کد مقاله | کد نشریه | سال انتشار | مقاله انگلیسی | نسخه تمام متن |
---|---|---|---|---|
8142878 | 1523920 | 2016 | 8 صفحه PDF | دانلود رایگان |
عنوان انگلیسی مقاله ISI
Support Vector Machine combined with Distance Correlation learning for Dst forecasting during intense geomagnetic storms
دانلود مقاله + سفارش ترجمه
دانلود مقاله ISI انگلیسی
رایگان برای ایرانیان
کلمات کلیدی
موضوعات مرتبط
مهندسی و علوم پایه
علوم زمین و سیارات
فیزیک زمین (ژئو فیزیک)
پیش نمایش صفحه اول مقاله
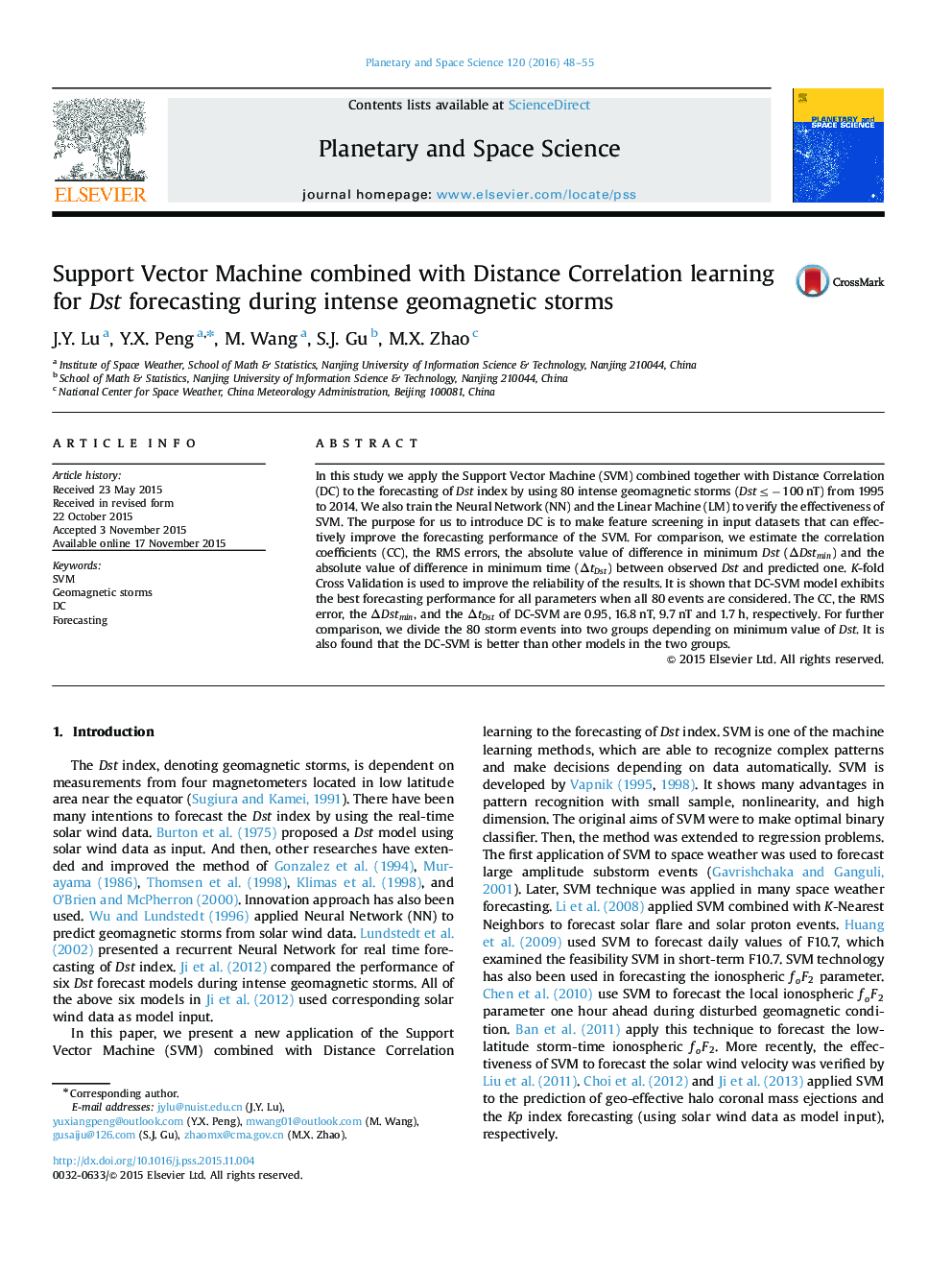
چکیده انگلیسی
In this study we apply the Support Vector Machine (SVM) combined together with Distance Correlation (DC) to the forecasting of Dst index by using 80 intense geomagnetic storms (Dstâ¤â100nT) from 1995 to 2014. We also train the Neural Network (NN) and the Linear Machine (LM) to verify the effectiveness of SVM. The purpose for us to introduce DC is to make feature screening in input datasets that can effectively improve the forecasting performance of the SVM. For comparison, we estimate the correlation coefficients (CC), the RMS errors, the absolute value of difference in minimum Dst (ÎDstmin) and the absolute value of difference in minimum time (ÎtDst) between observed Dst and predicted one. K-fold Cross Validation is used to improve the reliability of the results. It is shown that DC-SVM model exhibits the best forecasting performance for all parameters when all 80 events are considered. The CC, the RMS error, the ÎDstmin, and the ÎtDst of DC-SVM are 0.95, 16.8 nT, 9.7 nT and 1.7 h, respectively. For further comparison, we divide the 80 storm events into two groups depending on minimum value of Dst. It is also found that the DC-SVM is better than other models in the two groups.
ناشر
Database: Elsevier - ScienceDirect (ساینس دایرکت)
Journal: Planetary and Space Science - Volume 120, January 2016, Pages 48-55
Journal: Planetary and Space Science - Volume 120, January 2016, Pages 48-55
نویسندگان
J.Y. Lu, Y.X. Peng, M. Wang, S.J. Gu, M.X. Zhao,