کد مقاله | کد نشریه | سال انتشار | مقاله انگلیسی | نسخه تمام متن |
---|---|---|---|---|
4577669 | 1630015 | 2011 | 16 صفحه PDF | دانلود رایگان |
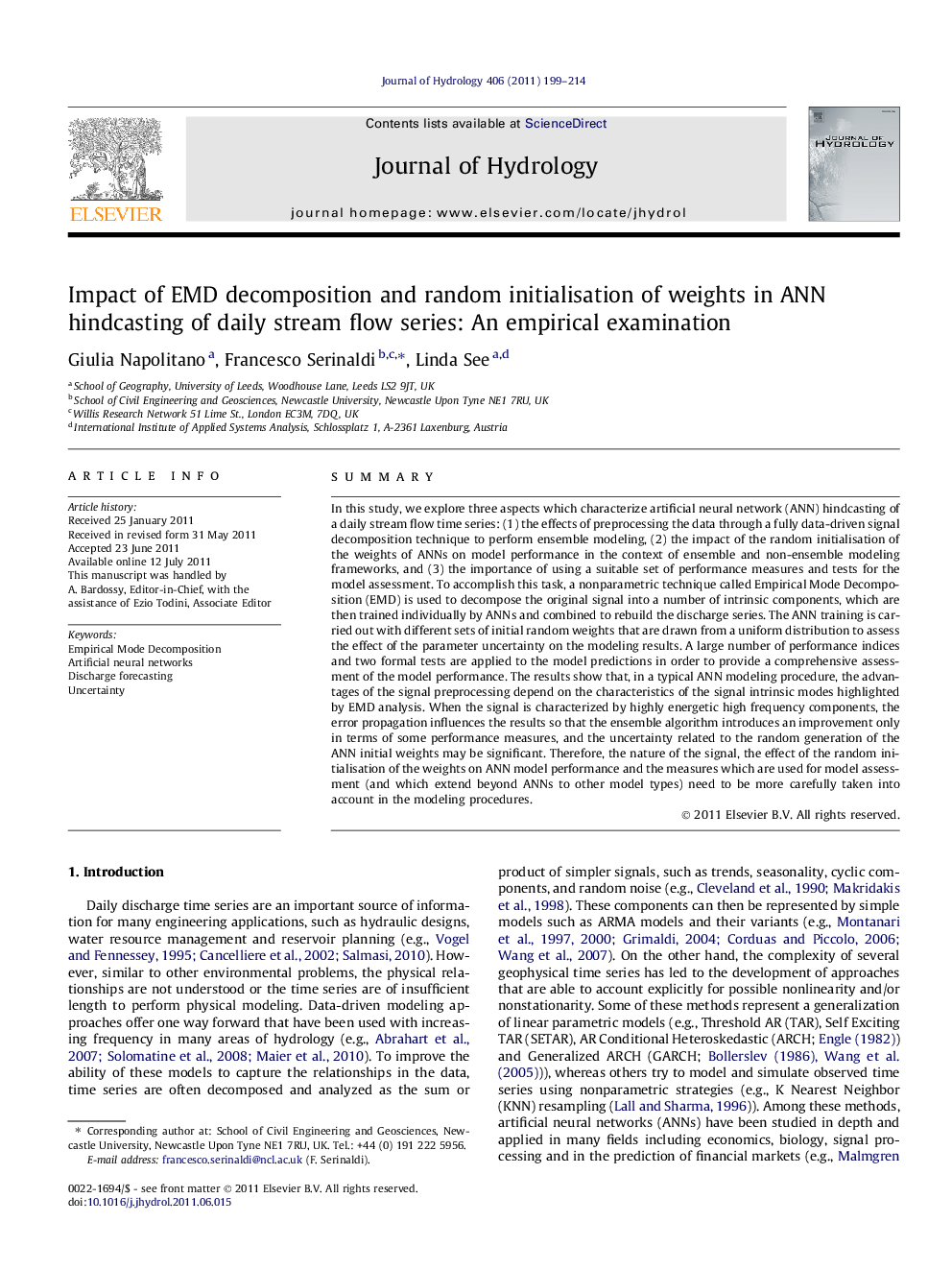
SummaryIn this study, we explore three aspects which characterize artificial neural network (ANN) hindcasting of a daily stream flow time series: (1) the effects of preprocessing the data through a fully data-driven signal decomposition technique to perform ensemble modeling, (2) the impact of the random initialisation of the weights of ANNs on model performance in the context of ensemble and non-ensemble modeling frameworks, and (3) the importance of using a suitable set of performance measures and tests for the model assessment. To accomplish this task, a nonparametric technique called Empirical Mode Decomposition (EMD) is used to decompose the original signal into a number of intrinsic components, which are then trained individually by ANNs and combined to rebuild the discharge series. The ANN training is carried out with different sets of initial random weights that are drawn from a uniform distribution to assess the effect of the parameter uncertainty on the modeling results. A large number of performance indices and two formal tests are applied to the model predictions in order to provide a comprehensive assessment of the model performance. The results show that, in a typical ANN modeling procedure, the advantages of the signal preprocessing depend on the characteristics of the signal intrinsic modes highlighted by EMD analysis. When the signal is characterized by highly energetic high frequency components, the error propagation influences the results so that the ensemble algorithm introduces an improvement only in terms of some performance measures, and the uncertainty related to the random generation of the ANN initial weights may be significant. Therefore, the nature of the signal, the effect of the random initialisation of the weights on ANN model performance and the measures which are used for model assessment (and which extend beyond ANNs to other model types) need to be more carefully taken into account in the modeling procedures.
► We explore three aspects of the ANN modeling.
► (1) The decomposition of the original signal by the Empirical Mode Decomposition (EMD).
► (2) The initialisation of the random weights in the ANN training.
► (3) The choice of a proper set of performance measures.
► The results depend on the signal intrinsic modes, ANN initialisation, and measures.
Journal: Journal of Hydrology - Volume 406, Issues 3–4, 6 September 2011, Pages 199–214