کد مقاله | کد نشریه | سال انتشار | مقاله انگلیسی | نسخه تمام متن |
---|---|---|---|---|
6431594 | 1635376 | 2016 | 11 صفحه PDF | دانلود رایگان |
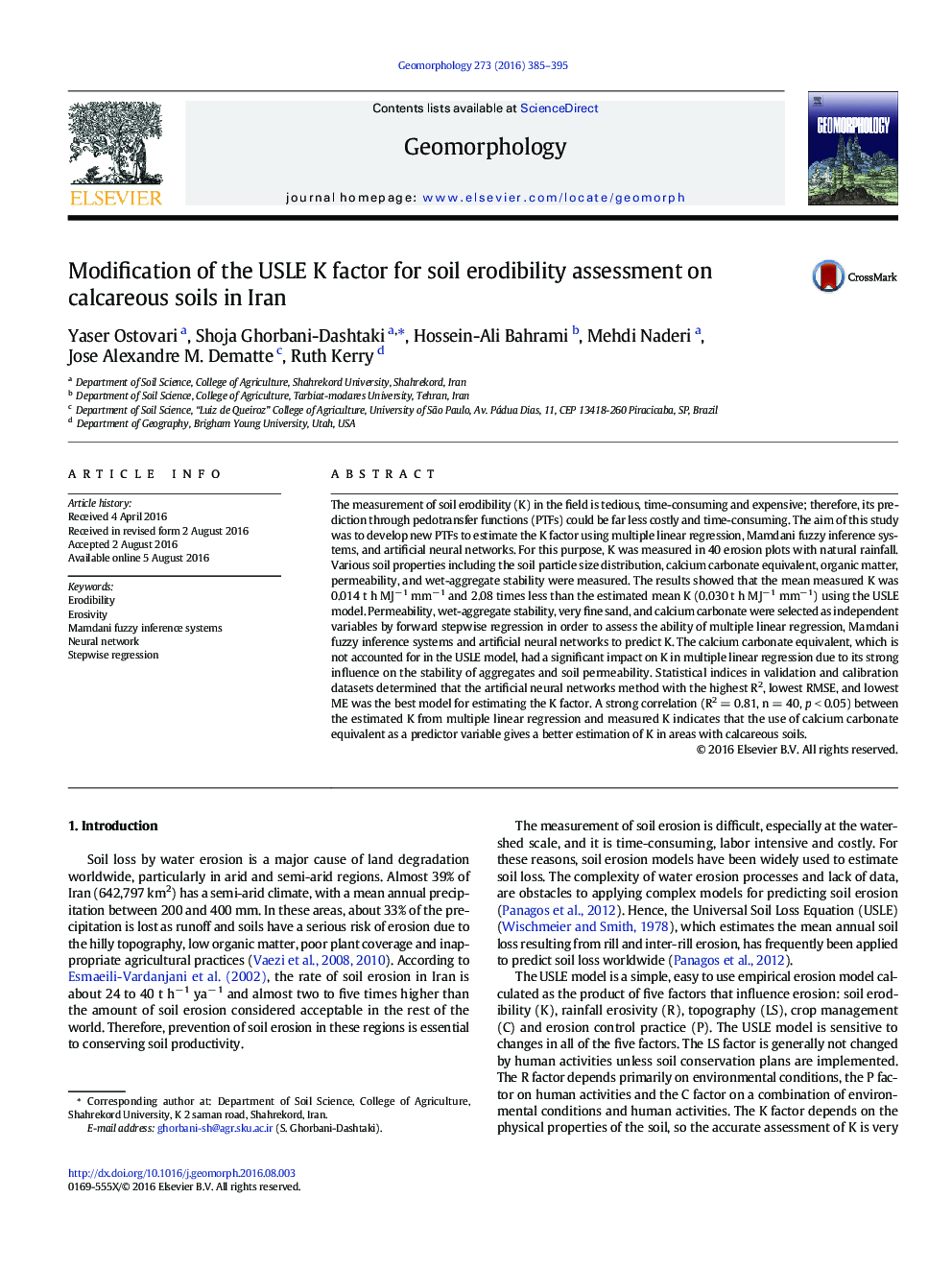
- Measuring the soil erodibility (K) in field using standard plot
- Developing new PTFs to predict K for calcareous soils
- Using fuzzy inference system and artificial neural network to predict K
- Comparing newly PTFs with the USLE equation to predict K
The measurement of soil erodibility (K) in the field is tedious, time-consuming and expensive; therefore, its prediction through pedotransfer functions (PTFs) could be far less costly and time-consuming. The aim of this study was to develop new PTFs to estimate the K factor using multiple linear regression, Mamdani fuzzy inference systems, and artificial neural networks. For this purpose, K was measured in 40 erosion plots with natural rainfall. Various soil properties including the soil particle size distribution, calcium carbonate equivalent, organic matter, permeability, and wet-aggregate stability were measured. The results showed that the mean measured K was 0.014 t h MJâ 1 mmâ 1 and 2.08 times less than the estimated mean K (0.030 t h MJâ 1 mmâ 1) using the USLE model. Permeability, wet-aggregate stability, very fine sand, and calcium carbonate were selected as independent variables by forward stepwise regression in order to assess the ability of multiple linear regression, Mamdani fuzzy inference systems and artificial neural networks to predict K. The calcium carbonate equivalent, which is not accounted for in the USLE model, had a significant impact on K in multiple linear regression due to its strong influence on the stability of aggregates and soil permeability. Statistical indices in validation and calibration datasets determined that the artificial neural networks method with the highest R2, lowest RMSE, and lowest ME was the best model for estimating the K factor. A strong correlation (R2 = 0.81, n = 40, p < 0.05) between the estimated K from multiple linear regression and measured K indicates that the use of calcium carbonate equivalent as a predictor variable gives a better estimation of K in areas with calcareous soils.
Journal: Geomorphology - Volume 273, 15 November 2016, Pages 385-395