کد مقاله | کد نشریه | سال انتشار | مقاله انگلیسی | نسخه تمام متن |
---|---|---|---|---|
4697048 | 1637233 | 2015 | 12 صفحه PDF | دانلود رایگان |
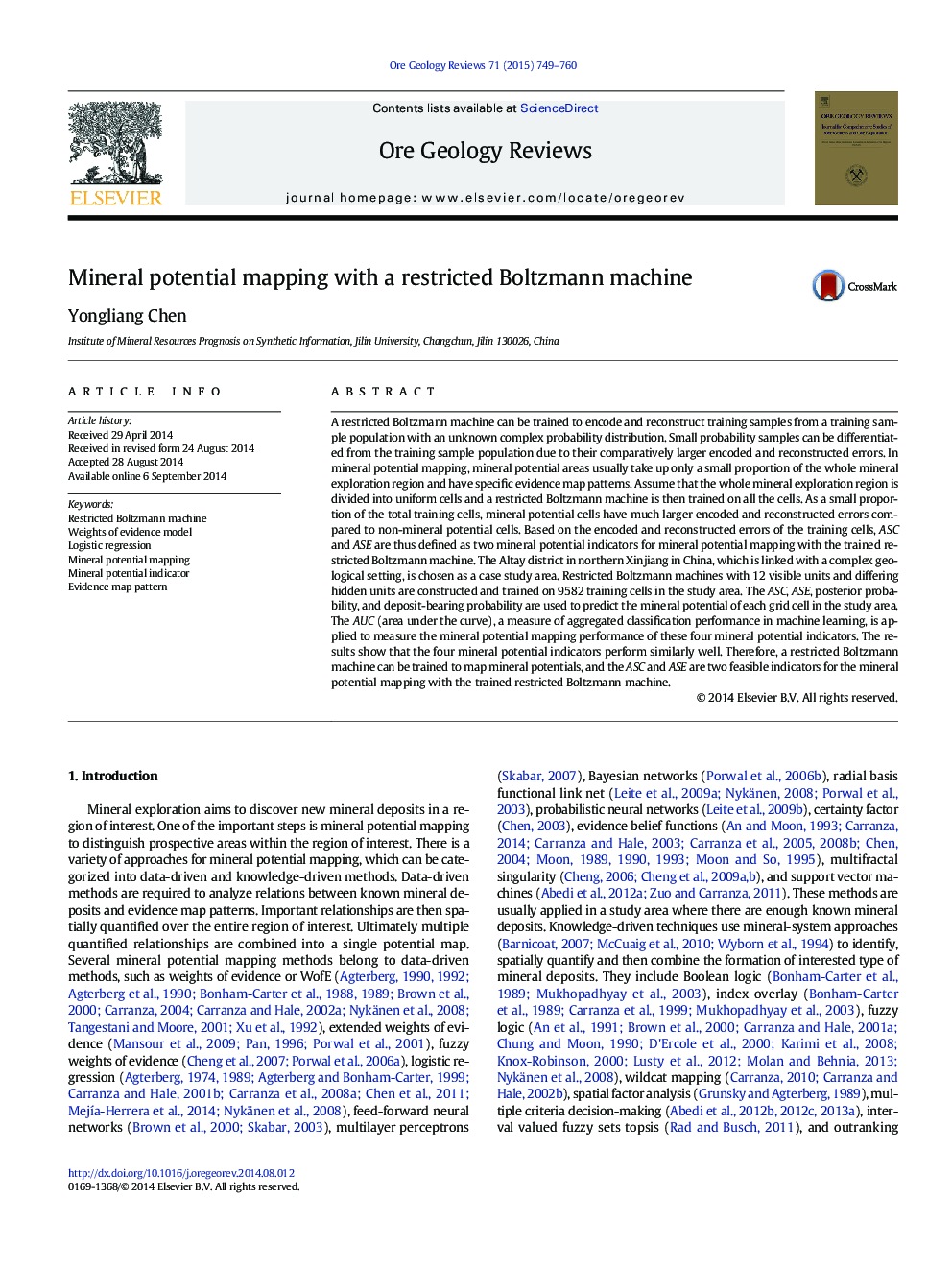
• A RBM-based method is proposed for prospectivity mapping.
• ASC and ASE are defined as mineral potential indicators based on a RBM.
• Prospectivity mapping performance is measured using AUC statistics.
• Separation of geochemical anomalies from background is optimized using the YI.
A restricted Boltzmann machine can be trained to encode and reconstruct training samples from a training sample population with an unknown complex probability distribution. Small probability samples can be differentiated from the training sample population due to their comparatively larger encoded and reconstructed errors. In mineral potential mapping, mineral potential areas usually take up only a small proportion of the whole mineral exploration region and have specific evidence map patterns. Assume that the whole mineral exploration region is divided into uniform cells and a restricted Boltzmann machine is then trained on all the cells. As a small proportion of the total training cells, mineral potential cells have much larger encoded and reconstructed errors compared to non-mineral potential cells. Based on the encoded and reconstructed errors of the training cells, ASC and ASE are thus defined as two mineral potential indicators for mineral potential mapping with the trained restricted Boltzmann machine. The Altay district in northern Xinjiang in China, which is linked with a complex geological setting, is chosen as a case study area. Restricted Boltzmann machines with 12 visible units and differing hidden units are constructed and trained on 9582 training cells in the study area. The ASC, ASE, posterior probability, and deposit-bearing probability are used to predict the mineral potential of each grid cell in the study area. The AUC (area under the curve), a measure of aggregated classification performance in machine learning, is applied to measure the mineral potential mapping performance of these four mineral potential indicators. The results show that the four mineral potential indicators perform similarly well. Therefore, a restricted Boltzmann machine can be trained to map mineral potentials, and the ASC and ASE are two feasible indicators for the mineral potential mapping with the trained restricted Boltzmann machine.
Journal: Ore Geology Reviews - Volume 71, December 2015, Pages 749–760