کد مقاله | کد نشریه | سال انتشار | مقاله انگلیسی | نسخه تمام متن |
---|---|---|---|---|
4576473 | 1629967 | 2013 | 13 صفحه PDF | دانلود رایگان |
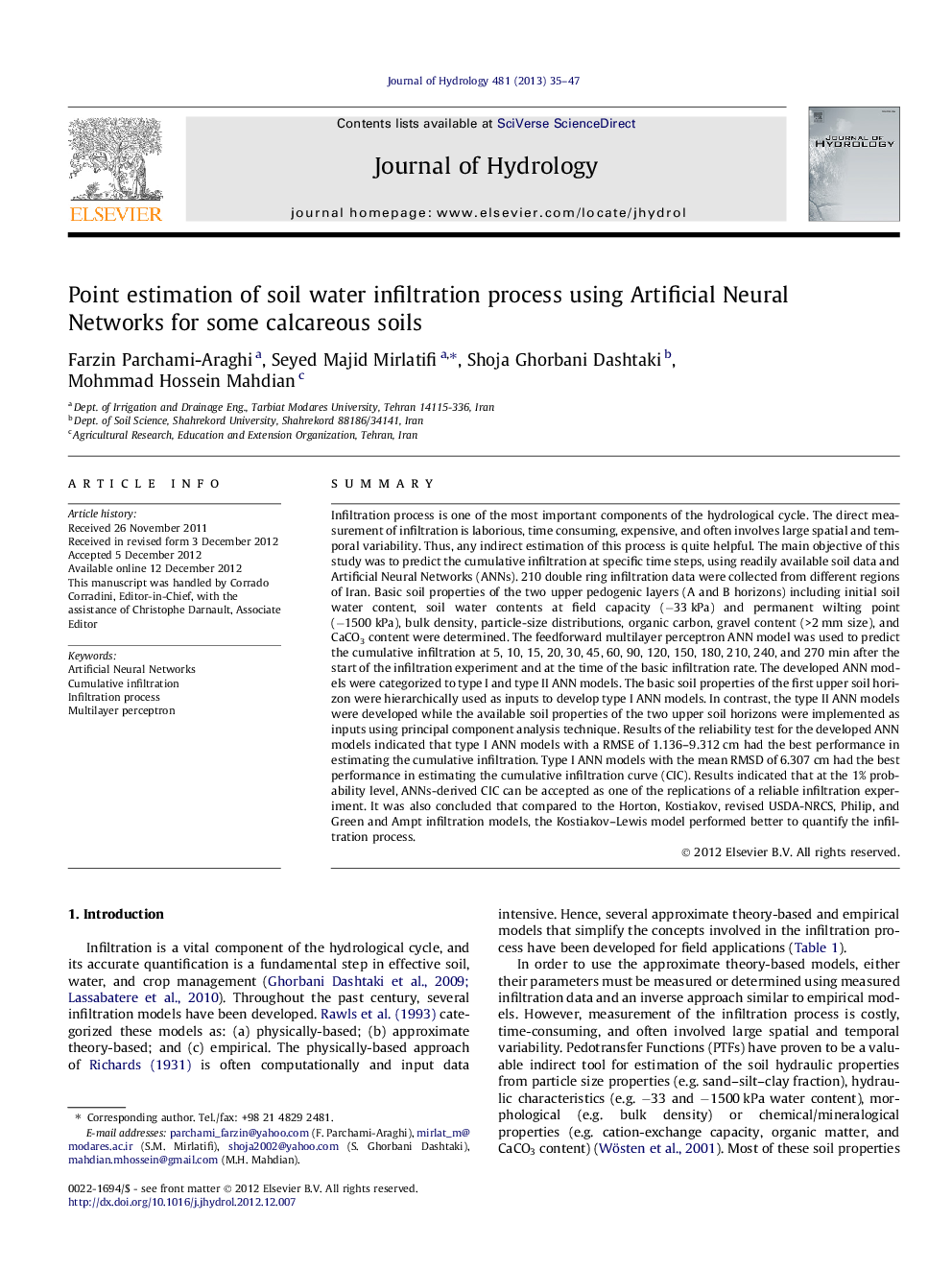
SummaryInfiltration process is one of the most important components of the hydrological cycle. The direct measurement of infiltration is laborious, time consuming, expensive, and often involves large spatial and temporal variability. Thus, any indirect estimation of this process is quite helpful. The main objective of this study was to predict the cumulative infiltration at specific time steps, using readily available soil data and Artificial Neural Networks (ANNs). 210 double ring infiltration data were collected from different regions of Iran. Basic soil properties of the two upper pedogenic layers (A and B horizons) including initial soil water content, soil water contents at field capacity (−33 kPa) and permanent wilting point (−1500 kPa), bulk density, particle-size distributions, organic carbon, gravel content (>2 mm size), and CaCO3 content were determined. The feedforward multilayer perceptron ANN model was used to predict the cumulative infiltration at 5, 10, 15, 20, 30, 45, 60, 90, 120, 150, 180, 210, 240, and 270 min after the start of the infiltration experiment and at the time of the basic infiltration rate. The developed ANN models were categorized to type I and type II ANN models. The basic soil properties of the first upper soil horizon were hierarchically used as inputs to develop type I ANN models. In contrast, the type II ANN models were developed while the available soil properties of the two upper soil horizons were implemented as inputs using principal component analysis technique. Results of the reliability test for the developed ANN models indicated that type I ANN models with a RMSE of 1.136–9.312 cm had the best performance in estimating the cumulative infiltration. Type I ANN models with the mean RMSD of 6.307 cm had the best performance in estimating the cumulative infiltration curve (CIC). Results indicated that at the 1% probability level, ANNs-derived CIC can be accepted as one of the replications of a reliable infiltration experiment. It was also concluded that compared to the Horton, Kostiakov, revised USDA-NRCS, Philip, and Green and Ampt infiltration models, the Kostiakov–Lewis model performed better to quantify the infiltration process.
► Performance of developed ANN models improved through the infiltration time.
► Cumulative infiltration curve, I(t), was estimated with the mean RMSD of 6.307 cm.
► The estimated I(t) can be accepted as a reliable infiltration test result (p < 0.01).
Journal: Journal of Hydrology - Volume 481, 25 February 2013, Pages 35–47