کد مقاله | کد نشریه | سال انتشار | مقاله انگلیسی | نسخه تمام متن |
---|---|---|---|---|
4482008 | 1316845 | 2013 | 9 صفحه PDF | دانلود رایگان |
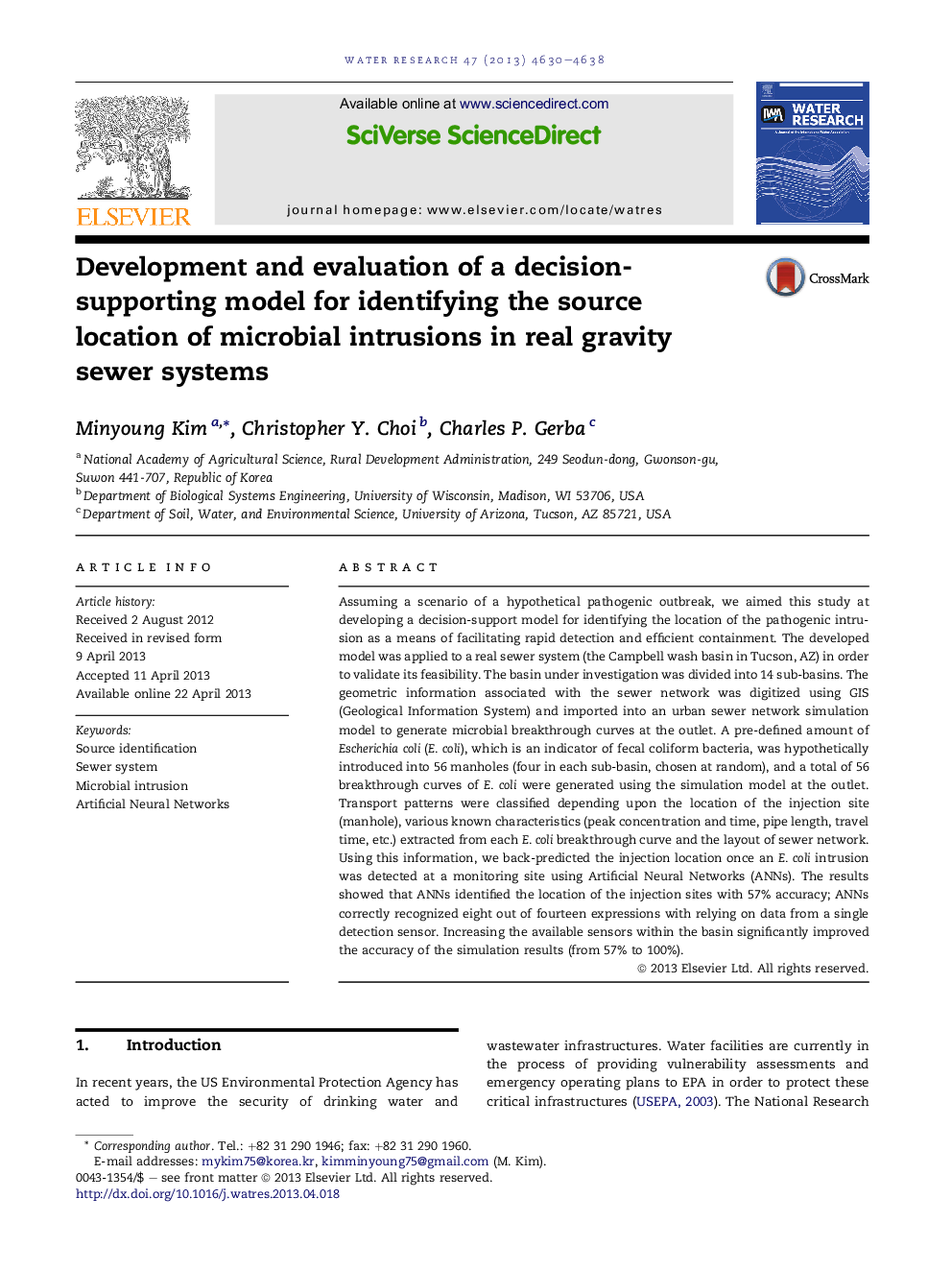
• Accurate prediction of microbial fate and transport.
• Establishment of decision-support technique to track microbial source location.
• Application of developed the technique into the real sewer system.
Assuming a scenario of a hypothetical pathogenic outbreak, we aimed this study at developing a decision-support model for identifying the location of the pathogenic intrusion as a means of facilitating rapid detection and efficient containment. The developed model was applied to a real sewer system (the Campbell wash basin in Tucson, AZ) in order to validate its feasibility. The basin under investigation was divided into 14 sub-basins. The geometric information associated with the sewer network was digitized using GIS (Geological Information System) and imported into an urban sewer network simulation model to generate microbial breakthrough curves at the outlet. A pre-defined amount of Escherichia coli (E. coli), which is an indicator of fecal coliform bacteria, was hypothetically introduced into 56 manholes (four in each sub-basin, chosen at random), and a total of 56 breakthrough curves of E. coli were generated using the simulation model at the outlet. Transport patterns were classified depending upon the location of the injection site (manhole), various known characteristics (peak concentration and time, pipe length, travel time, etc.) extracted from each E. coli breakthrough curve and the layout of sewer network. Using this information, we back-predicted the injection location once an E. coli intrusion was detected at a monitoring site using Artificial Neural Networks (ANNs). The results showed that ANNs identified the location of the injection sites with 57% accuracy; ANNs correctly recognized eight out of fourteen expressions with relying on data from a single detection sensor. Increasing the available sensors within the basin significantly improved the accuracy of the simulation results (from 57% to 100%).
Figure optionsDownload high-quality image (82 K)Download as PowerPoint slide
Journal: Water Research - Volume 47, Issue 13, 1 September 2013, Pages 4630–4638