کد مقاله | کد نشریه | سال انتشار | مقاله انگلیسی | نسخه تمام متن |
---|---|---|---|---|
9491508 | 1630190 | 2005 | 15 صفحه PDF | دانلود رایگان |
عنوان انگلیسی مقاله ISI
Input determination for neural network models in water resources applications. Part 2. Case study: forecasting salinity in a river
دانلود مقاله + سفارش ترجمه
دانلود مقاله ISI انگلیسی
رایگان برای ایرانیان
موضوعات مرتبط
مهندسی و علوم پایه
علوم زمین و سیارات
فرآیندهای سطح زمین
پیش نمایش صفحه اول مقاله
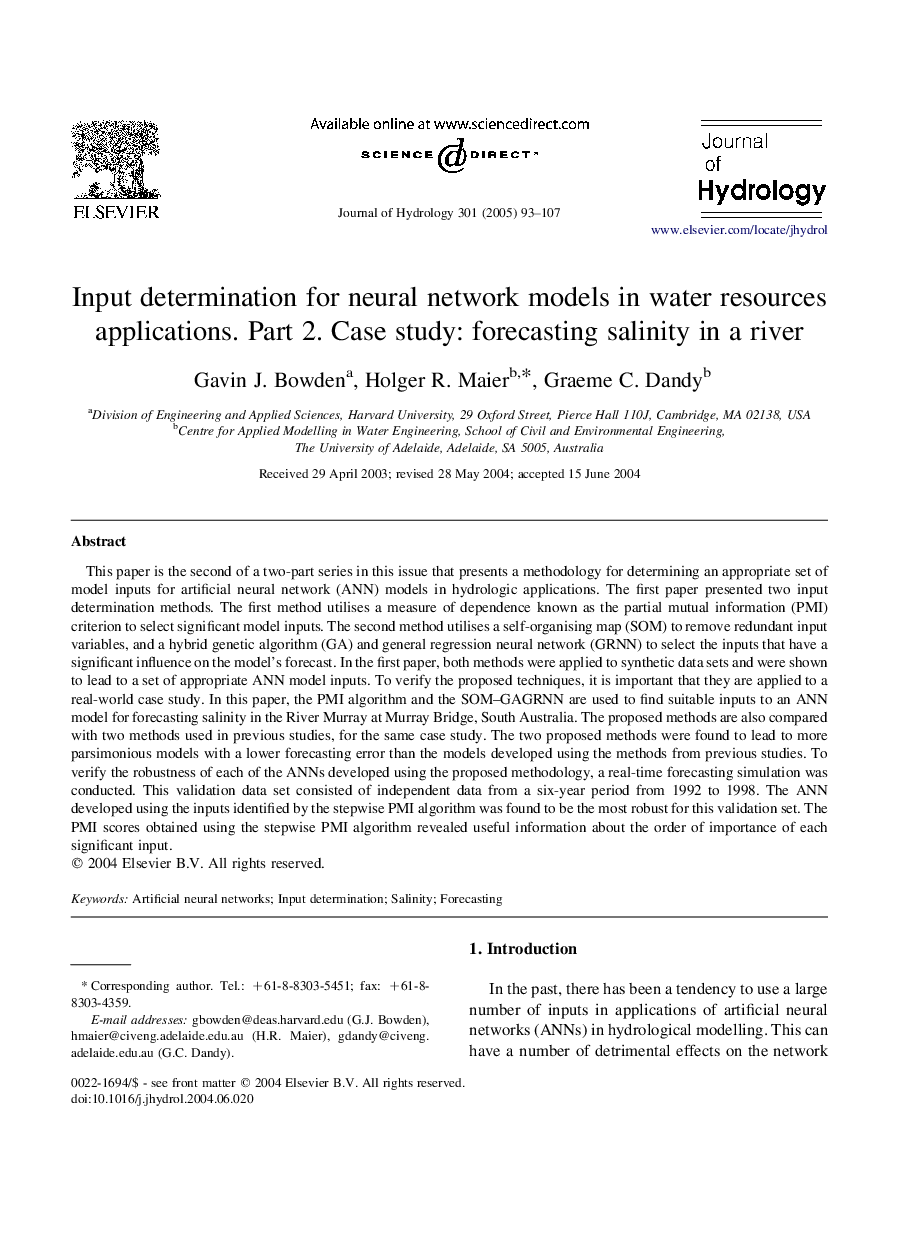
چکیده انگلیسی
This paper is the second of a two-part series in this issue that presents a methodology for determining an appropriate set of model inputs for artificial neural network (ANN) models in hydrologic applications. The first paper presented two input determination methods. The first method utilises a measure of dependence known as the partial mutual information (PMI) criterion to select significant model inputs. The second method utilises a self-organising map (SOM) to remove redundant input variables, and a hybrid genetic algorithm (GA) and general regression neural network (GRNN) to select the inputs that have a significant influence on the model's forecast. In the first paper, both methods were applied to synthetic data sets and were shown to lead to a set of appropriate ANN model inputs. To verify the proposed techniques, it is important that they are applied to a real-world case study. In this paper, the PMI algorithm and the SOM-GAGRNN are used to find suitable inputs to an ANN model for forecasting salinity in the River Murray at Murray Bridge, South Australia. The proposed methods are also compared with two methods used in previous studies, for the same case study. The two proposed methods were found to lead to more parsimonious models with a lower forecasting error than the models developed using the methods from previous studies. To verify the robustness of each of the ANNs developed using the proposed methodology, a real-time forecasting simulation was conducted. This validation data set consisted of independent data from a six-year period from 1992 to 1998. The ANN developed using the inputs identified by the stepwise PMI algorithm was found to be the most robust for this validation set. The PMI scores obtained using the stepwise PMI algorithm revealed useful information about the order of importance of each significant input.
ناشر
Database: Elsevier - ScienceDirect (ساینس دایرکت)
Journal: Journal of Hydrology - Volume 301, Issues 1â4, 20 January 2005, Pages 93-107
Journal: Journal of Hydrology - Volume 301, Issues 1â4, 20 January 2005, Pages 93-107
نویسندگان
Gavin J. Bowden, Holger R. Maier, Graeme C. Dandy,