کد مقاله | کد نشریه | سال انتشار | مقاله انگلیسی | نسخه تمام متن |
---|---|---|---|---|
6410819 | 1332885 | 2015 | 16 صفحه PDF | دانلود رایگان |
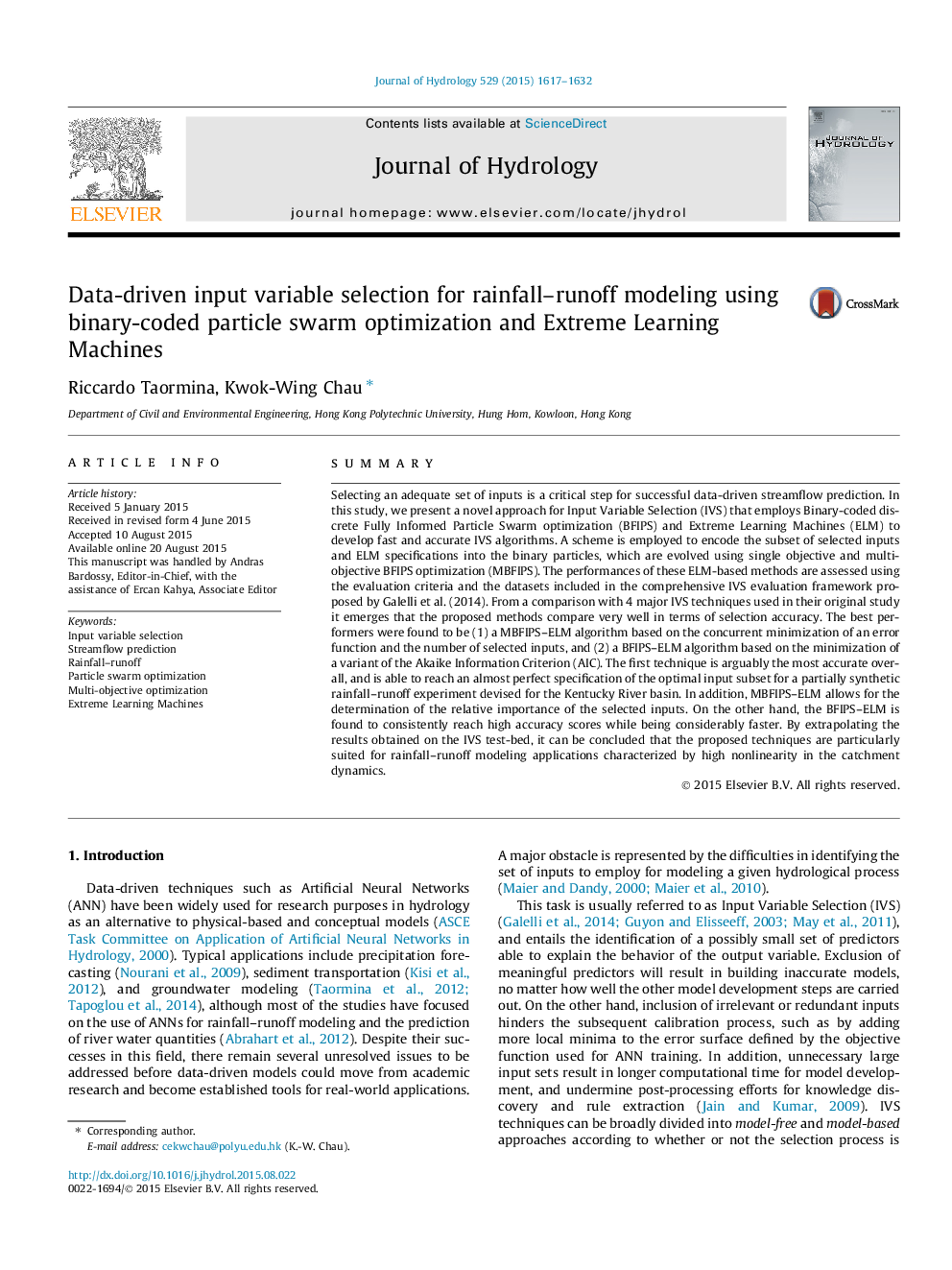
- Novel input variable selection techniques for hydrological modeling are presented.
- They employ single- and multi-objective binary PSO with Extreme Learning Machines.
- The methods concurrently optimize the structure of the underlying data-driven model.
- The methods perform very well when tested on a comprehensive evaluation framework.
- The methods outperform existing techniques on a streamflow forecasting case study.
SummarySelecting an adequate set of inputs is a critical step for successful data-driven streamflow prediction. In this study, we present a novel approach for Input Variable Selection (IVS) that employs Binary-coded discrete Fully Informed Particle Swarm optimization (BFIPS) and Extreme Learning Machines (ELM) to develop fast and accurate IVS algorithms. A scheme is employed to encode the subset of selected inputs and ELM specifications into the binary particles, which are evolved using single objective and multi-objective BFIPS optimization (MBFIPS). The performances of these ELM-based methods are assessed using the evaluation criteria and the datasets included in the comprehensive IVS evaluation framework proposed by Galelli et al. (2014). From a comparison with 4 major IVS techniques used in their original study it emerges that the proposed methods compare very well in terms of selection accuracy. The best performers were found to be (1) a MBFIPS-ELM algorithm based on the concurrent minimization of an error function and the number of selected inputs, and (2) a BFIPS-ELM algorithm based on the minimization of a variant of the Akaike Information Criterion (AIC). The first technique is arguably the most accurate overall, and is able to reach an almost perfect specification of the optimal input subset for a partially synthetic rainfall-runoff experiment devised for the Kentucky River basin. In addition, MBFIPS-ELM allows for the determination of the relative importance of the selected inputs. On the other hand, the BFIPS-ELM is found to consistently reach high accuracy scores while being considerably faster. By extrapolating the results obtained on the IVS test-bed, it can be concluded that the proposed techniques are particularly suited for rainfall-runoff modeling applications characterized by high nonlinearity in the catchment dynamics.
Journal: Journal of Hydrology - Volume 529, Part 3, October 2015, Pages 1617-1632