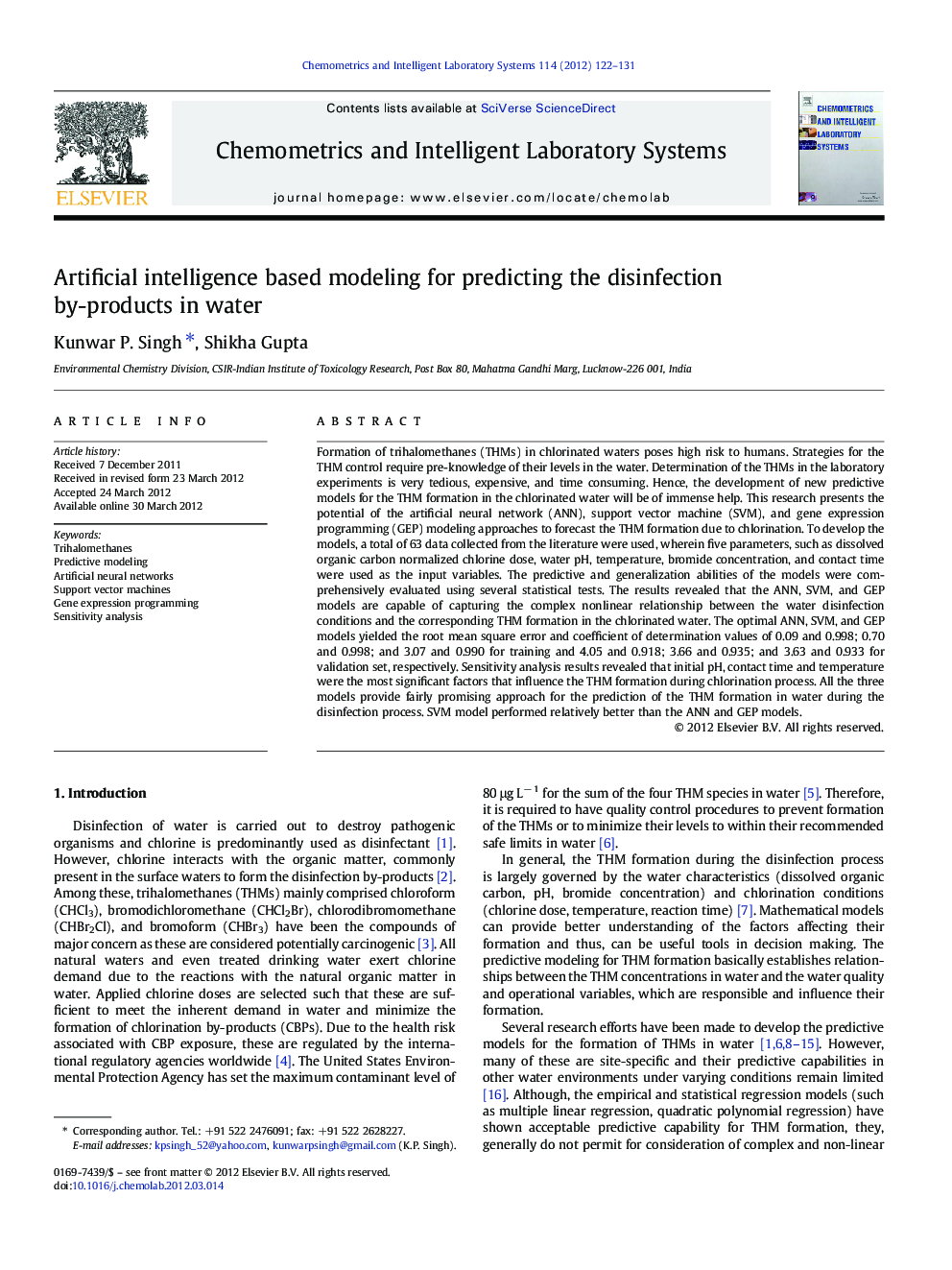
Formation of trihalomethanes (THMs) in chlorinated waters poses high risk to humans. Strategies for the THM control require pre-knowledge of their levels in the water. Determination of the THMs in the laboratory experiments is very tedious, expensive, and time consuming. Hence, the development of new predictive models for the THM formation in the chlorinated water will be of immense help. This research presents the potential of the artificial neural network (ANN), support vector machine (SVM), and gene expression programming (GEP) modeling approaches to forecast the THM formation due to chlorination. To develop the models, a total of 63 data collected from the literature were used, wherein five parameters, such as dissolved organic carbon normalized chlorine dose, water pH, temperature, bromide concentration, and contact time were used as the input variables. The predictive and generalization abilities of the models were comprehensively evaluated using several statistical tests. The results revealed that the ANN, SVM, and GEP models are capable of capturing the complex nonlinear relationship between the water disinfection conditions and the corresponding THM formation in the chlorinated water. The optimal ANN, SVM, and GEP models yielded the root mean square error and coefficient of determination values of 0.09 and 0.998; 0.70 and 0.998; and 3.07 and 0.990 for training and 4.05 and 0.918; 3.66 and 0.935; and 3.63 and 0.933 for validation set, respectively. Sensitivity analysis results revealed that initial pH, contact time and temperature were the most significant factors that influence the THM formation during chlorination process. All the three models provide fairly promising approach for the prediction of the THM formation in water during the disinfection process. SVM model performed relatively better than the ANN and GEP models.
► Artificial intelligence based models constructed for predicting DBPs in water.
► ANN, SVM, and GEP modeling approaches were used.
► All the three models exhibited comparable excellent prediction abilities (r>0.91).
► Steiger’s Z-test revealed relatively better performance of SVM than other models.
► All the five predictor variables contributed almost to same extent in three models.
Journal: Chemometrics and Intelligent Laboratory Systems - Volume 114, 15 May 2012, Pages 122–131